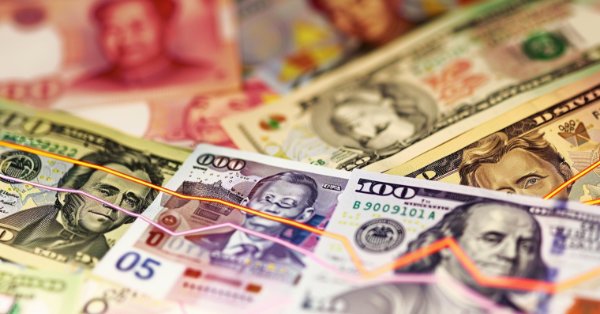
MQL5 Wizard Techniques you should know (Part 17): Multicurrency Trading
Trading across multiple currencies is not available by default when an expert advisor is assembled via the wizard. We examine 2 possible hacks traders can make when looking to test their ideas off more than one symbol at a time.
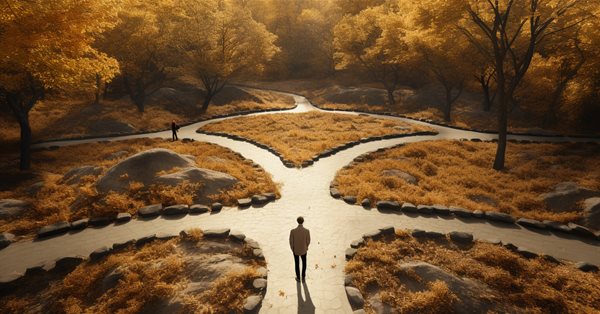
Neural networks made easy (Part 68): Offline Preference-guided Policy Optimization
Since the first articles devoted to reinforcement learning, we have in one way or another touched upon 2 problems: exploring the environment and determining the reward function. Recent articles have been devoted to the problem of exploration in offline learning. In this article, I would like to introduce you to an algorithm whose authors completely eliminated the reward function.
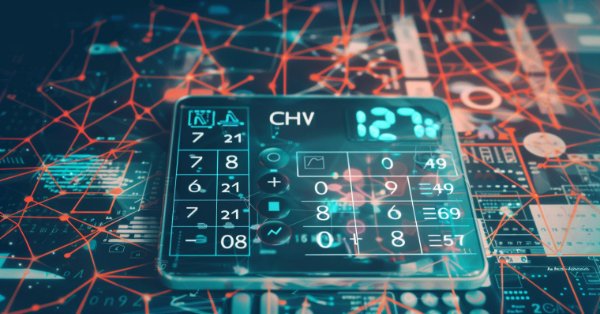
How to build and optimize a volatility-based trading system (Chaikin Volatility - CHV)
In this article, we will provide another volatility-based indicator named Chaikin Volatility. We will understand how to build a custom indicator after identifying how it can be used and constructed. We will share some simple strategies that can be used and then test them to understand which one can be better.
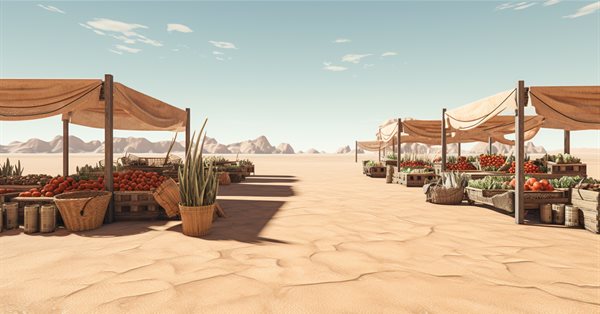
Creating a market making algorithm in MQL5
How do market makers work? Let's consider this issue and create a primitive market-making algorithm.
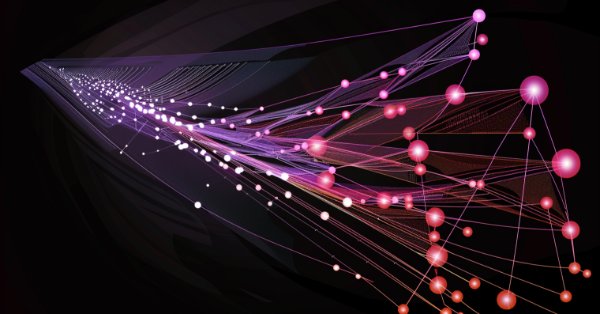
MQL5 Wizard Techniques you should know (Part 16): Principal Component Analysis with Eigen Vectors
Principal Component Analysis, a dimensionality reducing technique in data analysis, is looked at in this article, with how it could be implemented with Eigen values and vectors. As always, we aim to develop a prototype expert-signal-class usable in the MQL5 wizard.
