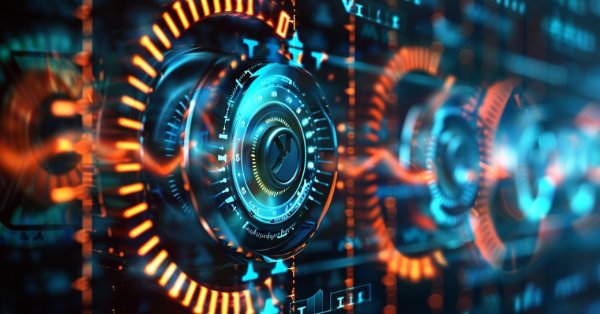
Сode Lock Algorithm (CLA)
In this article, we will rethink code locks, transforming them from security mechanisms into tools for solving complex optimization problems. Discover the world of code locks viewed not as simple security devices, but as inspiration for a new approach to optimization. We will create a whole population of "locks", where each lock represents a unique solution to the problem. We will then develop an algorithm that will "pick" these locks and find optimal solutions in a variety of areas, from machine learning to trading systems development.
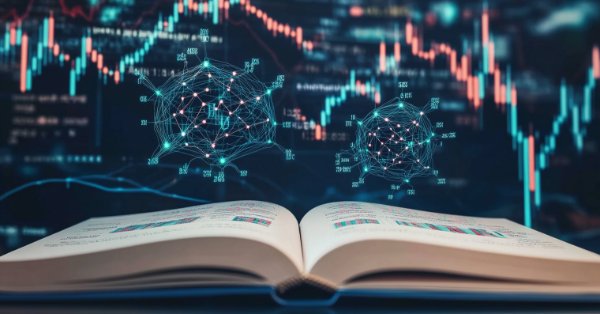
MQL5 Wizard Techniques you should know (Part 41): Deep-Q-Networks
The Deep-Q-Network is a reinforcement learning algorithm that engages neural networks in projecting the next Q-value and ideal action during the training process of a machine learning module. We have already considered an alternative reinforcement learning algorithm, Q-Learning. This article therefore presents another example of how an MLP trained with reinforcement learning, can be used within a custom signal class.
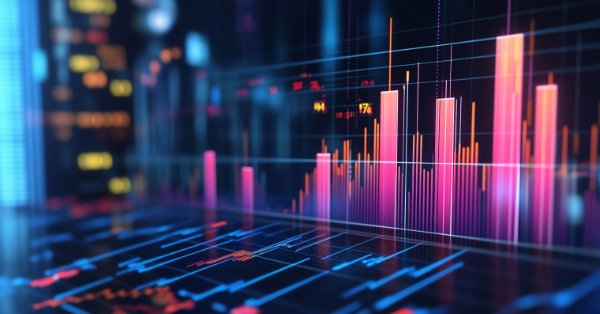
Gain An Edge Over Any Market (Part V): FRED EURUSD Alternative Data
In today’s discussion, we used alternative Daily data from the St. Louis Federal Reserve on the Broad US-Dollar Index and a collection of other macroeconomic indicators to predict the EURUSD future exchange rate. Unfortunately, while the data appears to have almost perfect correlation, we failed to realize any material gains in our model accuracy, possibly suggesting to us that investors may be better off using ordinary market quotes instead.
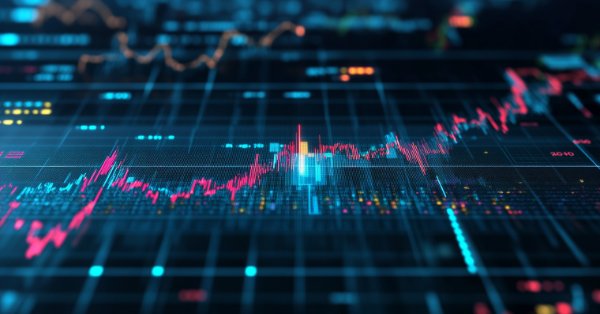
Data Science and ML(Part 30): The Power Couple for Predicting the Stock Market, Convolutional Neural Networks(CNNs) and Recurrent Neural Networks(RNNs)
In this article, We explore the dynamic integration of Convolutional Neural Networks (CNNs) and Recurrent Neural Networks (RNNs) in stock market prediction. By leveraging CNNs' ability to extract patterns and RNNs' proficiency in handling sequential data. Let us see how this powerful combination can enhance the accuracy and efficiency of trading algorithms.
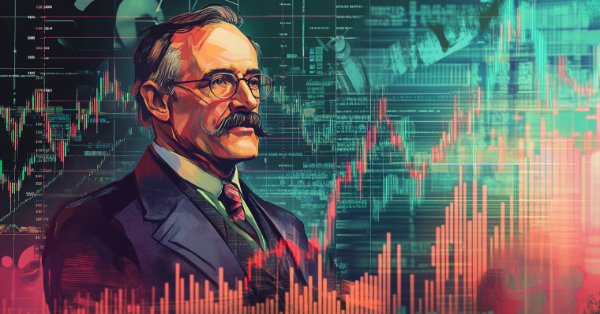
Building A Candlestick Trend Constraint Model (Part 9): Multiple Strategies Expert Advisor (I)
Today, we will explore the possibilities of incorporating multiple strategies into an Expert Advisor (EA) using MQL5. Expert Advisors provide broader capabilities than just indicators and scripts, allowing for more sophisticated trading approaches that can adapt to changing market conditions. Find, more in this article discussion.
