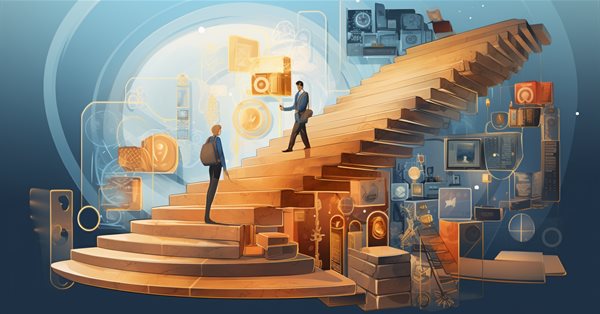
Introduction to MQL5 (Part 1): A Beginner's Guide into Algorithmic Trading
Dive into the fascinating realm of algorithmic trading with our beginner-friendly guide to MQL5 programming. Discover the essentials of MQL5, the language powering MetaTrader 5, as we demystify the world of automated trading. From understanding the basics to taking your first steps in coding, this article is your key to unlocking the potential of algorithmic trading even without a programming background. Join us on a journey where simplicity meets sophistication in the exciting universe of MQL5.
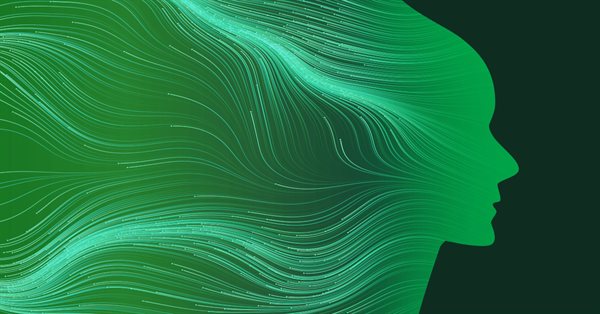
Neural networks made easy (Part 52): Research with optimism and distribution correction
As the model is trained based on the experience reproduction buffer, the current Actor policy moves further and further away from the stored examples, which reduces the efficiency of training the model as a whole. In this article, we will look at the algorithm of improving the efficiency of using samples in reinforcement learning algorithms.
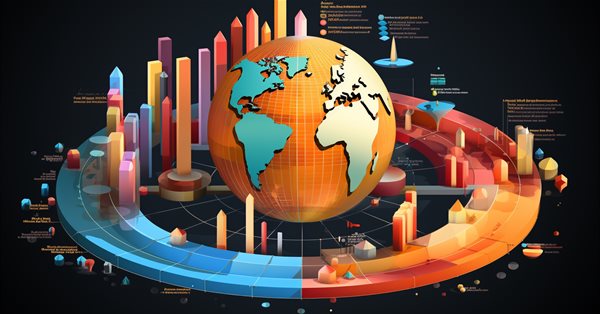
The case for using a Composite Data Set this Q4 in weighing SPDR XLY's next performance
We consider XLY, SPDR’s consumer discretionary spending ETF and see if with tools in MetaTrader’s IDE we can sift through an array of data sets in selecting what could work with a forecasting model with a forward outlook of not more than a year.
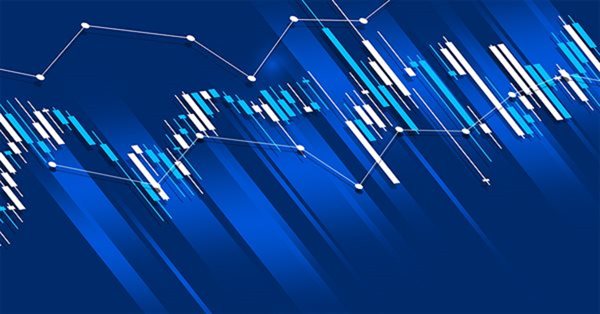
How to create a simple Multi-Currency Expert Advisor using MQL5 (Part 4): Triangular moving average — Indicator Signals
The Multi-Currency Expert Advisor in this article is Expert Advisor or trading robot that can trade (open orders, close orders and manage orders for example: Trailing Stop Loss and Trailing Profit) for more than one symbol pair only from one symbol chart. This time we will use only 1 indicator, namely Triangular moving average in multi-timeframes or single timeframe.
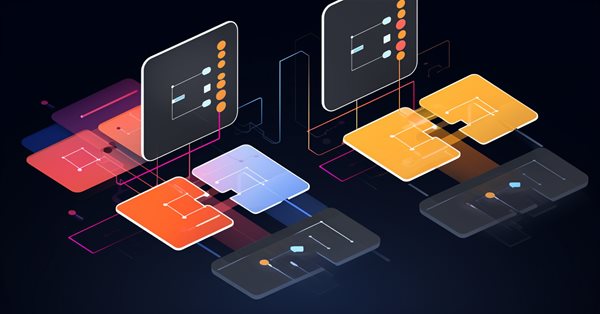
Design Patterns in software development and MQL5 (Part 2): Structural Patterns
In this article, we will continue our articles about Design Patterns after learning how much this topic is more important for us as developers to develop extendable, reliable applications not only by the MQL5 programming language but others as well. We will learn about another type of Design Patterns which is the structural one to learn how to design systems by using what we have as classes to form larger structures.