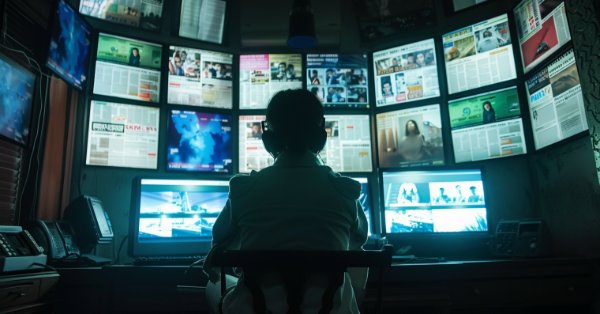
News Trading Made Easy (Part 1): Creating a Database
News trading can be complicated and overwhelming, in this article we will go through steps to obtain news data. Additionally we will learn about the MQL5 Economic Calendar and what it has to offer.
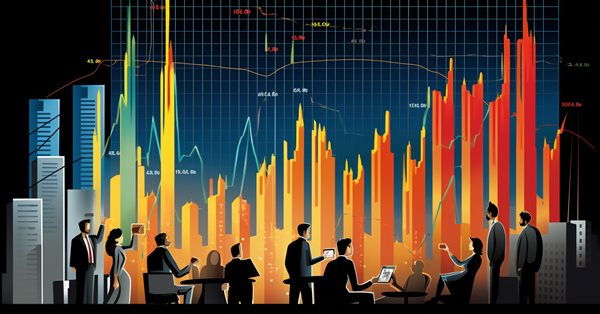
Quantitative analysis in MQL5: Implementing a promising algorithm
We will analyze the question of what quantitative analysis is and how it is used by major players. We will create one of the quantitative analysis algorithms in the MQL5 language.
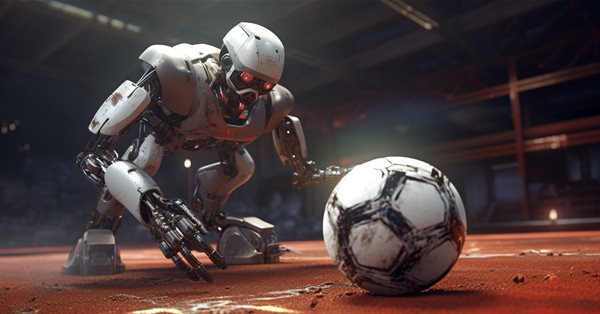
Neural networks made easy (Part 67): Using past experience to solve new tasks
In this article, we continue discussing methods for collecting data into a training set. Obviously, the learning process requires constant interaction with the environment. However, situations can be different.
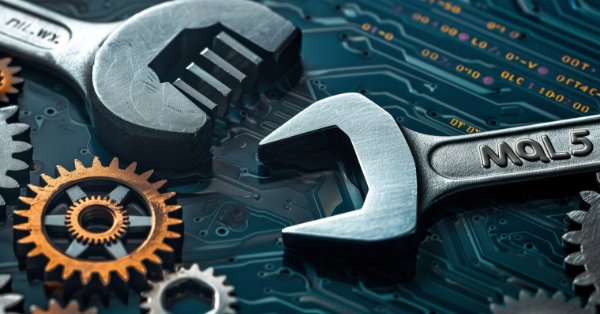
Build Self Optmising Expert Advisors in MQL5
Build expert advisors that look forward and adjust themselves to any market.
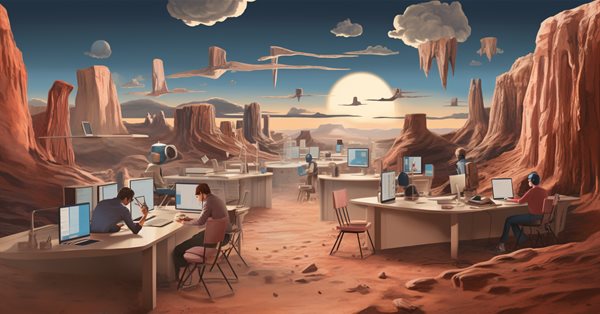
Neural networks made easy (Part 66): Exploration problems in offline learning
Models are trained offline using data from a prepared training dataset. While providing certain advantages, its negative side is that information about the environment is greatly compressed to the size of the training dataset. Which, in turn, limits the possibilities of exploration. In this article, we will consider a method that enables the filling of a training dataset with the most diverse data possible.
