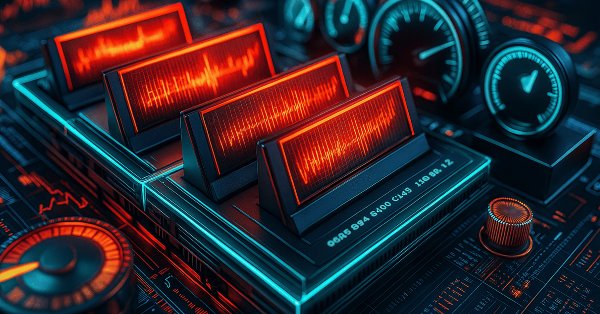
Advanced Memory Management and Optimization Techniques in MQL5
- Introduction
- Understanding Memory Management in MQL5
- Profiling and Measuring Memory Usage
- Implementing Custom Memory Pools
- Optimizing Data Structures for Trading Applications
- Advanced Techniques for High-Frequency Trading
- Conclusion
Introduction
Welcome! If you’ve spent any time building trading systems in MQL5, you’ve probably hit that frustrating wall — your Expert Advisor starts lagging, memory usage skyrockets, or worse, the whole thing crashes right when the market gets interesting. Sound familiar?
MQL5 is undeniably powerful, but with that power comes responsibility — especially when it comes to memory. Many developers focus solely on strategy logic, entry points, and risk management, while memory handling quietly becomes a ticking time bomb in the background. As your code scales — processing more symbols, higher frequencies, and heavier datasets — ignoring memory can lead to performance bottlenecks, instability, and missed opportunities.
In this article, we’re going under the hood. We’ll explore how memory really works in MQL5, the common traps that slow your systems down or cause them to fail, and — most importantly — how to fix them. You’ll learn practical optimization techniques that make your trading programs faster, leaner, and more reliable.
Here’s where efficient memory usage really matters:
-
High-Frequency Trading: Every millisecond is a potential edge — or a potential loss.
-
Multi-Timeframe Analysis: Combining charts? Expect memory pressure to multiply.
-
Heavy Indicator Logic: Complex math and big datasets can grind everything to a halt if unmanaged.
-
Backtesting Large Histories: Without smart optimization, backtests can feel like watching paint dry.
If you’re ready to get serious about performance, let’s dive in — and make your MQL5 systems as efficient as they are intelligent.
In the coming sections, we’ll go step by step—from the foundational concepts of MQL5 memory allocation to advanced techniques and code-focused examples. By following these practices, you’ll have the know-how to build faster, leaner, and more resilient trading systems capable of handling the intense demands of modern algorithmic trading. Let’s get started!
Understanding Memory Management in MQL5
When venturing into more sophisticated optimization strategies in MQL5, it’s important to first grasp how the language handles memory behind the scenes. Although MQL5 generally streamlines memory tasks compared to a lower-level language like C++, there’s still a need for developers to adopt efficient coding practices.
Differentiating Between Stack and Heap Memory
MQL5, like many modern languages, splits its memory usage between the stack and the heap:
- Stack Memory: This is where local variables go when their sizes are known at compile time. It’s managed automatically, and allocation here happens very quickly.
- Heap Memory: Used for scenarios where you need to allocate memory dynamically—perhaps when the size isn’t determined until runtime, or an object needs to stick around beyond a single function’s scope.
void ExampleFunction() { // Stack allocation - automatically managed double price = 1.2345; int volume = 100; // Heap allocation - requires proper management double dynamicArray[]; ArrayResize(dynamicArray, 1000); // Use the array... // In MQL5, the array will be automatically deallocated // when it goes out of scope, but this isn't always optimal }
While MQL5 does include automatic garbage collection, depending on it alone can still produce inefficiencies, particularly in high-frequency trading environments.
Memory Lifecycle in MQL5
To optimize performance, it helps to follow the journey of memory throughout your MQL5 program:
- Initialization: Right when your Expert Advisor (EA) or indicator starts, MQL5 carves out memory for any global variables and class instances.
- Event Handling: Each time an event fires—like OnTick() or OnCalculate()—the system sets up local variables on the stack. It may also dip into the heap if it needs more dynamic allocations.
- Deallocation: The moment local variables exit scope, the stack memory is automatically reclaimed. Heap allocations, however, are usually freed later by the garbage collector.
- Termination: Once your program shuts down, the remaining memory is fully released.
The crux of the matter is that, while MQL5 does handle deallocation, it doesn’t always do so instantly or in the most optimal way for time-sensitive trading tasks.
Common Memory Pitfalls
Despite automatic garbage collection, it’s still possible to bump into memory leaks or sluggish memory usage.
Here are some frequent culprits:
- Excessive Object Creation: Continuously spinning up new objects in frequently called functions (like OnTick) can eat up resources.