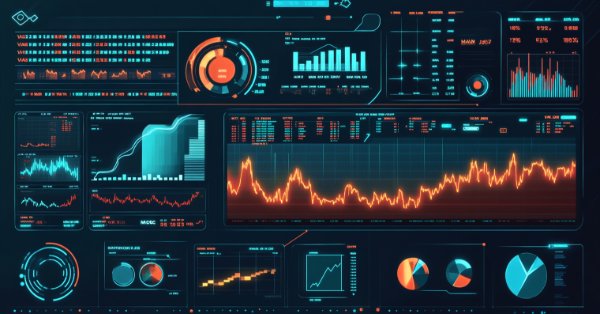
Example of new Indicator and Conditional LSTM
This article explores the development of an Expert Advisor (EA) for automated trading that combines technical analysis with deep learning predictions.
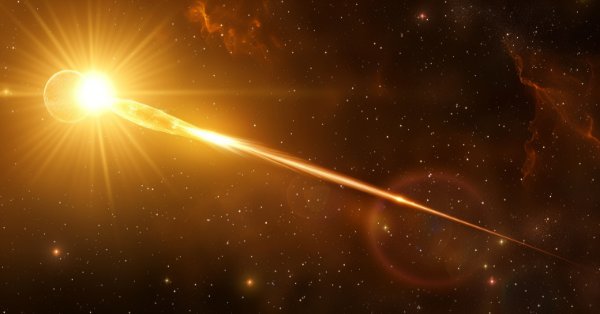
Comet Tail Algorithm (CTA)
In this article, we will look at the Comet Tail Optimization Algorithm (CTA), which draws inspiration from unique space objects - comets and their impressive tails that form when approaching the Sun. The algorithm is based on the concept of the motion of comets and their tails, and is designed to find optimal solutions in optimization problems.
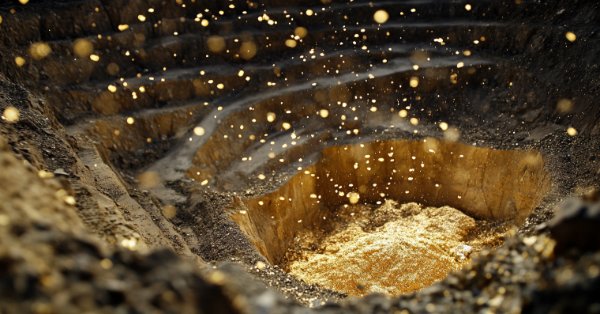
Gain An Edge Over Any Market (Part IV): CBOE Euro And Gold Volatility Indexes
We will analyze alternative data curated by the Chicago Board Of Options Exchange (CBOE) to improve the accuracy of our deep neural networks when forecasting the XAUEUR symbol.
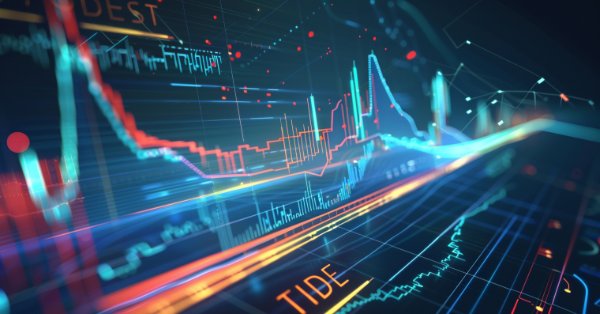
Neural Networks Made Easy (Part 88): Time-Series Dense Encoder (TiDE)
In an attempt to obtain the most accurate forecasts, researchers often complicate forecasting models. Which in turn leads to increased model training and maintenance costs. Is such an increase always justified? This article introduces an algorithm that uses the simplicity and speed of linear models and demonstrates results on par with the best models with a more complex architecture.
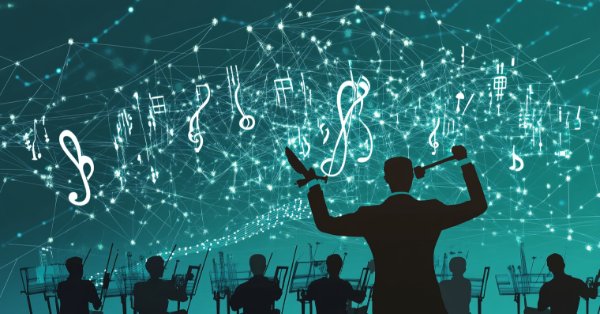
Self Optimizing Expert Advisor With MQL5 And Python (Part IV): Stacking Models
Today, we will demonstrate how you can build AI-powered trading applications capable of learning from their own mistakes. We will demonstrate a technique known as stacking, whereby we use 2 models to make 1 prediction. The first model is typically a weaker learner, and the second model is typically a more powerful model that learns the residuals of our weaker learner. Our goal is to create an ensemble of models, to hopefully attain higher accuracy.