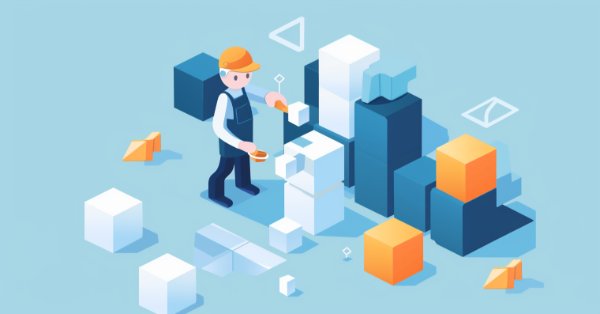
Introduction to MQL5 (Part 6): A Beginner's Guide to Array Functions in MQL5
Embark on the next phase of our MQL5 journey. In this insightful and beginner-friendly article, we'll look into the remaining array functions, demystifying complex concepts to empower you to craft efficient trading strategies. We’ll be discussing ArrayPrint, ArrayInsert, ArraySize, ArrayRange, ArrarRemove, ArraySwap, ArrayReverse, and ArraySort. Elevate your algorithmic trading expertise with these essential array functions. Join us on the path to MQL5 mastery!
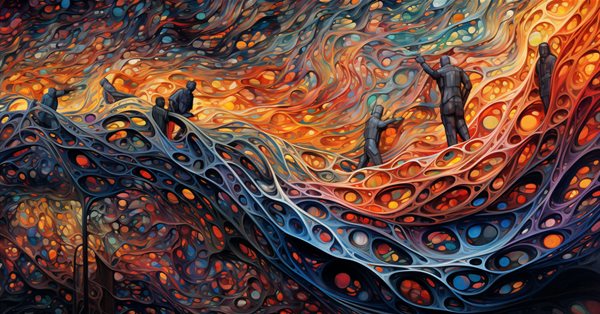
Neural networks made easy (Part 65): Distance Weighted Supervised Learning (DWSL)
In this article, we will get acquainted with an interesting algorithm that is built at the intersection of supervised and reinforcement learning methods.
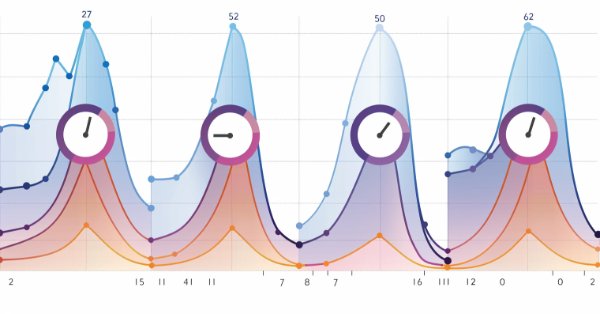
Seasonality Filtering and time period for Deep Learning ONNX models with python for EA
Can we benefit from seasonality when creating models for Deep Learning with python? Does filtering data for the ONNX models help to get better results? What time period should we use? We will cover all of this over this article.
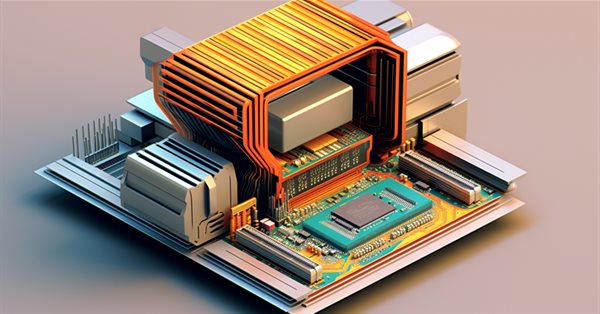
Master MQL5 from beginner to pro (Part I): Getting started with programming
This article is an introduction to a series of articles about programming. It is assumed here that the reader has never dealt with programming before. So, this series starts from the very basics. Programming knowledge level: Absolute Beginner.
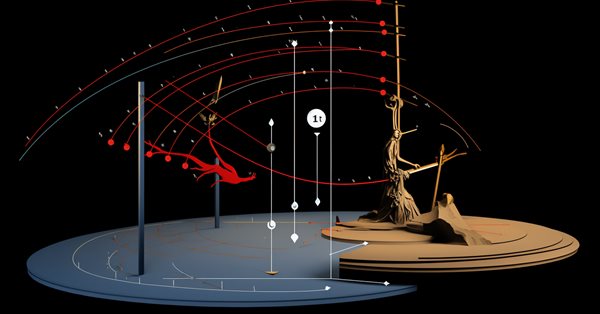
Neural networks made easy (Part 64): ConserWeightive Behavioral Cloning (CWBC) method
As a result of tests performed in previous articles, we came to the conclusion that the optimality of the trained strategy largely depends on the training set used. In this article, we will get acquainted with a fairly simple yet effective method for selecting trajectories to train models.