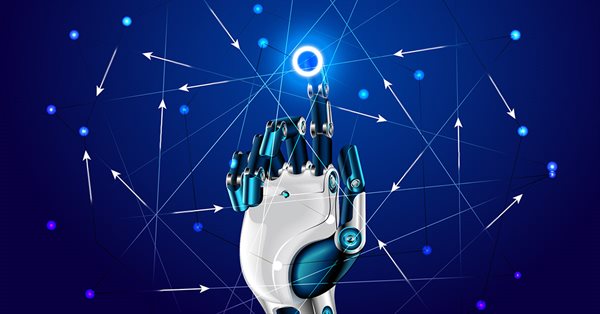
Developing a quality factor for Expert Advisors
In this article, we will see how to develop a quality score that your Expert Advisor can display in the strategy tester. We will look at two well-known calculation methods – Van Tharp and Sunny Harris.
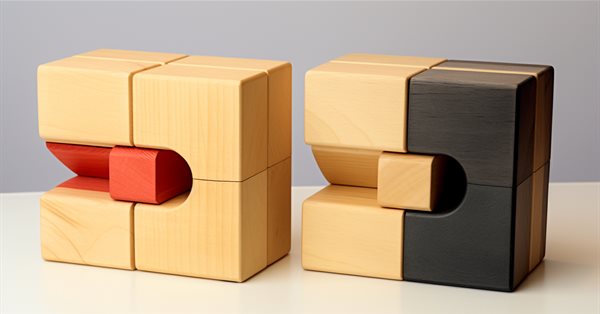
Combinatorially Symmetric Cross Validation In MQL5
In this article we present the implementation of Combinatorially Symmetric Cross Validation in pure MQL5, to measure the degree to which a overfitting may occure after optimizing a strategy using the slow complete algorithm of the Strategy Tester.
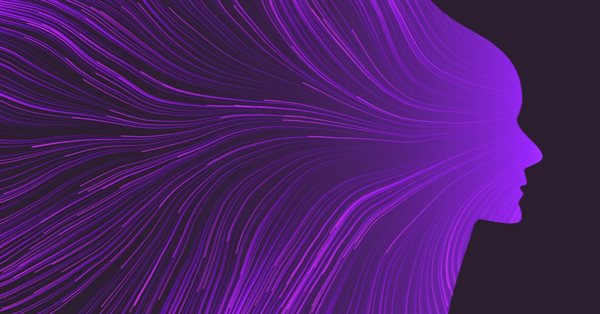
Neural networks made easy (Part 51): Behavior-Guided Actor-Critic (BAC)
The last two articles considered the Soft Actor-Critic algorithm, which incorporates entropy regularization into the reward function. This approach balances environmental exploration and model exploitation, but it is only applicable to stochastic models. The current article proposes an alternative approach that is applicable to both stochastic and deterministic models.
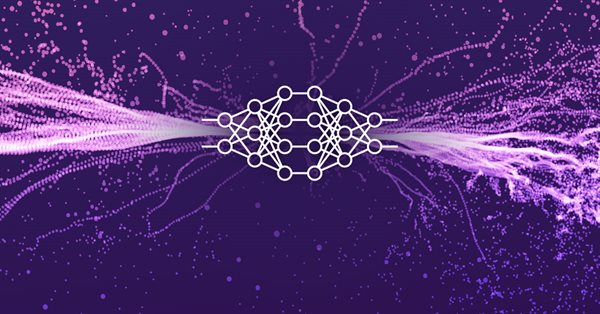
Neural networks made easy (Part 50): Soft Actor-Critic (model optimization)
In the previous article, we implemented the Soft Actor-Critic algorithm, but were unable to train a profitable model. Here we will optimize the previously created model to obtain the desired results.
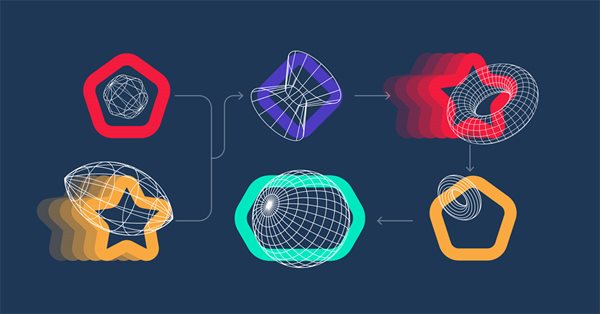
Brute force approach to patterns search (Part V): Fresh angle
In this article, I will show a completely different approach to algorithmic trading I ended up with after quite a long time. Of course, all this has to do with my brute force program, which has undergone a number of changes that allow it to solve several problems simultaneously. Nevertheless, the article has turned out to be more general and as simple as possible, which is why it is also suitable for those who know nothing about brute force.