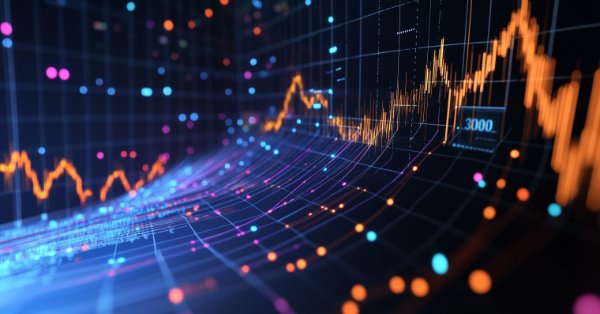
Using PSAR, Heiken Ashi, and Deep Learning Together for Trading
This project explores the fusion of deep learning and technical analysis to test trading strategies in forex. A Python script is used for rapid experimentation, employing an ONNX model alongside traditional indicators like PSAR, SMA, and RSI to predict EUR/USD movements. A MetaTrader 5 script then brings this strategy into a live environment, using historical data and technical analysis to make informed trading decisions. The backtesting results indicate a cautious yet consistent approach, with a focus on risk management and steady growth rather than aggressive profit-seeking.
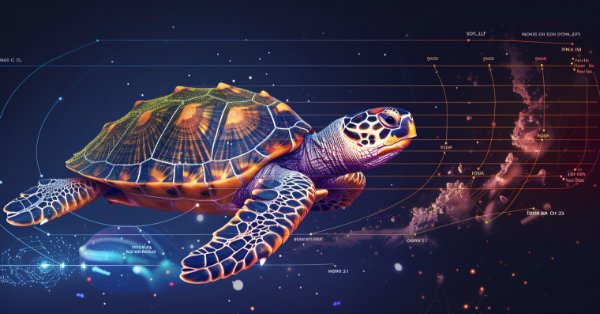
Turtle Shell Evolution Algorithm (TSEA)
This is a unique optimization algorithm inspired by the evolution of the turtle shell. The TSEA algorithm emulates the gradual formation of keratinized skin areas, which represent optimal solutions to a problem. The best solutions become "harder" and are located closer to the outer surface, while the less successful solutions remain "softer" and are located inside. The algorithm uses clustering of solutions by quality and distance, allowing to preserve less successful options and providing flexibility and adaptability.
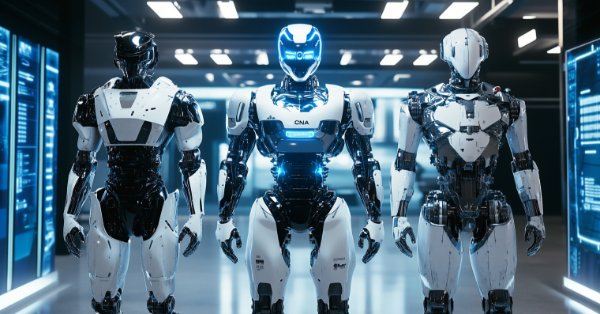
Example of CNA (Causality Network Analysis), SMOC (Stochastic Model Optimal Control) and Nash Game Theory with Deep Learning
We will add Deep Learning to those three examples that were published in previous articles and compare results with previous. The aim is to learn how to add DL to other EA.
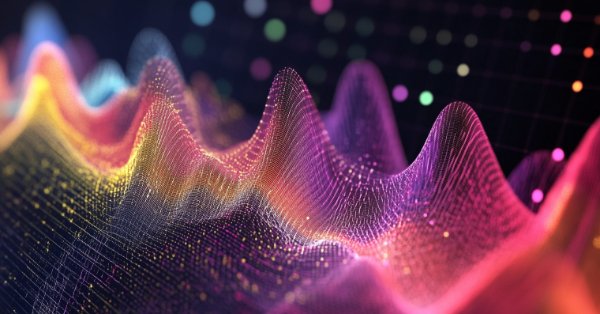
Applying Localized Feature Selection in Python and MQL5
This article explores a feature selection algorithm introduced in the paper 'Local Feature Selection for Data Classification' by Narges Armanfard et al. The algorithm is implemented in Python to build binary classifier models that can be integrated with MetaTrader 5 applications for inference.
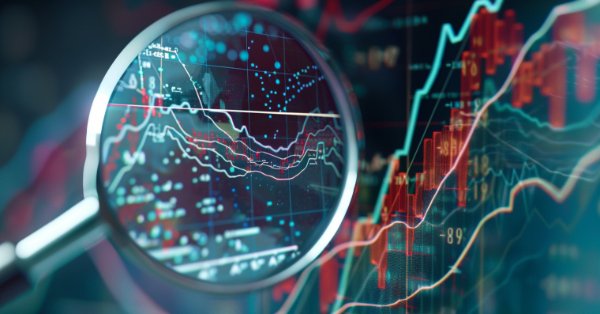
Neural Networks Made Easy (Part 87): Time Series Patching
Forecasting plays an important role in time series analysis. In the new article, we will talk about the benefits of time series patching.