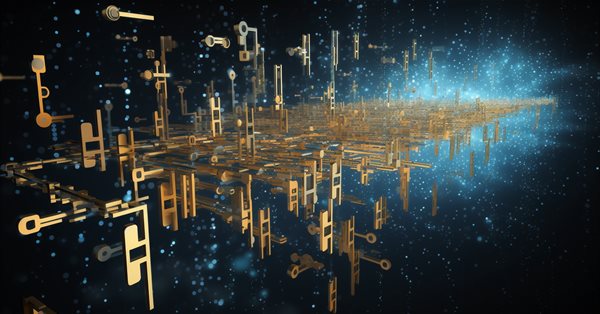
Neural networks made easy (Part 63): Unsupervised Pretraining for Decision Transformer (PDT)
We continue to discuss the family of Decision Transformer methods. From previous article, we have already noticed that training the transformer underlying the architecture of these methods is a rather complex task and requires a large labeled dataset for training. In this article we will look at an algorithm for using unlabeled trajectories for preliminary model training.
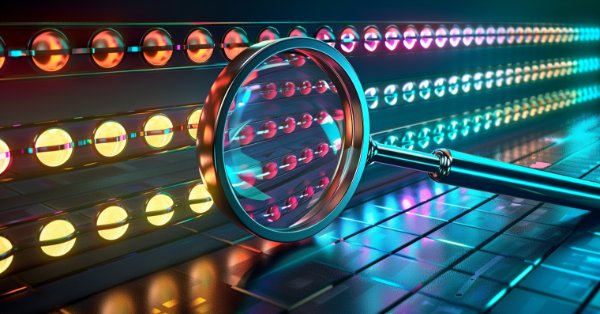
Introduction to MQL5 (Part 5): A Beginner's Guide to Array Functions in MQL5
Explore the world of MQL5 arrays in Part 5, designed for absolute beginners. Simplifying complex coding concepts, this article focuses on clarity and inclusivity. Join our community of learners, where questions are embraced, and knowledge is shared!
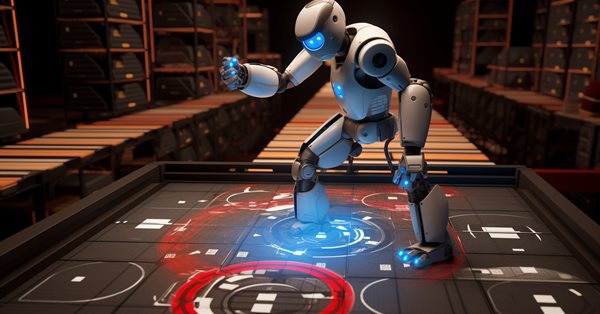
Neural networks made easy (Part 62): Using Decision Transformer in hierarchical models
In recent articles, we have seen several options for using the Decision Transformer method. The method allows analyzing not only the current state, but also the trajectory of previous states and actions performed in them. In this article, we will focus on using this method in hierarchical models.
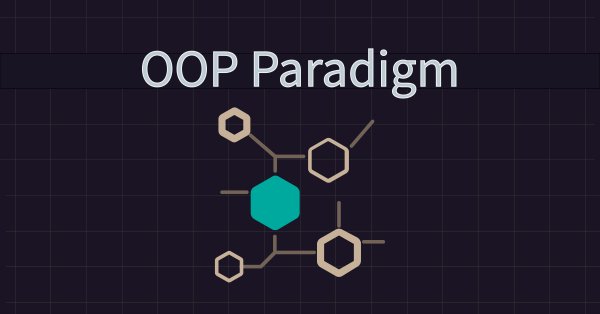
Understanding Programming Paradigms (Part 2): An Object-Oriented Approach to Developing a Price Action Expert Advisor
Learn about the object-oriented programming paradigm and its application in MQL5 code. This second article goes deeper into the specifics of object-oriented programming, offering hands-on experience through a practical example. You'll learn how to convert our earlier developed procedural price action expert advisor using the EMA indicator and candlestick price data to object-oriented code.
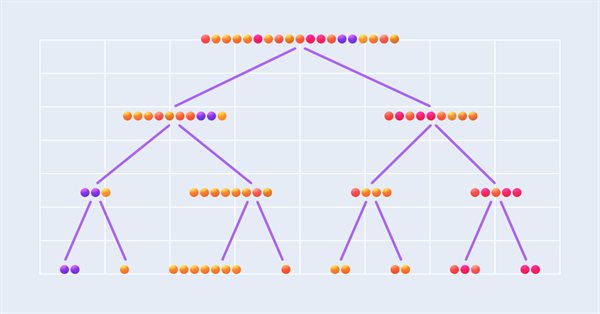
Integrating ML models with the Strategy Tester (Conclusion): Implementing a regression model for price prediction
This article describes the implementation of a regression model based on a decision tree. The model should predict prices of financial assets. We have already prepared the data, trained and evaluated the model, as well as adjusted and optimized it. However, it is important to note that this model is intended for study purposes only and should not be used in real trading.