- Large Arrays: Storing big arrays that hang around for the entire run of the program might gobble up memory unnecessarily.
- Circular References: If two objects keep references to each other, it can postpone or disrupt garbage collection.
- Improper Resource Management: Forgetting to close files, database connections, or other system resources can lead to wasted memory.
// Inefficient approach - creates new arrays on every tick void OnTick() { // This creates a new array on every tick double prices[]; ArrayResize(prices, 1000); // Fill the array with price data for(int i = 0; i < 1000; i++) { prices[i] = iClose(_Symbol, PERIOD_M1, i); } // Process the data... // Array will be garbage collected eventually, but this // creates unnecessary memory churn }
A more efficient approach would be:
// Class member variable - created once double prices[]; void OnTick() { // Reuse the existing array for(int i = 0; i < 1000; i++) { prices[i] = iClose(_Symbol, PERIOD_M1, i); } // Process the data... }
Often, minor tweaks—like reusing objects instead of repeatedly instantiating them—can make a substantial difference, especially in fast-paced trading environments.
Memory Allocation Patterns in MQL5
MQL5 uses different memory allocation patterns depending on the data type:
Finally, it’s helpful to know how MQL5 allocates common data types:
-
Primitive Types ( int , double , bool , etc.)
These are usually allocated on the stack when declared as local variables. -
Arrays
Dynamic arrays in MQL5 are stored on the heap. -
Strings
MQL5 strings use reference counting and live on the heap. -
Objects
Instances of classes also live on the heap.
By keeping these allocation patterns in mind, you’ll be better equipped to craft code that’s more efficient, stable, and optimized for real-world trading conditions.
Profiling and Measuring Memory Usage
When it comes to streamlining memory usage in MQL5, the first step is to pinpoint exactly where the bottlenecks occur. Although MQL5 lacks native memory profiling tools, we can roll up our sleeves and craft a homemade approach.
Building a Simple Memory Profiler
To get a better handle on memory usage, we can set up a minimalistic profiling class that takes advantage of the TERMINAL_MEMORY_AVAILABLE property. By comparing the initial and current available memory, you can keep tabs on how much memory your application is consuming.
//+------------------------------------------------------------------+ //| MemoryProfiler class for tracking memory usage | //+------------------------------------------------------------------+ class CMemoryProfiler { private: ulong m_startMemory; ulong m_peakMemory; string m_profileName; public: // Constructor CMemoryProfiler(string profileName) { m_profileName = profileName; m_startMemory = TerminalInfoInteger(TERMINAL_MEMORY_AVAILABLE); m_peakMemory = m_startMemory; Print("Memory profiling started for: ", m_profileName); Print("Initial available memory: ", m_startMemory, " bytes"); } // Update peak memory usage void UpdatePeak() { ulong currentMemory = TerminalInfoInteger(TERMINAL_MEMORY_AVAILABLE); if(currentMemory < m_peakMemory) m_peakMemory = currentMemory; } // Get memory usage ulong GetUsedMemory() { return m_startMemory - TerminalInfoInteger(TERMINAL_MEMORY_AVAILABLE); } // Get peak memory usage ulong GetPeakUsage() { return m_startMemory - m_peakMemory; } // Print memory usage report void PrintReport() { ulong currentUsage = GetUsedMemory(); ulong peakUsage = GetPeakUsage(); Print("Memory profile report for: ", m_profileName); Print("Current memory usage: ", currentUsage, " bytes"); Print("Peak memory usage: ", peakUsage, " bytes"); } // Destructor ~CMemoryProfiler() { PrintReport(); } };
Once you have the CMemoryProfiler class in your project, putting it to work looks something like this:
void OnStart() { // Create a profiler for the entire function CMemoryProfiler profiler("OnStart function"); // Allocate some arrays double largeArray1[]; ArrayResize(largeArray1, 100000); profiler.UpdatePeak(); double largeArray2[]; ArrayResize(largeArray2, 200000); profiler.UpdatePeak(); // The profiler will print a report when it goes out of scope }
The profiler initializes by recording a baseline of the available memory the moment it’s constructed. Each time you call UpdatePeak() , it checks if your application’s current memory footprint has exceeded the previously measured high-water mark. The GetUsedMemory() and GetPeakUsage() methods tell you how much memory you’ve used since the baseline, while PrintReport() logs a summary to the terminal. That summary is automatically generated when the profiler goes out of scope, courtesy of the class destructor.
Keep in mind that this approach only measures the terminal’s overall memory usage rather than your specific program’s consumption. Still, it’s a handy way to gain a bird’s-eye view of how your memory usage evolves over time.
Benchmarking Memory Operations
Optimizing memory usage isn’t just about knowing how much memory you’re using—it’s also about understanding how quickly different memory operations execute. By timing various operations, you can see where inefficiencies hide and discover potential performance tweaks.