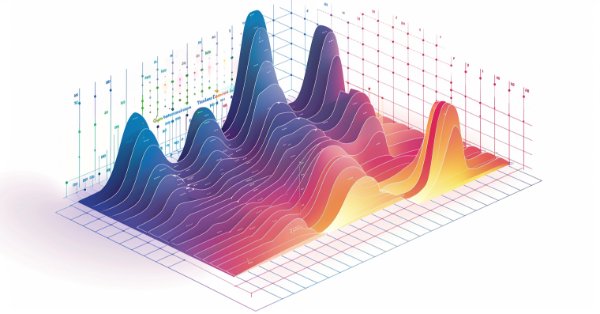
Neural Networks Made Easy (Part 86): U-Shaped Transformer
We continue to study timeseries forecasting algorithms. In this article, we will discuss another method: the U-shaped Transformer.
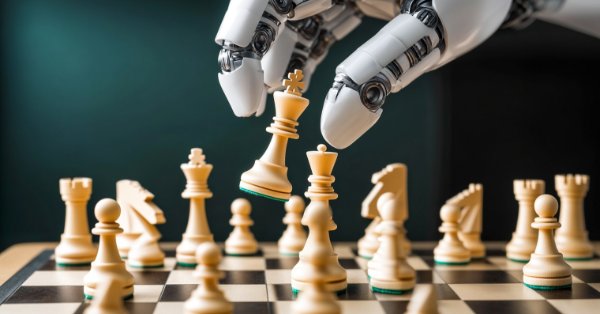
MQL5 Wizard Techniques you should know (Part 36): Q-Learning with Markov Chains
Reinforcement Learning is one of the three main tenets in machine learning, alongside supervised learning and unsupervised learning. It is therefore concerned with optimal control, or learning the best long-term policy that will best suit the objective function. It is with this back-drop, that we explore its possible role in informing the learning-process to an MLP of a wizard assembled Expert Advisor.
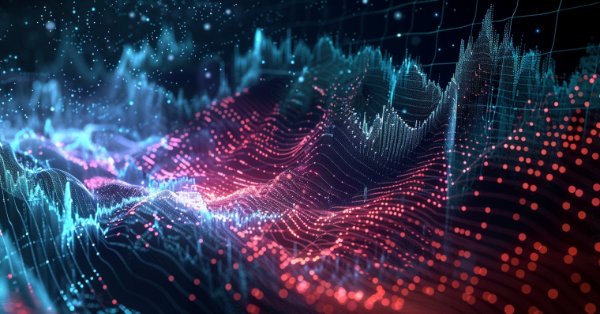
Neural Networks Made Easy (Part 85): Multivariate Time Series Forecasting
In this article, I would like to introduce you to a new complex timeseries forecasting method, which harmoniously combines the advantages of linear models and transformers.
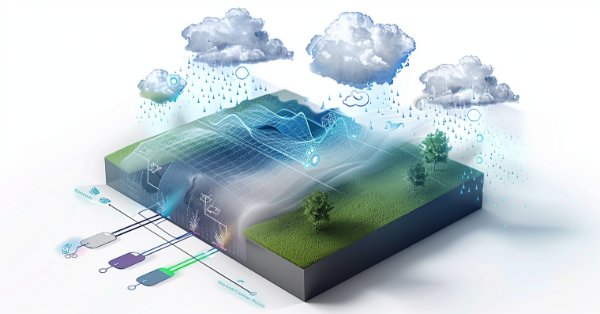
Neural Networks Made Easy (Part 84): Reversible Normalization (RevIN)
We already know that pre-processing of the input data plays a major role in the stability of model training. To process "raw" input data online, we often use a batch normalization layer. But sometimes we need a reverse procedure. In this article, we discuss one of the possible approaches to solving this problem.
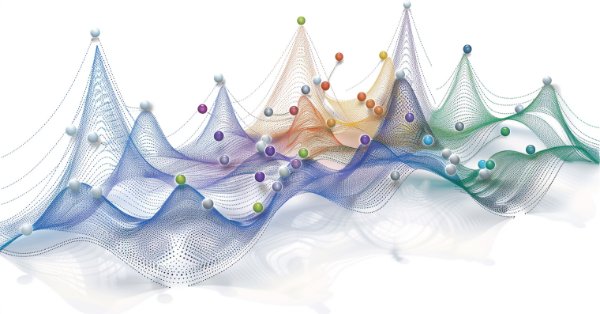
Brain Storm Optimization algorithm (Part II): Multimodality
In the second part of the article, we will move on to the practical implementation of the BSO algorithm, conduct tests on test functions and compare the efficiency of BSO with other optimization methods.