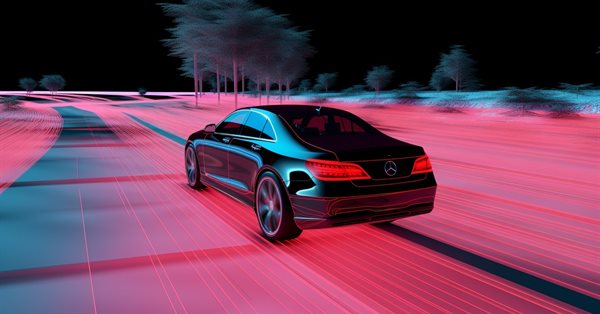
Neural networks made easy (Part 61): Optimism issue in offline reinforcement learning
During the offline learning, we optimize the Agent's policy based on the training sample data. The resulting strategy gives the Agent confidence in its actions. However, such optimism is not always justified and can cause increased risks during the model operation. Today we will look at one of the methods to reduce these risks.
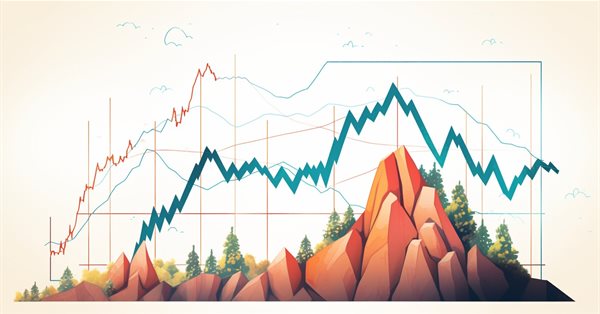
Creating multi-symbol, multi-period indicators
In this article, we will look at the principles of creating multi-symbol, multi-period indicators. We will also see how to access the data of such indicators from Expert Advisors and other indicators. We will consider the main features of using multi-indicators in Expert Advisors and indicators and will see how to plot them through custom indicator buffers.
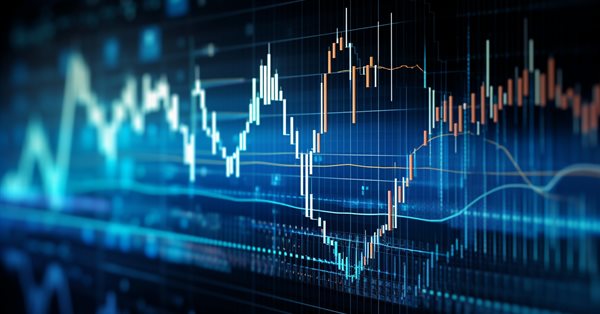
Experiments with neural networks (Part 7): Passing indicators
Examples of passing indicators to a perceptron. The article describes general concepts and showcases the simplest ready-made Expert Advisor followed by the results of its optimization and forward test.
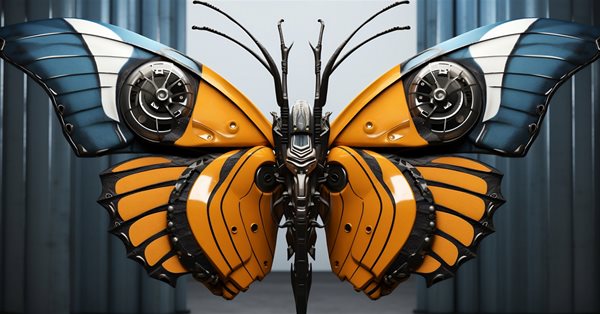
Neural networks made easy (Part 60): Online Decision Transformer (ODT)
The last two articles were devoted to the Decision Transformer method, which models action sequences in the context of an autoregressive model of desired rewards. In this article, we will look at another optimization algorithm for this method.
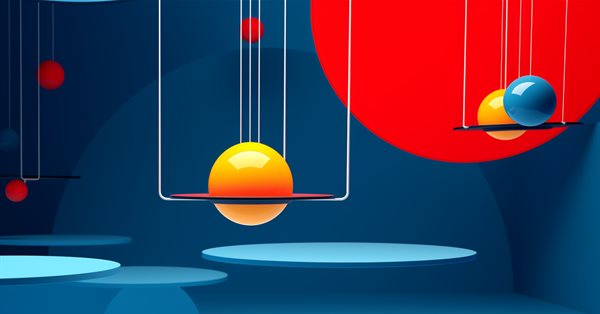
Neural networks are easy (Part 59): Dichotomy of Control (DoC)
In the previous article, we got acquainted with the Decision Transformer. But the complex stochastic environment of the foreign exchange market did not allow us to fully implement the potential of the presented method. In this article, I will introduce an algorithm that is aimed at improving the performance of algorithms in stochastic environments.