//+------------------------------------------------------------------+ //| Benchmark different memory operations | //+------------------------------------------------------------------+ void BenchmarkMemoryOperations() { const int iterations = 1000; const int arraySize = 10000; // Benchmark array allocation ulong startTime = GetMicrosecondCount(); for(int i = 0; i < iterations; i++) { double tempArray[]; ArrayResize(tempArray, arraySize); // Do something minimal to prevent optimization tempArray[0] = 1.0; } ulong allocTime = GetMicrosecondCount() - startTime; // Benchmark array reuse double reuseArray[]; ArrayResize(reuseArray, arraySize); startTime = GetMicrosecondCount(); for(int i = 0; i < iterations; i++) { ArrayInitialize(reuseArray, 0); reuseArray[0] = 1.0; } ulong reuseTime = GetMicrosecondCount() - startTime; // Benchmark string operations startTime = GetMicrosecondCount(); for(int i = 0; i < iterations; i++) { string tempString = "Base string "; for(int j = 0; j < 100; j++) { // Inefficient string concatenation tempString = tempString + IntegerToString(j); } } ulong stringConcatTime = GetMicrosecondCount() - startTime; // Benchmark string builder approach startTime = GetMicrosecondCount(); for(int i = 0; i < iterations; i++) { string tempString = "Base string "; string parts[]; ArrayResize(parts, 100); for(int j = 0; j < 100; j++) { parts[j] = IntegerToString(j); } tempString = tempString + StringImplode(" ", parts); } ulong stringBuilderTime = GetMicrosecondCount() - startTime; // Print results Print("Memory operation benchmarks:"); Print("Array allocation time: ", allocTime, " microseconds"); Print("Array reuse time: ", reuseTime, " microseconds"); Print("String concatenation time: ", stringConcatTime, " microseconds"); Print("String builder time: ", stringBuilderTime, " microseconds"); Print("Reuse vs. Allocation speedup: ", (double)allocTime / reuseTime); Print("String builder vs. Concatenation speedup: ", (double)stringConcatTime / stringBuilderTime); }
This simple testing function demonstrates how to measure the execution speed of various memory-intensive tasks. It compares the performance of allocating arrays repeatedly versus reusing a single pre-allocated array, as well as the difference between straightforward string concatenation and a “string builder” style approach. These tests use GetMicrosecondCount() to measure time in microseconds, ensuring you get a precise view of any delays.
Typically, your results will show that reusing arrays offers a clear performance edge over allocating new ones in every loop, and that collecting string parts in an array (and then joining them) beats piecemeal concatenations. These distinctions become especially critical in high-frequency trading scenarios where every fraction of a millisecond can matter.
// Helper function for string array joining string StringImplode(string separator, string &array[]) { string result = ""; int size = ArraySize(array); for(int i = 0; i < size; i++) { if(i > 0) result += separator; result += array[i]; } return result; }
When you run the benchmark, you’ll come away with concrete data on how different memory operations stack up in MQL5. Armed with these insights, you’ll be well-prepared to make adjustments that keep your trading robots running lean and mean.
Implementing Custom Memory Pools
When performance is paramount, one standout strategy to streamline memory usage is memory pooling. Rather than constantly asking the system for memory and then giving it back, the trick is to pre-allocate a chunk of memory and manage it ourselves. This section explores how to do this in both simple and advanced scenarios.
Basic Object Pool Implementation
Imagine you have a class called CTradeSignal that you instantiate and destroy often—maybe in a high-frequency trading system. Instead of hitting the memory allocator repeatedly, you create a dedicated pool for these objects. Below is a basic example:
//+------------------------------------------------------------------+ //| Trade signal class that will be pooled | //+------------------------------------------------------------------+ class CTradeSignal { public: datetime time; double price; ENUM_ORDER_TYPE type; double volume; bool isValid; // Reset the object for reuse void Reset() { time = 0; price = 0.0; type = ORDER_TYPE_BUY; volume = 0.0; isValid = false; } }; //+------------------------------------------------------------------+ //| Object pool for CTradeSignal instances | //+------------------------------------------------------------------+ class CTradeSignalPool { private: CTradeSignal* m_pool[]; int m_poolSize; int m_nextAvailable; public: // Constructor CTradeSignalPool(int initialSize = 100) { m_poolSize = initialSize; ArrayResize(m_pool, m_poolSize); m_nextAvailable = 0; // Pre-allocate objects for(int i = 0; i < m_poolSize; i++) { m_pool[i] = new CTradeSignal(); } Print("Trade signal pool initialized with ", m_poolSize, " objects"); } // Get an object from the pool CTradeSignal* Acquire() { // If we've used all objects, expand the pool if(m_nextAvailable >= m_poolSize) { int oldSize = m_poolSize; m_poolSize *= 2; // Double the pool size ArrayResize(m_pool, m_poolSize); // Allocate new objects for(int i = oldSize; i < m_poolSize; i++) { m_pool[i] = new CTradeSignal(); } Print("Trade signal pool expanded to ", m_poolSize, " objects"); } // Get the next available object CTradeSignal* signal = m_pool[m_nextAvailable++]; signal.Reset(); // Ensure it's in a clean state return signal; } // Return an object to the pool void Release(CTradeSignal* &signal) { if(signal == NULL) return; // In a more sophisticated implementation, we would // actually track which objects are in use and reuse them. // For simplicity, we're just decrementing the counter. if(m_nextAvailable > 0) m_nextAvailable--; signal = NULL; // Clear the reference } // Destructor ~CTradeSignalPool() { // Clean up all allocated objects for(int i = 0; i < m_poolSize; i++) { delete m_pool[i]; } Print("Trade signal pool destroyed"); } };
In the above snippet, CTradeSignalPool pre-allocates a batch of CTradeSignal objects and carefully manages their lifecycles. When you call Acquire() , the pool will hand you an available object. If it runs out of available ones, it’ll enlarge itself and keep going. Once you’re done with an object, Release() hands it back into the pool’s custody.