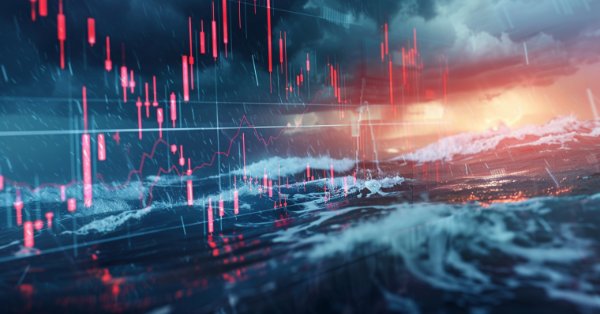
Neural Networks Made Easy (Part 83): The "Conformer" Spatio-Temporal Continuous Attention Transformer Algorithm
This article introduces the Conformer algorithm originally developed for the purpose of weather forecasting, which in terms of variability and capriciousness can be compared to financial markets. Conformer is a complex method. It combines the advantages of attention models and ordinary differential equations.
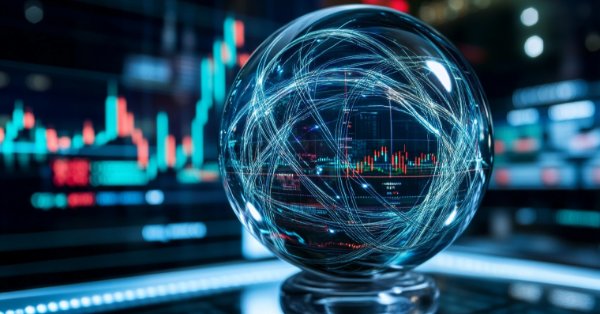
Example of Causality Network Analysis (CNA) and Vector Auto-Regression Model for Market Event Prediction
This article presents a comprehensive guide to implementing a sophisticated trading system using Causality Network Analysis (CNA) and Vector Autoregression (VAR) in MQL5. It covers the theoretical background of these methods, provides detailed explanations of key functions in the trading algorithm, and includes example code for implementation.
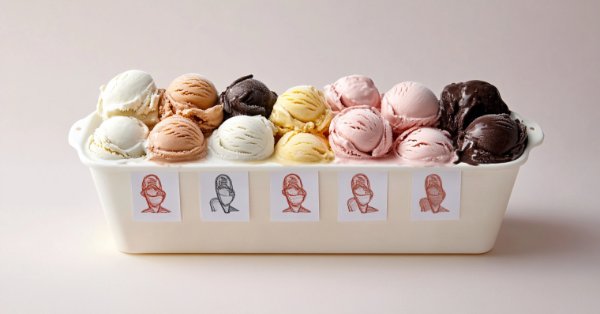
MQL5 Wizard Techniques you should know (Part 35): Support Vector Regression
Support Vector Regression is an idealistic way of finding a function or ‘hyper-plane’ that best describes the relationship between two sets of data. We attempt to exploit this in time series forecasting within custom classes of the MQL5 wizard.
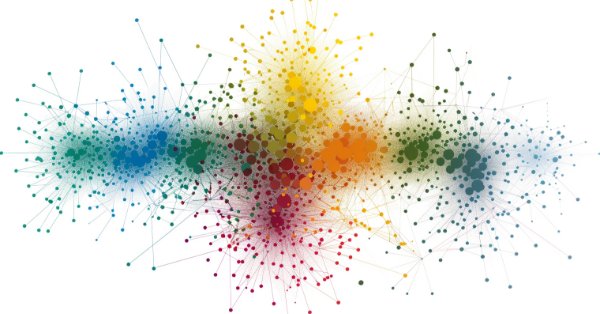
Brain Storm Optimization algorithm (Part I): Clustering
In this article, we will look at an innovative optimization method called BSO (Brain Storm Optimization) inspired by a natural phenomenon called "brainstorming". We will also discuss a new approach to solving multimodal optimization problems the BSO method applies. It allows finding multiple optimal solutions without the need to pre-determine the number of subpopulations. We will also consider the K-Means and K-Means++ clustering methods.
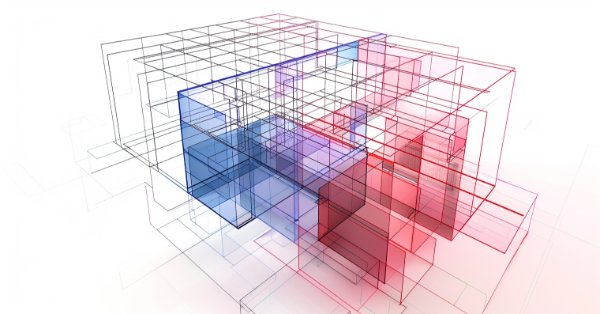
Matrix Factorization: The Basics
Since the goal here is didactic, we will proceed as simply as possible. That is, we will implement only what we need: matrix multiplication. You will see today that this is enough to simulate matrix-scalar multiplication. The most significant difficulty that many people encounter when implementing code using matrix factorization is this: unlike scalar factorization, where in almost all cases the order of the factors does not change the result, this is not the case when using matrices.