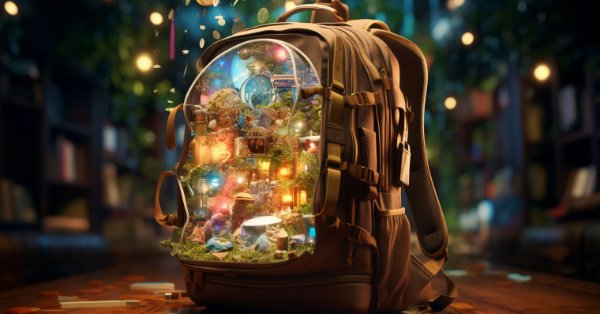
Introduction to MQL5 (Part 4): Mastering Structures, Classes, and Time Functions
Unlock the secrets of MQL5 programming in our latest article! Delve into the essentials of structures, classes, and time functions, empowering your coding journey. Whether you're a beginner or an experienced developer, our guide simplifies complex concepts, providing valuable insights for mastering MQL5. Elevate your programming skills and stay ahead in the world of algorithmic trading!
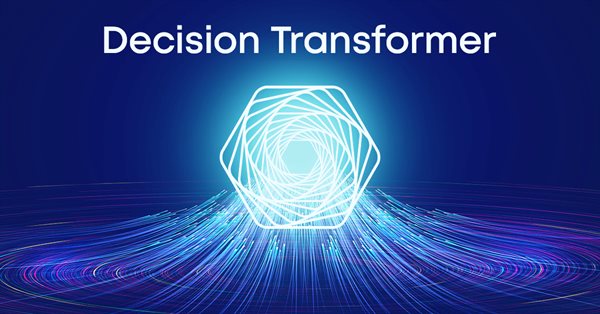
Neural networks made easy (Part 58): Decision Transformer (DT)
We continue to explore reinforcement learning methods. In this article, I will focus on a slightly different algorithm that considers the Agent’s policy in the paradigm of constructing a sequence of actions.
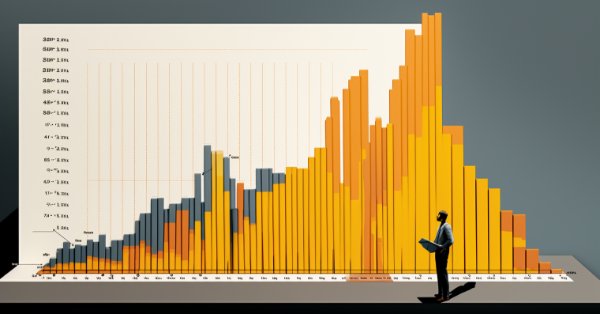
Implementing the Generalized Hurst Exponent and the Variance Ratio test in MQL5
In this article, we investigate how the Generalized Hurst Exponent and the Variance Ratio test can be utilized to analyze the behaviour of price series in MQL5.
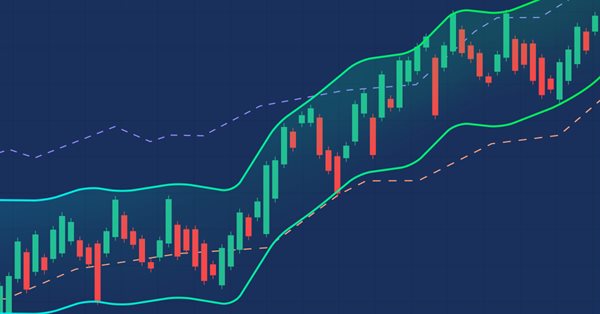
Ready-made templates for including indicators to Expert Advisors (Part 3): Trend indicators
In this reference article, we will look at standard indicators from the Trend Indicators category. We will create ready-to-use templates for indicator use in EAs - declaring and setting parameters, indicator initialization and deinitialization, as well as receiving data and signals from indicator buffers in EAs.
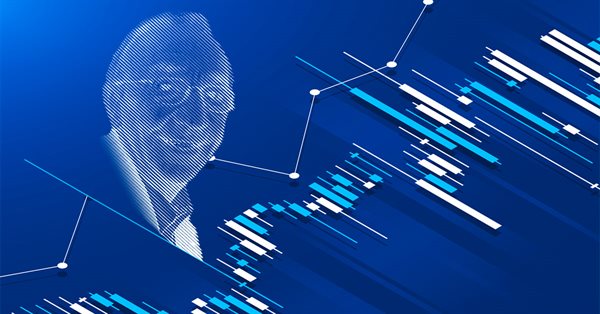
Ready-made templates for including indicators to Expert Advisors (Part 2): Volume and Bill Williams indicators
In this article, we will look at standard indicators of the Volume and Bill Williams' indicators category. We will create ready-to-use templates for indicator use in EAs - declaring and setting parameters, indicator initialization and deinitialization, as well as receiving data and signals from indicator buffers in EAs.