The main benefit here is a big reduction in the overhead that comes from allocating and deallocating memory all the time. This is particularly handy when you’re churning through objects at a rapid clip, such as trade signals in a high-speed environment.
Below is a short example of how you might use this pool:
// Global pool instance CTradeSignalPool* g_signalPool = NULL; void OnInit() { // Initialize the pool g_signalPool = new CTradeSignalPool(100); } void OnTick() { // Acquire a signal from the pool CTradeSignal* signal = g_signalPool.Acquire(); // Set signal properties signal.time = TimeCurrent(); signal.price = SymbolInfoDouble(_Symbol, SYMBOL_ASK); signal.type = ORDER_TYPE_BUY; signal.volume = 0.1; signal.isValid = true; // Process the signal... // Return the signal to the pool when done g_signalPool.Release(signal); } void OnDeinit(const int reason) { // Clean up the pool delete g_signalPool; g_signalPool = NULL; }
Because the pool recycles its objects, it cuts down on the repeated creation/destruction costs you’d otherwise run into.
Advanced Memory Pool for Variable-Size Allocations
Sometimes you’re faced with more complex requirements, like needing to handle differently sized chunks of memory. For those cases, you can build a more advanced pool:
//+------------------------------------------------------------------+ //| Advanced memory pool for variable-size allocations | //| MQL5 version without raw pointer arithmetic | //+------------------------------------------------------------------+ #property strict class CMemoryPool { private: // Usage tracking bool m_blockUsage[]; // Size settings int m_totalSize; // Total bytes in the pool int m_blockSize; // Size of each block // Statistics int m_used; // How many bytes are currently in use public: // Memory buffer (dynamic array of bytes) uchar m_memory[]; // Constructor CMemoryPool(const int totalSize=1024*1024, // default 1 MB const int blockSize=1024) // default 1 KB blocks { m_totalSize = totalSize; m_blockSize = blockSize; m_used = 0; // Allocate the memory pool ArrayResize(m_memory, m_totalSize); // Initialize block usage tracking int numBlocks = m_totalSize / m_blockSize; ArrayResize(m_blockUsage, numBlocks); ArrayInitialize(m_blockUsage, false); Print("Memory pool initialized: ", m_totalSize, " bytes, ", numBlocks, " blocks of ", m_blockSize, " bytes each"); } // Allocate memory from the pool // Returns an offset (>= 0) if successful, or -1 on failure int Allocate(const int size) { // Round up how many blocks are needed int blocksNeeded = (size + m_blockSize - 1) / m_blockSize; int consecutive = 0; int startBlock = -1; // Search for consecutive free blocks int numBlocks = ArraySize(m_blockUsage); for(int i=0; i < numBlocks; i++) { if(!m_blockUsage[i]) { // Found a free block if(consecutive == 0) startBlock = i; consecutive++; // If we found enough blocks, stop if(consecutive >= blocksNeeded) break; } else { // Reset consecutive = 0; startBlock = -1; } } // If we couldn't find enough consecutive blocks if(consecutive < blocksNeeded) { Print("Memory pool allocation failed: needed ", blocksNeeded, " consecutive blocks"); return -1; // indicate failure } // Mark the found blocks as used for(int b=startBlock; b < startBlock + blocksNeeded; b++) { m_blockUsage[b] = true; } // Increase usage m_used += blocksNeeded * m_blockSize; // Return the offset in bytes where allocation starts return startBlock * m_blockSize; } // Free memory (by offset) void Free(const int offset) { // Validate offset if(offset < 0 || offset >= m_totalSize) { Print("Memory pool error: invalid offset in Free()"); return; } // Determine the starting block int startBlock = offset / m_blockSize; // Walk forward, freeing used blocks int numBlocks = ArraySize(m_blockUsage); for(int b=startBlock; b < numBlocks; b++) { if(!m_blockUsage[b]) break; // found an already-free block => done // Free it m_blockUsage[b] = false; m_used -= m_blockSize; } } // Get usage statistics in % double GetUsagePercentage() const { return (double)m_used / (double)m_totalSize * 100.0; } // Destructor ~CMemoryPool() { // Optionally free arrays (usually automatic at script end) ArrayFree(m_memory); ArrayFree(m_blockUsage); Print("Memory pool destroyed. Final usage: ", GetUsagePercentage(), "% of ", m_totalSize, " bytes"); } }; //+------------------------------------------------------------------+ //| Example usage in an Expert Advisor | //+------------------------------------------------------------------+ int OnInit(void) { // Create a memory pool CMemoryPool pool(1024*1024, 1024); // 1 MB total, 1 KB block size // Allocate 500 bytes from the pool int offset = pool.Allocate(500); if(offset >= 0) { // Write something in the allocated area pool.m_memory[offset] = 123; Print("Wrote 123 at offset=", offset, " usage=", pool.GetUsagePercentage(), "%"); // Free this block pool.Free(offset); Print("Freed offset=", offset, " usage=", pool.GetUsagePercentage(), "%"); } return(INIT_SUCCEEDED); } void OnTick(void) { // ... }
This CMemoryPool class sets up a large, pre-allocated memory buffer, then slices it into fixed-size pieces. When you request memory, it locates enough adjacent blocks to satisfy that need, flags them as occupied, and gives back a pointer to the start of that series of blocks. When you free the memory, it reverts those blocks to “available” status.
Instead of C++-style allocations, this approach uses MQL5 array functions—like ArrayResize , ArrayInitialize , and ArrayFree —so it fits neatly into MQL5’s memory ecosystem. It also leverages GetPointer() , which offers a safe way to handle array pointers in MQL5.
Here’s why this approach stands out:
- Reduced Fragmentation: Handling your memory in tidy, fixed-size chunks helps stave off the fragmentation headaches that come from frequent allocations.
- Improved Performance: Asking for a block of memory from your own pool is typically faster than dipping into the system allocator each time.
- Enhanced Visibility: Detailed usage stats from your pool can shine a light on any memory-related trouble spots.
- Predictability: Pre-allocation cuts down on the odds of out-of-memory errors at a critical juncture.
This more robust pool is perfect when you need memory blocks of different sizes, say for intricate data structures or dynamic workloads that shift frequently. By tailoring your pools—be it a simple object pool or a more powerful variable-size pool—you can keep memory usage under tight control and streamline performance in demanding applications.
Optimizing Data Structures for Trading Applications
When handling time series in trading environments, you need data structures that won’t let performance lag behind the market. Let’s explore two powerful strategies for storing and retrieving your price data with maximum efficiency.
Time Series Data Storage That Never Misses a Beat
A workhorse in trading systems is the price history buffer—and an optimized circular buffer can shoulder the burden with ease. Below is an example of how you might implement one:
//+------------------------------------------------------------------+ //| Circular buffer for price data | //+------------------------------------------------------------------+ class CPriceBuffer { private: double m_prices[]; int m_capacity; int m_head; int m_size; public: // Constructor CPriceBuffer(int capacity = 1000) { m_capacity = capacity; ArrayResize(m_prices, m_capacity); m_head = 0; m_size = 0; } // Add a price to the buffer void Add(double price) { m_prices[m_head] = price; m_head = (m_head + 1) % m_capacity; if(m_size < m_capacity) m_size++; } // Get a price at a specific index (0 is the most recent) double Get(int index) { if(index < 0 || index >= m_size) return 0.0; int actualIndex = (m_head - 1 - index + m_capacity) % m_capacity; return m_prices[actualIndex]; } // Get the current size int Size() { return m_size; } // Get the capacity int Capacity() { return m_capacity; } // Clear the buffer void Clear() { m_head = 0; m_size = 0; } // Calculate simple moving average double SMA(int period) { if(period <= 0 || period > m_size) return 0.0; double sum = 0.0; for(int i = 0; i < period; i++) { sum += Get(i); } return sum / period; } };
Here, the CPriceBuffer class uses a circular buffer designed around a fixed-size array. The “head” pointer wraps around the array’s end, making it possible to add new price entries without expensive resizing operations. When the buffer reaches capacity, it simply overwrites the oldest entries with fresh data, maintaining a seamless, sliding window of recent prices.
Why this approach is so efficient:
- Memory is pre-allocated and reused, eliminating the overhead of constant expansions.