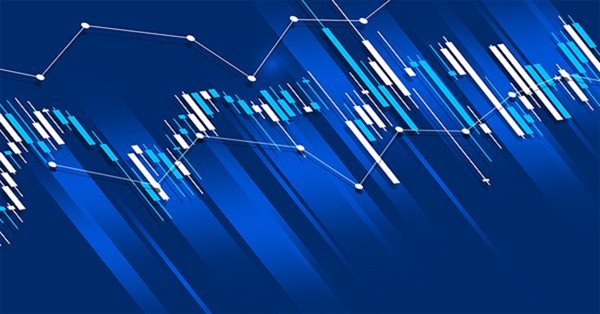
How to create a simple Multi-Currency Expert Advisor using MQL5 (Part 3): Added symbols prefixes and/or suffixes and Trading Time Session
Several fellow traders sent emails or commented about how to use this Multi-Currency EA on brokers with symbol names that have prefixes and/or suffixes, and also how to implement trading time zones or trading time sessions on this Multi-Currency EA.
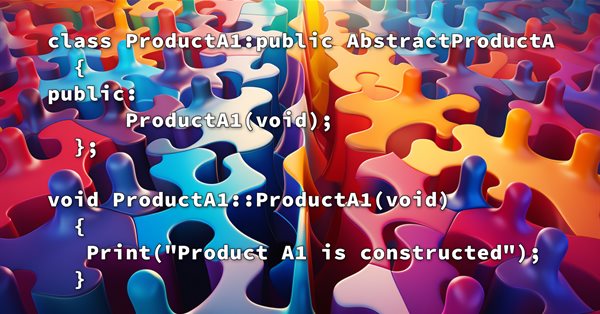
Design Patterns in software development and MQL5 (Part I): Creational Patterns
There are methods that can be used to solve many problems that can be repeated. Once understand how to use these methods it can be very helpful to create your software effectively and apply the concept of DRY ((Do not Repeat Yourself). In this context, the topic of Design Patterns will serve very well because they are patterns that provide solutions to well-described and repeated problems.
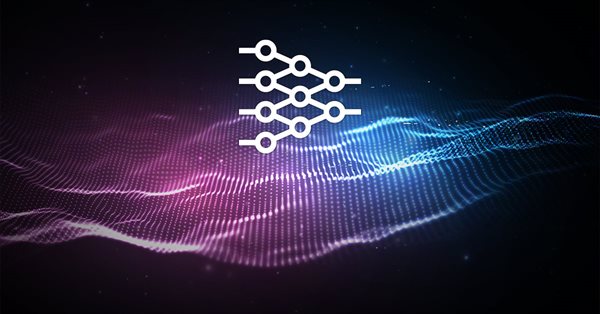
Neural networks made easy (Part 49): Soft Actor-Critic
We continue our discussion of reinforcement learning algorithms for solving continuous action space problems. In this article, I will present the Soft Actor-Critic (SAC) algorithm. The main advantage of SAC is the ability to find optimal policies that not only maximize the expected reward, but also have maximum entropy (diversity) of actions.
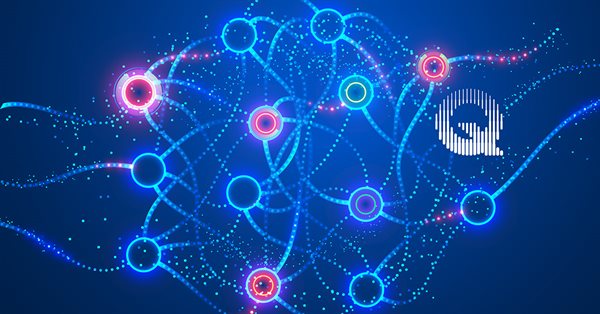
Neural networks made easy (Part 48): Methods for reducing overestimation of Q-function values
In the previous article, we introduced the DDPG method, which allows training models in a continuous action space. However, like other Q-learning methods, DDPG is prone to overestimating Q-function values. This problem often results in training an agent with a suboptimal strategy. In this article, we will look at some approaches to overcome the mentioned issue.
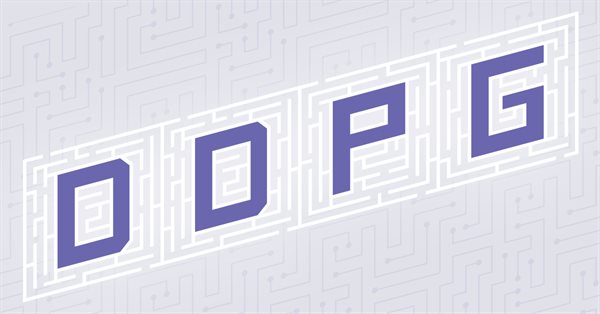
Neural networks made easy (Part 47): Continuous action space
In this article, we expand the range of tasks of our agent. The training process will include some aspects of money and risk management, which are an integral part of any trading strategy.