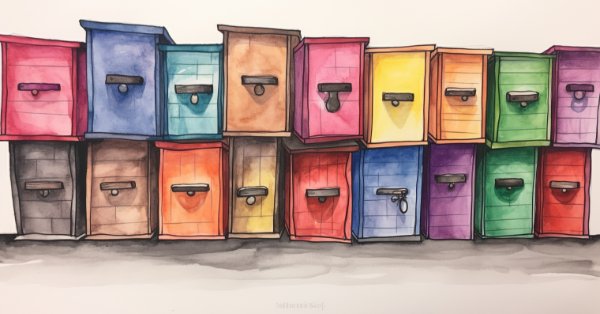
Introduction to MQL5 (Part 3): Mastering the Core Elements of MQL5
Explore the fundamentals of MQL5 programming in this beginner-friendly article, where we demystify arrays, custom functions, preprocessors, and event handling, all explained with clarity making every line of code accessible. Join us in unlocking the power of MQL5 with a unique approach that ensures understanding at every step. This article sets the foundation for mastering MQL5, emphasizing the explanation of each line of code, and providing a distinct and enriching learning experience.
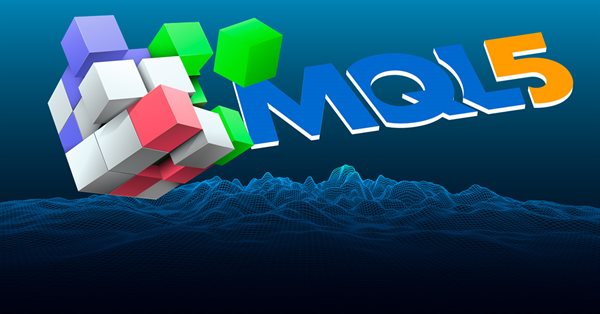
ALGLIB numerical analysis library in MQL5
The article takes a quick look at the ALGLIB 3.19 numerical analysis library, its applications and new algorithms that can improve the efficiency of financial data analysis.
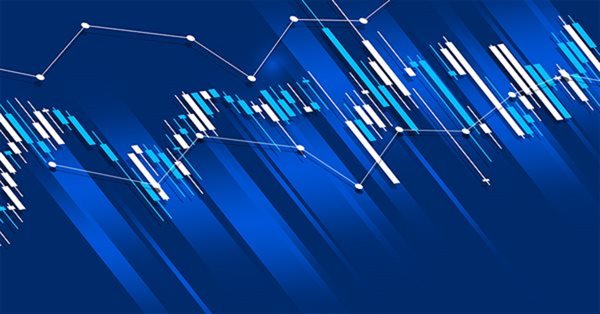
How to create a simple Multi-Currency Expert Advisor using MQL5 (Part 6): Two RSI indicators cross each other's lines
The multi-currency expert advisor in this article is an expert advisor or trading robot that uses two RSI indicators with crossing lines, the Fast RSI which crosses with the Slow RSI.
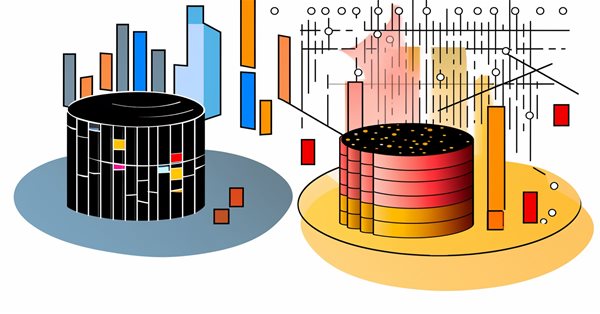
Deep Learning Forecast and ordering with Python and MetaTrader5 python package and ONNX model file
The project involves using Python for deep learning-based forecasting in financial markets. We will explore the intricacies of testing the model's performance using key metrics such as Mean Absolute Error (MAE), Mean Squared Error (MSE), and R-squared (R2) and we will learn how to wrap everything into an executable. We will also make a ONNX model file with its EA.
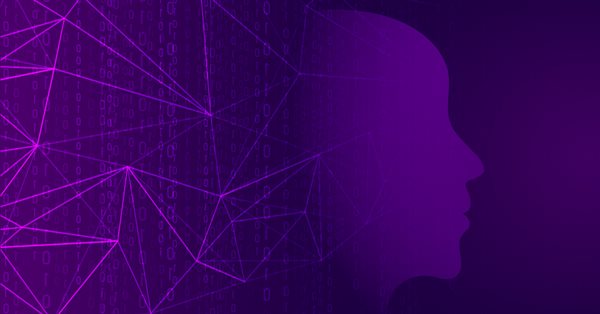
Neural networks made easy (Part 57): Stochastic Marginal Actor-Critic (SMAC)
Here I will consider the fairly new Stochastic Marginal Actor-Critic (SMAC) algorithm, which allows building latent variable policies within the framework of entropy maximization.