- Adding new prices and fetching the most recent data both happen in O(1) time.
- The sliding window mechanism automatically manages old and new entries without hassle.
Below is a quick snippet showing how to put this to use:
// Global price buffer CPriceBuffer* g_priceBuffer = NULL; void OnInit() { // Initialize the price buffer g_priceBuffer = new CPriceBuffer(5000); } void OnTick() { // Add current price to the buffer double price = SymbolInfoDouble(_Symbol, SYMBOL_BID); g_priceBuffer.Add(price); // Calculate moving averages double sma20 = g_priceBuffer.SMA(20); double sma50 = g_priceBuffer.SMA(50); // Trading logic based on moving averages... } void OnDeinit(const int reason) { // Clean up delete g_priceBuffer; g_priceBuffer = NULL; }
Cache-Friendly Structures for Extra Zip
Modern CPUs thrive on cache efficiency. By arranging data so the processor fetches only the parts it needs, you can significantly cut down on wasted time. Take a look at this layout for storing OHLC (Open, High, Low, Close) data:
//+------------------------------------------------------------------+ //| Cache-friendly OHLC data structure | //+------------------------------------------------------------------+ class COHLCData { private: int m_capacity; int m_size; // Structure of arrays (SoA) layout for better cache locality datetime m_time[]; double m_open[]; double m_high[]; double m_low[]; double m_close[]; long m_volume[]; public: // Constructor COHLCData(int capacity = 1000) { m_capacity = capacity; m_size = 0; // Allocate arrays ArrayResize(m_time, m_capacity); ArrayResize(m_open, m_capacity); ArrayResize(m_high, m_capacity); ArrayResize(m_low, m_capacity); ArrayResize(m_close, m_capacity); ArrayResize(m_volume, m_capacity); } // Add a new bar bool Add(datetime time, double open, double high, double low, double close, long volume) { if(m_size >= m_capacity) return false; m_time[m_size] = time; m_open[m_size] = open; m_high[m_size] = high; m_low[m_size] = low; m_close[m_size] = close; m_volume[m_size] = volume; m_size++; return true; } // Get bar data by index bool GetBar(int index, datetime &time, double &open, double &high, double &low, double &close, long &volume) { if(index < 0 || index >= m_size) return false; time = m_time[index]; open = m_open[index]; high = m_high[index]; low = m_low[index]; close = m_close[index]; volume = m_volume[index]; return true; } // Get size int Size() { return m_size; } // Process all high values (example of cache-friendly operation) double CalculateAverageHigh() { if(m_size == 0) return 0.0; double sum = 0.0; for(int i = 0; i < m_size; i++) { sum += m_high[i]; } return sum / m_size; } // Process all low values (example of cache-friendly operation) double CalculateAverageLow() { if(m_size == 0) return 0.0; double sum = 0.0; for(int i = 0; i < m_size; i++) { sum += m_low[i]; } return sum / m_size; } };
The COHLCData class breaks each attribute (like high or low) into its own array—a Structure of Arrays (SoA)—instead of the more traditional Array of Structures (AoS). Why does this matter? Let’s say you want to calculate the average of all the “high” values. With a SoA setup, the processor glides through a contiguous array of highs, making fewer trips to memory. In contrast, an AoS forces the CPU to jump past open, low, close, and volume data just to grab each high value.
With COHLCData , you’ll find it straightforward to:
- Add new OHLC bars on the fly.
- Retrieve specific bars by index.
- Run calculations on any single field (like all highs) without tripping over unrelated data.
This design choice means your technical analysis—whether it’s moving averages, volatility calculations, or just scanning for breakouts—runs far more efficiently thanks to better cache locality.
Advanced Techniques for High-Frequency Trading
High-frequency trading (HFT) demands extremely low latency and constant performance. Even the slightest slowdown can disrupt trade execution and result in missed opportunities. Below, we explore two pivotal approaches—pre-allocation and simulated memory mapping—that can help keep latency to an absolute minimum in MQL5.
Pre-allocation Strategies
When your system needs to respond within microseconds, you can’t afford the unpredictable delays caused by on-the-fly memory allocation. The solution is pre-allocation—reserve in advance all the memory your application might possibly need, so you never have to allocate more during peak operation times.