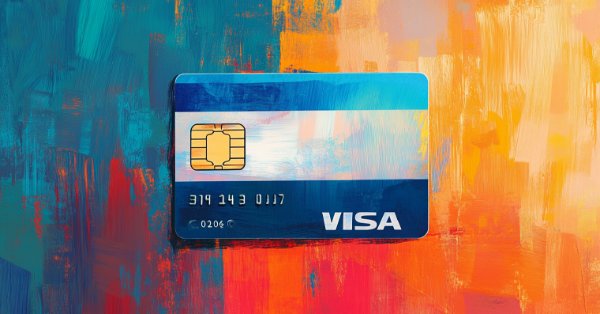
Gain an Edge Over Any Market (Part III): Visa Spending Index
In the world of big data, there are millions of alternative datasets that hold the potential to enhance our trading strategies. In this series of articles, we will help you identify the most informative public datasets.
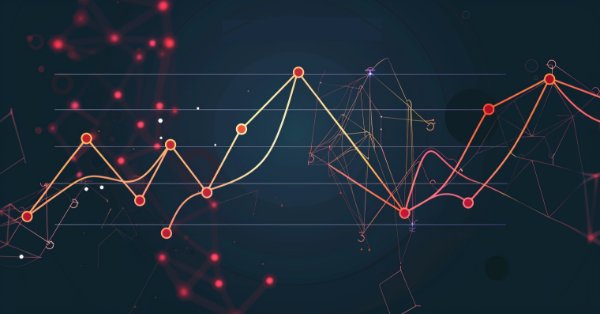
Neural Network in Practice: Secant Line
As already explained in the theoretical part, when working with neural networks we need to use linear regressions and derivatives. Why? The reason is that linear regression is one of the simplest formulas in existence. Essentially, linear regression is just an affine function. However, when we talk about neural networks, we are not interested in the effects of direct linear regression. We are interested in the equation that generates this line. We are not that interested in the line created. Do you know the main equation that we need to understand? If not, I recommend reading this article to understanding it.
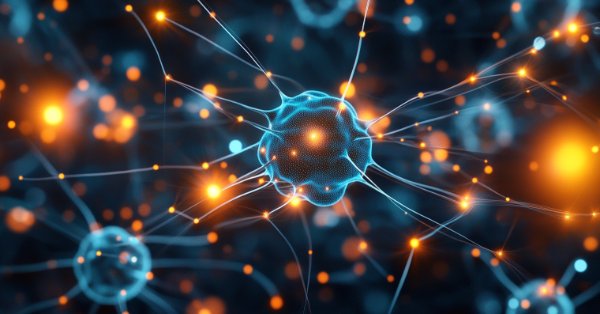
MQL5 Wizard Techniques you should know (Part 34): Price-Embedding with an Unconventional RBM
Restricted Boltzmann Machines are a form of neural network that was developed in the mid 1980s at a time when compute resources were prohibitively expensive. At its onset, it relied on Gibbs Sampling and Contrastive Divergence in order to reduce dimensionality or capture the hidden probabilities/properties over input training data sets. We examine how Backpropagation can perform similarly when the RBM ‘embeds’ prices for a forecasting Multi-Layer-Perceptron.
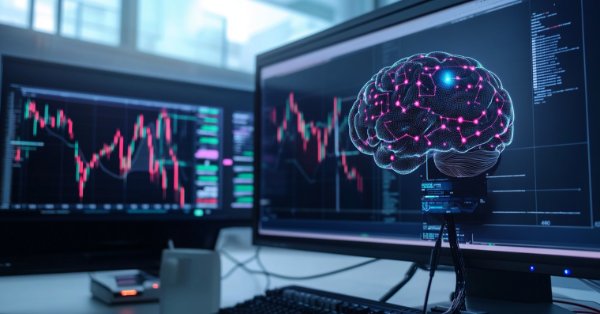
Reimagining Classic Strategies (Part VI): Multiple Time-Frame Analysis
In this series of articles, we revisit classic strategies to see if we can improve them using AI. In today's article, we will examine the popular strategy of multiple time-frame analysis to judge if the strategy would be enhanced with AI.
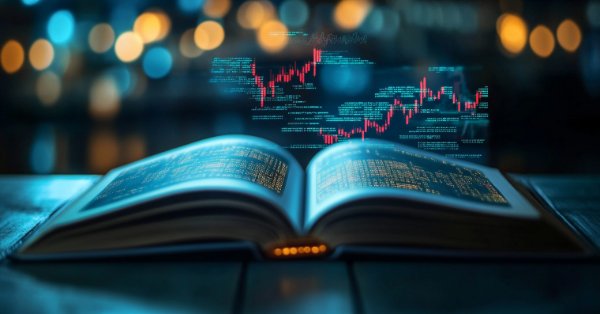
Integrating MQL5 with data processing packages (Part 2): Machine Learning and Predictive Analytics
In our series on integrating MQL5 with data processing packages, we delve in to the powerful combination of machine learning and predictive analysis. We will explore how to seamlessly connect MQL5 with popular machine learning libraries, to enable sophisticated predictive models for financial markets.