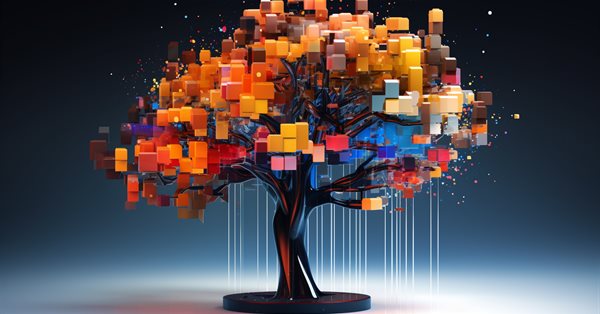
MQL5 Wizard Techniques you should know (Part 07): Dendrograms
Data classification for purposes of analysis and forecasting is a very diverse arena within machine learning and it features a large number of approaches and methods. This piece looks at one such approach, namely Agglomerative Hierarchical Classification.
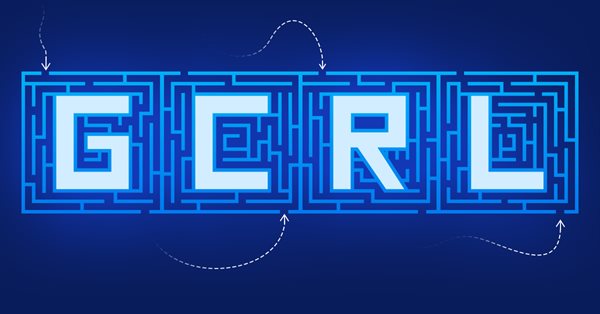
Neural networks made easy (Part 46): Goal-conditioned reinforcement learning (GCRL)
In this article, we will have a look at yet another reinforcement learning approach. It is called goal-conditioned reinforcement learning (GCRL). In this approach, an agent is trained to achieve different goals in specific scenarios.
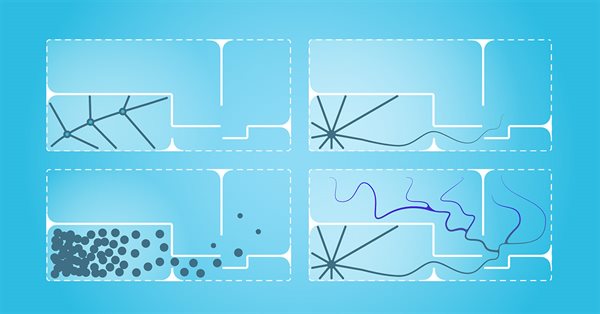
Neural networks made easy (Part 45): Training state exploration skills
Training useful skills without an explicit reward function is one of the main challenges in hierarchical reinforcement learning. Previously, we already got acquainted with two algorithms for solving this problem. But the question of the completeness of environmental research remains open. This article demonstrates a different approach to skill training, the use of which directly depends on the current state of the system.
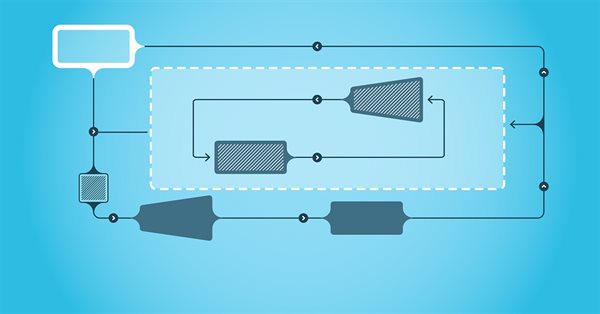
Neural networks made easy (Part 44): Learning skills with dynamics in mind
In the previous article, we introduced the DIAYN method, which offers the algorithm for learning a variety of skills. The acquired skills can be used for various tasks. But such skills can be quite unpredictable, which can make them difficult to use. In this article, we will look at an algorithm for learning predictable skills.
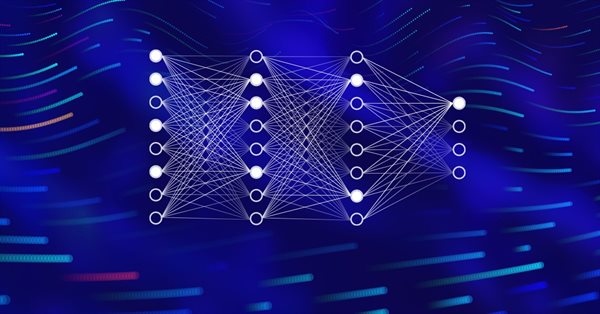
Neural networks made easy (Part 43): Mastering skills without the reward function
The problem of reinforcement learning lies in the need to define a reward function. It can be complex or difficult to formalize. To address this problem, activity-based and environment-based approaches are being explored to learn skills without an explicit reward function.