//+------------------------------------------------------------------+ //| Pre-allocation example for high-frequency trading | //+------------------------------------------------------------------+ class CHFTSystem { private: // Pre-allocated arrays for price data double m_bidPrices[]; double m_askPrices[]; datetime m_times[]; // Pre-allocated arrays for calculations double m_tempArray1[]; double m_tempArray2[]; double m_tempArray3[]; // Pre-allocated string buffers string m_logMessages[]; int m_logIndex; int m_capacity; int m_dataIndex; public: // Constructor CHFTSystem(int capacity = 10000) { m_capacity = capacity; m_dataIndex = 0; m_logIndex = 0; // Pre-allocate all arrays ArrayResize(m_bidPrices, m_capacity); ArrayResize(m_askPrices, m_capacity); ArrayResize(m_times, m_capacity); ArrayResize(m_tempArray1, m_capacity); ArrayResize(m_tempArray2, m_capacity); ArrayResize(m_tempArray3, m_capacity); ArrayResize(m_logMessages, 1000); // Pre-allocate log buffer Print("HFT system initialized with capacity for ", m_capacity, " data points"); } // Add price data void AddPriceData(double bid, double ask) { // Use modulo to create a circular buffer effect int index = m_dataIndex % m_capacity; m_bidPrices[index] = bid; m_askPrices[index] = ask; m_times[index] = TimeCurrent(); m_dataIndex++; } // Log a message without allocating new strings void Log(string message) { int index = m_logIndex % 1000; m_logMessages[index] = message; m_logIndex++; } // Perform calculations using pre-allocated arrays double CalculateSpread(int lookback = 100) { int available = MathMin(m_dataIndex, m_capacity); int count = MathMin(lookback, available); if(count <= 0) return 0.0; double sumSpread = 0.0; for(int i = 0; i < count; i++) { int index = (m_dataIndex - 1 - i + m_capacity) % m_capacity; sumSpread += m_askPrices[index] - m_bidPrices[index]; } return sumSpread / count; } };
The CHFTSystem class illustrates how pre-allocation can be integrated into an HFT framework. It sets up all arrays and buffers ahead of time, ensuring that no additional memory requests occur once the trading engine is live. Circular buffers are used to maintain a sliding window of recent price data, which eliminates costly reallocation. Temporary arrays for calculations and a dedicated log message buffer are also set up in advance. By doing so, this strategy avoids the risk of sudden allocation spikes when market conditions are at their most critical.
Memory-Mapped Files for Large Datasets
Some trading strategies rely on huge amounts of historical data—sometimes more than your available RAM can handle. While MQL5 does not support native memory-mapped files, you can emulate the approach using standard file I/O:
//+------------------------------------------------------------------+ //| Simple memory-mapped file simulation for large datasets | //+------------------------------------------------------------------+ class CDatasetMapper { private: int m_fileHandle; string m_fileName; int m_recordSize; int m_recordCount; // Cache for recently accessed records double m_cache[]; int m_cacheSize; int m_cacheStart; public: // Constructor CDatasetMapper(string fileName, int recordSize, int cacheSize = 1000) { m_fileName = fileName; m_recordSize = recordSize; m_cacheSize = cacheSize; // Open or create the file m_fileHandle = FileOpen(m_fileName, FILE_READ|FILE_WRITE|FILE_BIN); if(m_fileHandle != INVALID_HANDLE) { // Get file size and calculate record count m_recordCount = (int)(FileSize(m_fileHandle) / (m_recordSize * sizeof(double))); // Initialize cache ArrayResize(m_cache, m_cacheSize * m_recordSize); m_cacheStart = -1; // Cache is initially empty Print("Dataset mapper initialized: ", m_fileName, ", ", m_recordCount, " records"); } else { Print("Failed to open dataset file: ", m_fileName, ", error: ", GetLastError()); } } // Add a record to the dataset bool AddRecord(double &record[]) { if(m_fileHandle == INVALID_HANDLE || ArraySize(record) != m_recordSize) return false; // Seek to the end of the file FileSeek(m_fileHandle, 0, SEEK_END); // Write the record int written = FileWriteArray(m_fileHandle, record, 0, m_recordSize); if(written == m_recordSize) { m_recordCount++; return true; } return false; } // Get a record from the dataset bool GetRecord(int index, double &record[]) { if(m_fileHandle == INVALID_HANDLE || index < 0 || index >= m_recordCount) return false; // Check if the record is in cache if(index >= m_cacheStart && index < m_cacheStart + m_cacheSize) { // Copy from cache int cacheOffset = (index - m_cacheStart) * m_recordSize; ArrayCopy(record, m_cache, 0, cacheOffset, m_recordSize); return true; } // Load a new cache block m_cacheStart = (index / m_cacheSize) * m_cacheSize; int fileOffset = m_cacheStart * m_recordSize * sizeof(double); // Seek to the start of the cache block FileSeek(m_fileHandle, fileOffset, SEEK_SET); // Read into cache int read = FileReadArray(m_fileHandle, m_cache, 0, m_cacheSize * m_recordSize); if(read > 0) { // Copy from cache int cacheOffset = (index - m_cacheStart) * m_recordSize; ArrayCopy(record, m_cache, 0, cacheOffset, m_recordSize); return true; } return false; } // Get record count int GetRecordCount() { return m_recordCount; } // Destructor ~CDatasetMapper() { if(m_fileHandle != INVALID_HANDLE) { FileClose(m_fileHandle); Print("Dataset mapper closed: ", m_fileName); } } };
The CDatasetMapper class simulates memory mapping by reading and writing fixed-size records to a binary file and storing the most recently accessed items in a small in-memory cache. This design allows you to work with datasets of practically unlimited size, while still keeping performance overhead manageable when reading sequential data or nearby records. Although it’s not true memory mapping at the operating-system level, it delivers many of the same advantages—particularly the ability to process extensive datasets without depleting system memory.