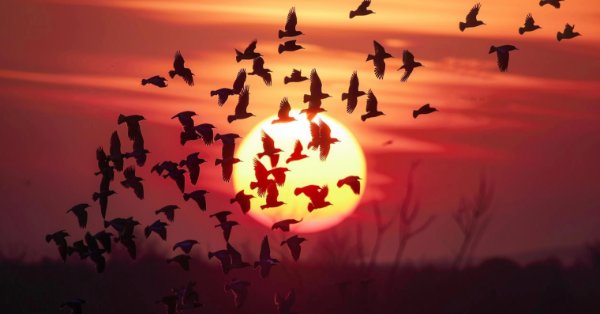
Population optimization algorithms: Bird Swarm Algorithm (BSA)
The article explores the bird swarm-based algorithm (BSA) inspired by the collective flocking interactions of birds in nature. The different search strategies of individuals in BSA, including switching between flight, vigilance and foraging behavior, make this algorithm multifaceted. It uses the principles of bird flocking, communication, adaptability, leading and following to efficiently find optimal solutions.
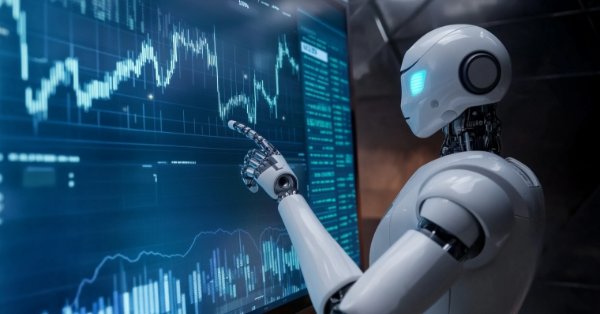
Reimagining Classic Strategies (Part V): Multiple Symbol Analysis on USDZAR
In this series of articles, we revisit classical strategies to see if we can improve the strategy using AI. In today's article, we will examine a popular strategy of multiple symbol analysis using a basket of correlated securities, we will focus on the exotic USDZAR currency pair.
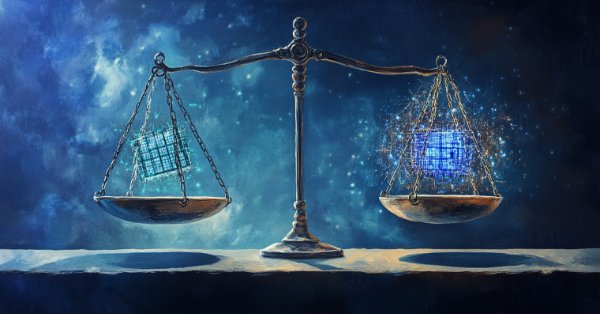
MQL5 Wizard Techniques you should know (Part 32): Regularization
Regularization is a form of penalizing the loss function in proportion to the discrete weighting applied throughout the various layers of a neural network. We look at the significance, for some of the various regularization forms, this can have in test runs with a wizard assembled Expert Advisor.
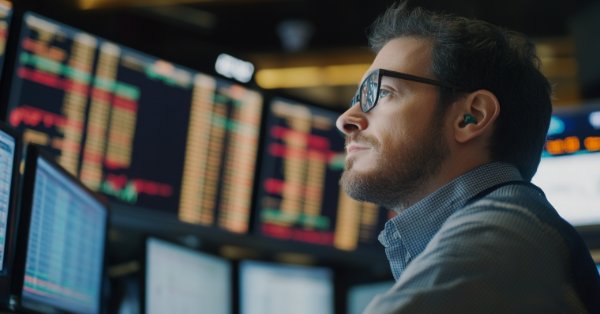
Reimagining Classic Strategies (Part IV): SP500 and US Treasury Notes
In this series of articles, we analyze classical trading strategies using modern algorithms to determine whether we can improve the strategy using AI. In today's article, we revisit a classical approach for trading the SP500 using the relationship it has with US Treasury Notes.
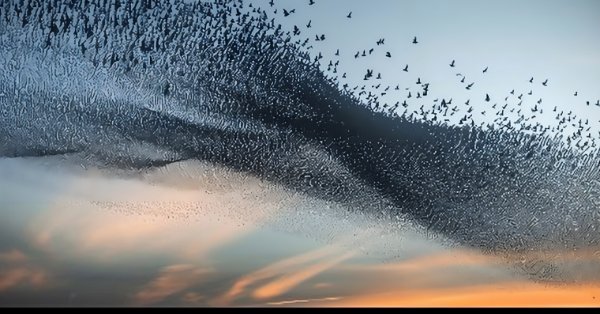
Population optimization algorithms: Boids Algorithm
The article considers Boids algorithm based on unique examples of animal flocking behavior. In turn, the Boids algorithm serves as the basis for the creation of the whole class of algorithms united under the name "Swarm Intelligence".