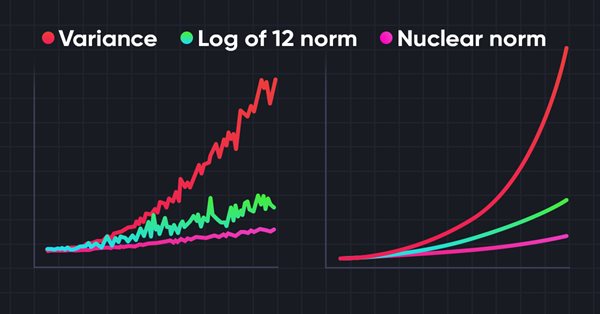
Neural networks made easy (Part 56): Using nuclear norm to drive research
The study of the environment in reinforcement learning is a pressing problem. We have already looked at some approaches previously. In this article, we will have a look at yet another method based on maximizing the nuclear norm. It allows agents to identify environmental states with a high degree of novelty and diversity.
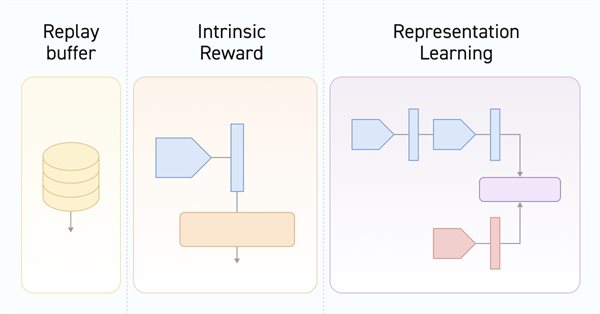
Neural networks made easy (Part 55): Contrastive intrinsic control (CIC)
Contrastive training is an unsupervised method of training representation. Its goal is to train a model to highlight similarities and differences in data sets. In this article, we will talk about using contrastive training approaches to explore different Actor skills.
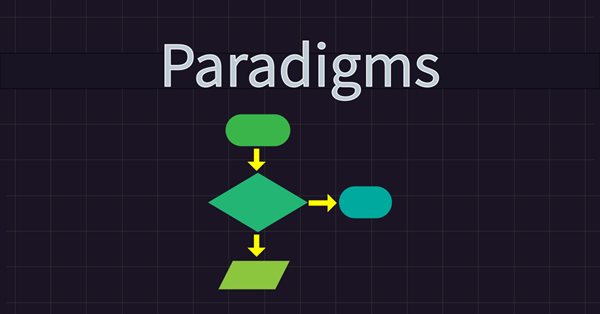
Understanding Programming Paradigms (Part 1): A Procedural Approach to Developing a Price Action Expert Advisor
Learn about programming paradigms and their application in MQL5 code. This article explores the specifics of procedural programming, offering hands-on experience through a practical example. You'll learn how to develop a price action expert advisor using the EMA indicator and candlestick price data. Additionally, the article introduces you to the functional programming paradigm.
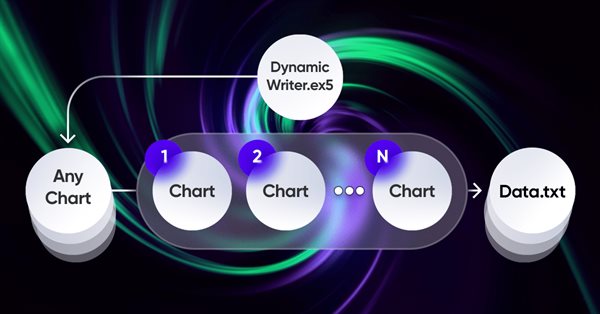
Brute force approach to patterns search (Part VI): Cyclic optimization
In this article I will show the first part of the improvements that allowed me not only to close the entire automation chain for MetaTrader 4 and 5 trading, but also to do something much more interesting. From now on, this solution allows me to fully automate both creating EAs and optimization, as well as to minimize labor costs for finding effective trading configurations.
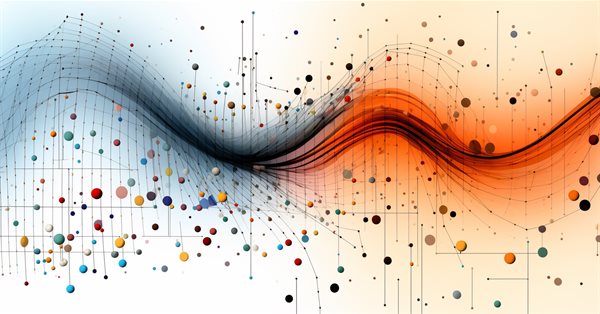
Filtering and feature extraction in the frequency domain
In this article we explore the application of digital filters on time series represented in the frequency domain so as to extract unique features that may be useful to prediction models.