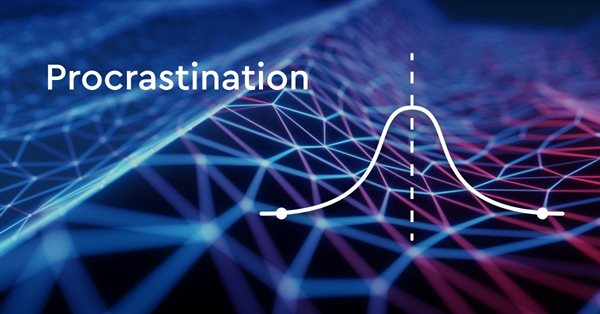
Neural networks made easy (Part 42): Model procrastination, reasons and solutions
In the context of reinforcement learning, model procrastination can be caused by several reasons. The article considers some of the possible causes of model procrastination and methods for overcoming them.
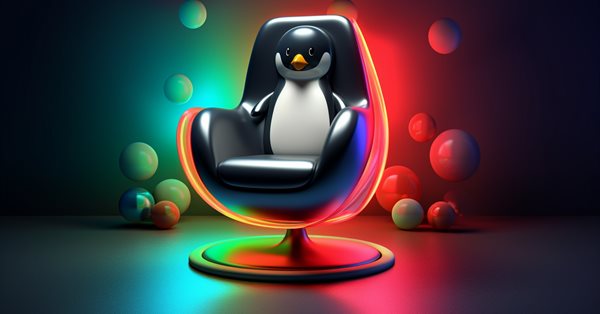
Integrate Your Own LLM into EA (Part 2): Example of Environment Deployment
With the rapid development of artificial intelligence today, language models (LLMs) are an important part of artificial intelligence, so we should think about how to integrate powerful LLMs into our algorithmic trading. For most people, it is difficult to fine-tune these powerful models according to their needs, deploy them locally, and then apply them to algorithmic trading. This series of articles will take a step-by-step approach to achieve this goal.
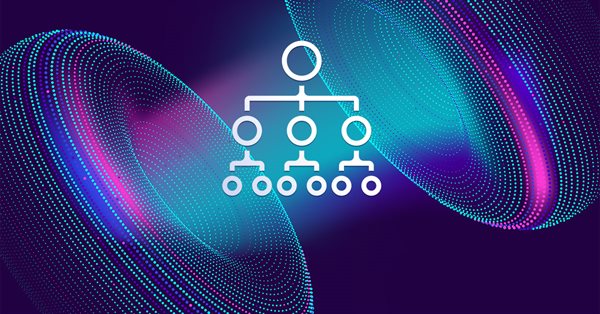
Neural networks made easy (Part 41): Hierarchical models
The article describes hierarchical training models that offer an effective approach to solving complex machine learning problems. Hierarchical models consist of several levels, each of which is responsible for different aspects of the task.
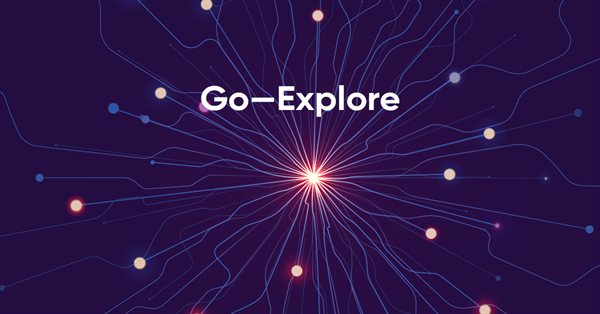
Neural networks made easy (Part 40): Using Go-Explore on large amounts of data
This article discusses the use of the Go-Explore algorithm over a long training period, since the random action selection strategy may not lead to a profitable pass as training time increases.
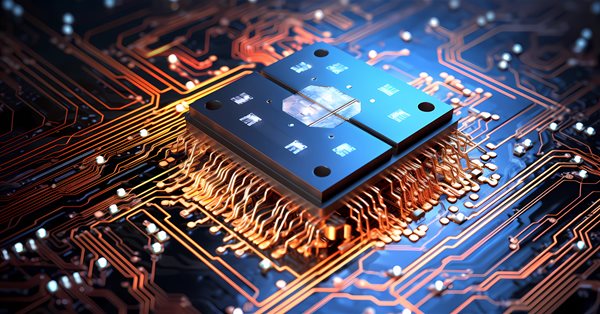
Integrate Your Own LLM into EA (Part 1): Hardware and Environment Deployment
With the rapid development of artificial intelligence today, language models (LLMs) are an important part of artificial intelligence, so we should think about how to integrate powerful LLMs into our algorithmic trading. For most people, it is difficult to fine-tune these powerful models according to their needs, deploy them locally, and then apply them to algorithmic trading. This series of articles will take a step-by-step approach to achieve this goal.