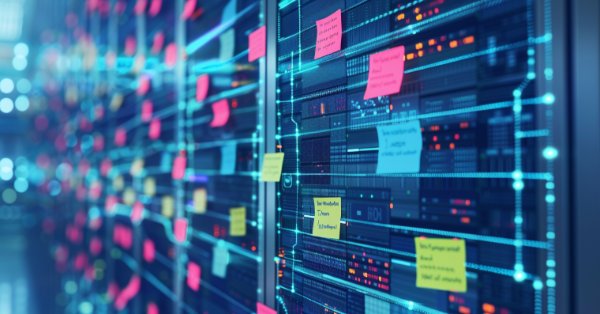
Developing a robot in Python and MQL5 (Part 1): Data preprocessing
Developing a trading robot based on machine learning: A detailed guide. The first article in the series deals with collecting and preparing data and features. The project is implemented using the Python programming language and libraries, as well as the MetaTrader 5 platform.
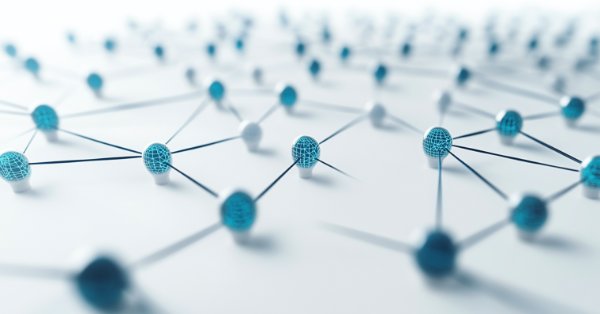
Example of Auto Optimized Take Profits and Indicator Parameters with SMA and EMA
This article presents a sophisticated Expert Advisor for forex trading, combining machine learning with technical analysis. It focuses on trading Apple stock, featuring adaptive optimization, risk management, and multiple strategies. Backtesting shows promising results with high profitability but also significant drawdowns, indicating potential for further refinement.
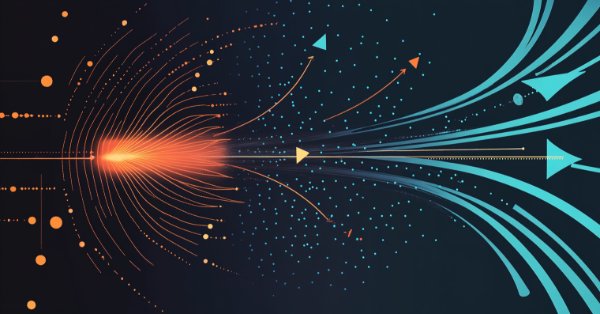
MQL5 Wizard Techniques you should know (Part 31): Selecting the Loss Function
Loss Function is the key metric of machine learning algorithms that provides feedback to the training process by quantifying how well a given set of parameters are performing when compared to their intended target. We explore the various formats of this function in an MQL5 custom wizard class.
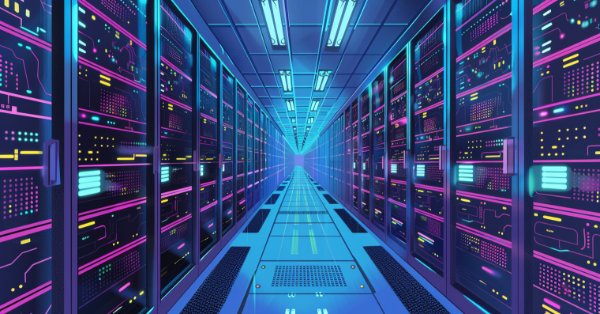
Integrate Your Own LLM into EA (Part 5): Develop and Test Trading Strategy with LLMs(I)-Fine-tuning
With the rapid development of artificial intelligence today, language models (LLMs) are an important part of artificial intelligence, so we should think about how to integrate powerful LLMs into our algorithmic trading. For most people, it is difficult to fine-tune these powerful models according to their needs, deploy them locally, and then apply them to algorithmic trading. This series of articles will take a step-by-step approach to achieve this goal.
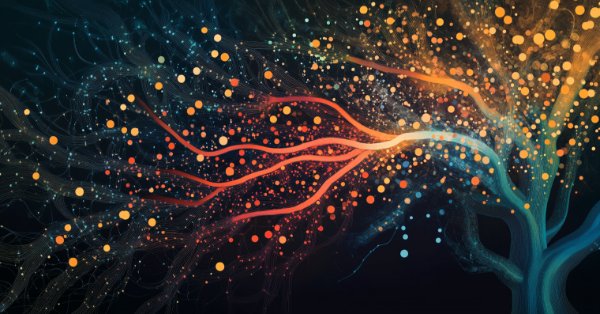
Data Science and ML (Part 29): Essential Tips for Selecting the Best Forex Data for AI Training Purposes
In this article, we dive deep into the crucial aspects of choosing the most relevant and high-quality Forex data to enhance the performance of AI models.