Conclusion
Memory optimization isn’t just about saving a few bytes — it’s about speed, stability, and staying in control. In MQL5, where every millisecond counts, smart memory management becomes a real competitive edge.
In this article, we explored practical strategies that go far beyond theory: understanding MQL5’s internal memory model, reusing objects to cut down on overhead, crafting cache-friendly data structures, and building custom memory pools for high-frequency trading environments.
The golden rule? Don’t guess — measure. Profiling reveals where the real bottlenecks are, allowing you to optimize with precision. Whether it’s pre-allocating memory to avoid runtime latency or simulating memory mapping to work with massive datasets efficiently, every technique we covered serves one purpose: to make your MQL5 applications faster and more resilient.
Apply even a few of these techniques, and you’ll feel the difference. Your systems will be leaner, quicker, and better equipped to handle the demands of modern algorithmic trading.
This isn’t the end — it’s just the starting line. Keep experimenting, keep refining, and take your performance to the next level.
Happy trading! Happy Coding!
File Name | Description |
---|---|
BenchmarkMemoryOperations.mq5 | Code demonstrating how to benchmark and compare memory operations like array allocation, reuse, and string concatenation in MQL5. |
MemoryPoolUsage.mq5 | Example implementation demonstrating how to use custom memory pools for variable-size allocations in MQL5. |
PriceBufferUsage.mq5 | Example script showing practical usage of a circular price buffer for efficient handling of time series data. |
SignalPoolUsage.mq5 | Example illustrating how to utilize an object pool to efficiently manage frequently used trading signal objects. |
CDatasetMapper.mqh | Header file containing the implementation of a simulated memory-mapped file mechanism for handling large datasets. |
CHFTSystem.mqh | Header file defining a class for high-frequency trading systems using pre-allocation strategies to minimize latency. |
CMemoryProfiler.mqh | Header file defining a simple memory profiling class to measure memory usage in MQL5 applications. |
COHLCData.mqh | Header file with a cache-friendly data structure optimized for storing OHLC price data efficiently. |
CPriceBuffer.mqh | Header file containing the circular buffer implementation optimized for rapid price data storage and retrieval. |





- Free trading apps
- Over 8,000 signals for copying
- Economic news for exploring financial markets
You agree to website policy and terms of use
The article looks very debatable (just a couple of points).
What is the class you have mentioned here?
From the presence of OnTick handler and how the array is accessed it's implied that you added the prices array into global scope, which is a bad idea (because of the namespace pollution, if the array is only needed in the handler's scope). Probably it would be more appropriate to keep inital code from the same example, but made the array static, this way everyone clearly see the difference:
Also, if you replace Array of Structures (AoS) with Structure of Arrays (SoA) for OHLCV - the access to the prices of the same bar needs more references (switching between arrays instead of incrementing offset inside a single structure) and slows down the process, but such operations are very common.
For this example with OHLCV, to make it more appropriate for memory and time efficiency, it would be probably more interesting to pack all values into a single 2D or even 1D array:
This is possible because all values of types (double, datetime, long) have the same size 8 byte and can be casted to each other directly.
For this example with OHLCV, to make it more appropriate for memory and time efficiency, it would be probably more interesting to pack all values into a single 2D or even 1D array:
A 2D array instead of an array of structures may slightly save processor time, but it will greatly increase the developer's time spent on developing and maintaining code. In my personal opinion. I agree with the rest of your statements.
https://www.mql5.com/en/articles/17693#sec2
Let's look at a problematic example:
A more efficient approach would be:
The article looks very debatable (just a couple of points).
What is the class you have mentioned here?
From the presence of OnTick handler and how the array is accessed it's implied that you added the prices array into global scope, which is a bad idea (because of the namespace pollution, if the array is only needed in the handler's scope). Probably it would be more appropriate to keep inital code from the same example, but made the array static, this way everyone clearly see the difference:
As far as I understand, that example (I quoted it above) is, roughly speaking, pseudocode. That is, the author does not pay attention to the following (in order to concentrate on what exactly he is talking about, I guess):
In terms of efficiency, I suspect it would be better to replace the entire following loop with a single CopySeries call:
Correct me if I'm wrong, but as far as I remember, every iClose call contains a CopySeries call under the hood.