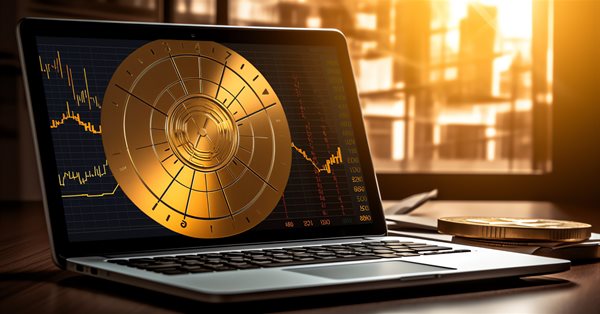
Learn how to deal with date and time in MQL5
A new article about a new important topic which is dealing with date and time. As traders or programmers of trading tools, it is very crucial to understand how to deal with these two aspects date and time very well and effectively. So, I will share some important information about how we can deal with date and time to create effective trading tools smoothly and simply without any complicity as much as I can.
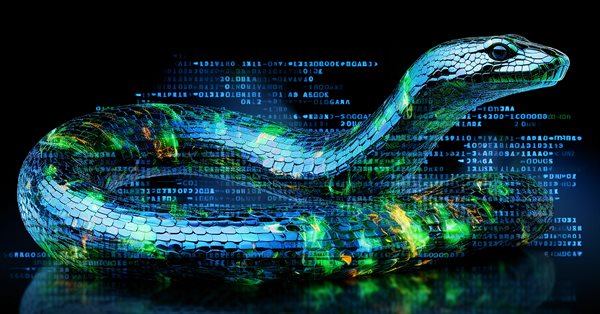
Data label for time series mining (Part 3):Example for using label data
This series of articles introduces several time series labeling methods, which can create data that meets most artificial intelligence models, and targeted data labeling according to needs can make the trained artificial intelligence model more in line with the expected design, improve the accuracy of our model, and even help the model make a qualitative leap!
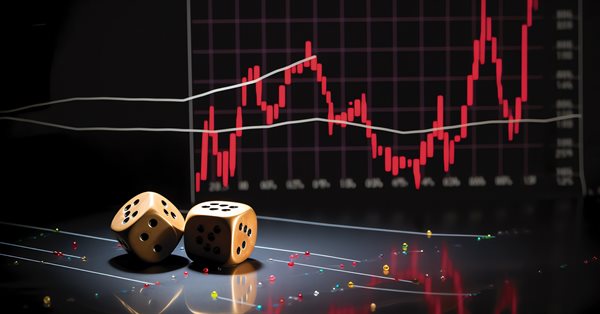
Alternative risk return metrics in MQL5
In this article we present the implementation of several risk return metrics billed as alternatives to the Sharpe ratio and examine hypothetical equity curves to analyze their characteristics.
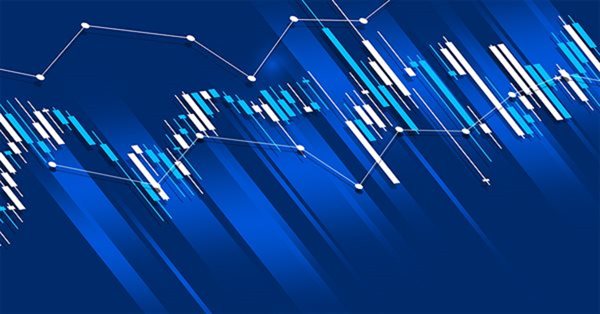
How to create a simple Multi-Currency Expert Advisor using MQL5 (Part 2): Indicator Signals: Multi Timeframe Parabolic SAR Indicator
The Multi-Currency Expert Advisor in this article is Expert Advisor or trading robot that can trade (open orders, close orders and manage orders for example: Trailing Stop Loss and Trailing Profit) for more than 1 symbol pair only from one symbol chart. This time we will use only 1 indicator, namely Parabolic SAR or iSAR in multi-timeframes starting from PERIOD_M15 to PERIOD_D1.
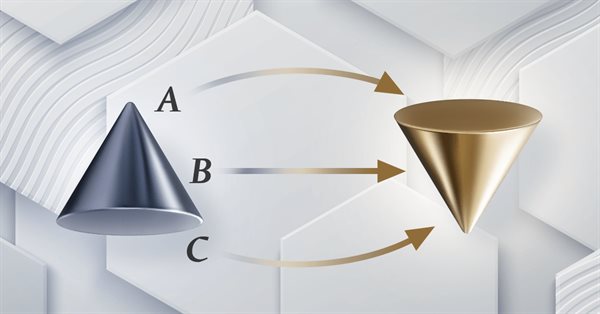
Category Theory in MQL5 (Part 22): A different look at Moving Averages
In this article we attempt to simplify our illustration of concepts covered in these series by dwelling on just one indicator, the most common and probably the easiest to understand. The moving average. In doing so we consider significance and possible applications of vertical natural transformations.
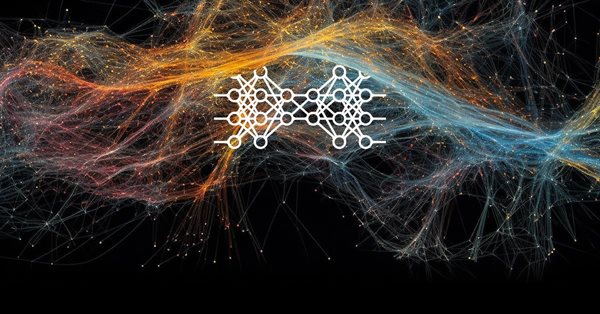
Neural networks made easy (Part 39): Go-Explore, a different approach to exploration
We continue studying the environment in reinforcement learning models. And in this article we will look at another algorithm – Go-Explore, which allows you to effectively explore the environment at the model training stage.
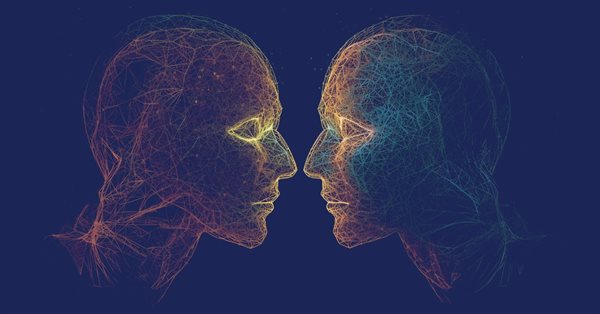
Neural networks made easy (Part 38): Self-Supervised Exploration via Disagreement
One of the key problems within reinforcement learning is environmental exploration. Previously, we have already seen the research method based on Intrinsic Curiosity. Today I propose to look at another algorithm: Exploration via Disagreement.
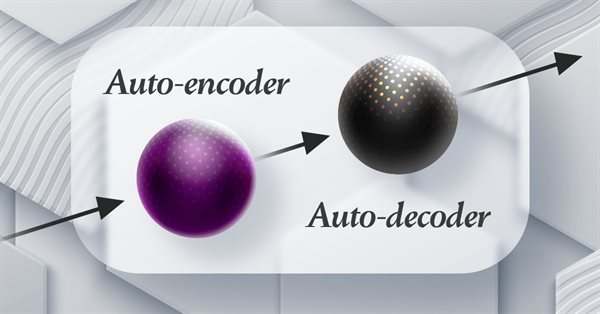
Category Theory in MQL5 (Part 20): A detour to Self-Attention and the Transformer
We digress in our series by pondering at part of the algorithm to chatGPT. Are there any similarities or concepts borrowed from natural transformations? We attempt to answer these and other questions in a fun piece, with our code in a signal class format.
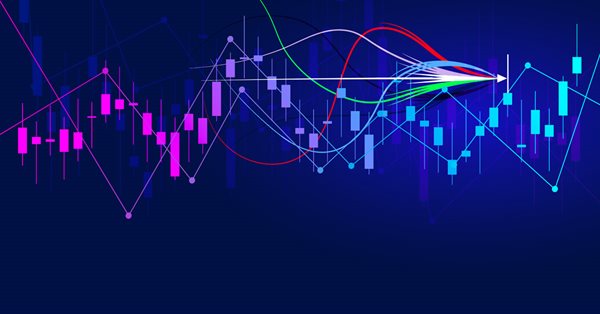
Data label for timeseries mining (Part 2):Make datasets with trend markers using Python
This series of articles introduces several time series labeling methods, which can create data that meets most artificial intelligence models, and targeted data labeling according to needs can make the trained artificial intelligence model more in line with the expected design, improve the accuracy of our model, and even help the model make a qualitative leap!
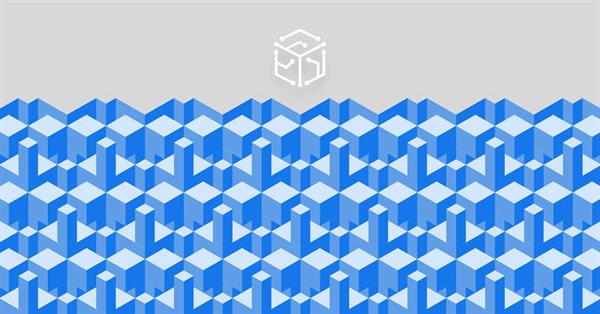
Neural networks made easy (Part 37): Sparse Attention
In the previous article, we discussed relational models which use attention mechanisms in their architecture. One of the specific features of these models is the intensive utilization of computing resources. In this article, we will consider one of the mechanisms for reducing the number of computational operations inside the Self-Attention block. This will increase the general performance of the model.
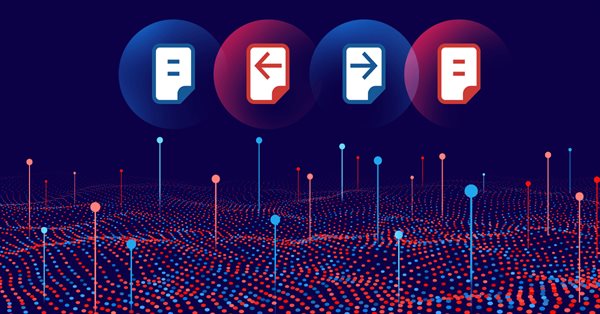
Understanding order placement in MQL5
When creating any trading system, there is a task we need to deal with effectively. This task is order placement or to let the created trading system deal with orders automatically because it is crucial in any trading system. So, you will find in this article most of the topics that you need to understand about this task to create your trading system in terms of order placement effectively.
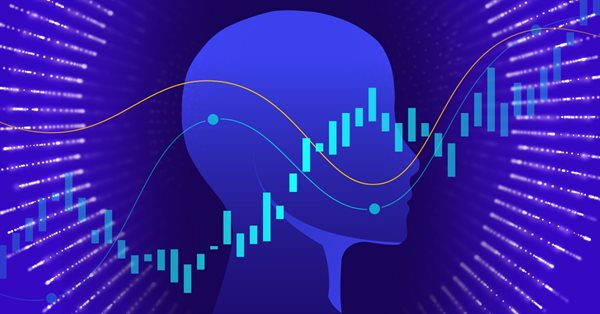
Data label for time series mining(Part 1):Make a dataset with trend markers through the EA operation chart
This series of articles introduces several time series labeling methods, which can create data that meets most artificial intelligence models, and targeted data labeling according to needs can make the trained artificial intelligence model more in line with the expected design, improve the accuracy of our model, and even help the model make a qualitative leap!
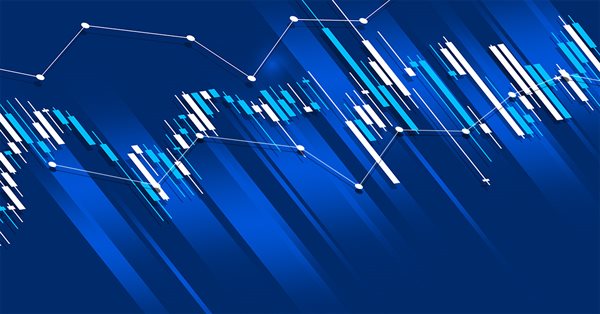
How to create a simple Multi-Currency Expert Advisor using MQL5 (Part 1): Indicator Signals based on ADX in combination with Parabolic SAR
The Multi-Currency Expert Advisor in this article is Expert Advisor or trading robot that can trade (open orders, close orders and manage orders an more) for more than 1 symbol pair only from one symbol chart.
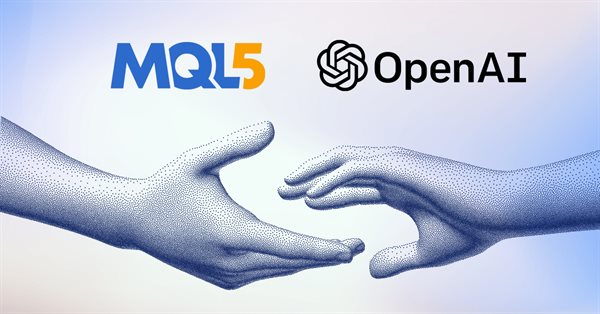
OpenAI's ChatGPT features within the framework of MQL4 and MQL5 development
In this article, we will fiddle around ChatGPT from OpenAI in order to understand its capabilities in terms of reducing the time and labor intensity of developing Expert Advisors, indicators and scripts. I will quickly navigate you through this technology and try to show you how to use it correctly for programming in MQL4 and MQL5.
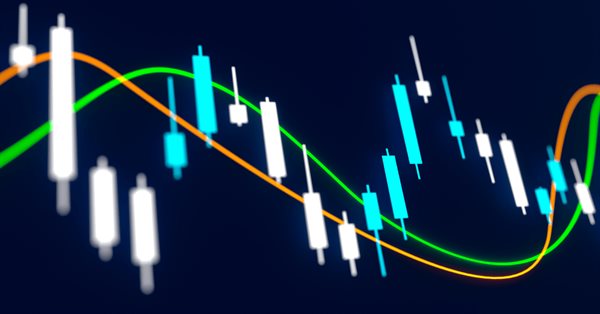
Testing different Moving Average types to see how insightful they are
We all know the importance of the Moving Average indicator for a lot of traders. There are other Moving average types that can be useful in trading, we will identify these types in this article and make a simple comparison between each one of them and the most popular simple Moving average type to see which one can show the best results.