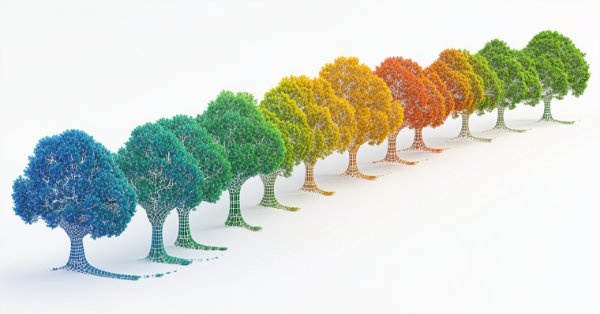
データサイエンスとML(第31回):取引のためのCatBoost AIモデルの使用
「CatBoostは勾配ブースティングライブラリであり、効率的でスケーラブルな方法でカテゴリ特徴量を扱うことで差別化を図り、多くの実世界の問題に対して大幅な性能向上を提供します。」
— Anthony Goldbloom
CatBoostとは?
CatBoostは、決定木に基づく勾配ブースティングアルゴリズムを備えたオープンソースのソフトウェアライブラリであり、機械学習におけるカテゴリ特徴量やデータ処理の課題を解決するために特化して設計されています。
CatBoostはYandexによって開発され、2017年にオープンソースとして公開されました(詳細)。
CatBoostは、線形回帰やSVMなどの従来の機械学習手法と比べて比較的新しい技術であるにもかかわらず、AIコミュニティで急速に注目を集め、Kaggleのようなプラットフォームで最も利用される機械学習モデルの1つとなっています。
CatBoostが特に評価されているのは、多くの機械学習アルゴリズムが苦手とするカテゴリ特徴量の自動処理能力にあります。
- このモデルは、設定やチューニングにほとんど手を加えなくても、他のモデルと比較して優れたパフォーマンスを発揮することが多いです。デフォルトのパラメータを使用した場合でも高い精度を実現できる点が大きな特長です。
- また、ニューラルネットワークのように専門知識や複雑なコーディングが必要とされることがなく、CatBoostは簡単に実装できます。
この記事では、機械学習、決定木、XGBoost、LightGBM、ONNXについての基本的な理解があることを前提としています。
CatBoostの動作方法
CatBoostは、Light Gradient Machine (LightGBM)やExtreme Gradient Boosting (XGBoost)と同様に、勾配ブースティングアルゴリズムに基づいて動作します。このアルゴリズムでは、複数の決定木モデルを順次構築し、各モデルが前のモデルの誤差を補正するように設計されています。各モデルは前のモデルを土台として積み上げる形で学習を進めます。
最終的な予測は、この過程で構築されたすべてのモデルの予測を重み付きで合計することで得られます。
これらの勾配ブースティング決定木(GBDT: Gradient Boosted Decision Tree)の主な目的は、損失関数を最小化することにあります。これは、前のモデルの誤差を修正する新しいモデルを順次追加していくことで実現されます。
カテゴリ特徴量の処理
前述の通り、CatBoostは、ワンホット(One-hot)エンコーディングやラベルエンコーディングのような明示的なエンコーディングを必要とせずに、カテゴリ特徴量を直接扱うことができます。これは、CatBoostがカテゴリ特徴量に対してターゲットベースのエンコーディングを採用しているためです。
このターゲットベースのエンコーディングでは、各カテゴリ特徴値に対するターゲットの条件付き分布を計算してエンコードをおこないます。
さらに、CatBoostが革新的である点の1つに、カテゴリ特徴量の統計量を計算する際に順序付きブースティング(ordered boosting)を用いることが挙げられます。この手法により、各データポイントのエンコーディングが、以前に観測されたデータポイントの情報に基づいて計算されることが保証されます。
これにより、データ漏洩を防ぐと同時に、過剰適合のリスクを低減することが可能になります。
対称決定木構造の採用
LightGBMやXGBoostが非対称な決定木を使用しているのに対し、CatBoostは意思決定プロセスで対称決定木を採用しています。対称木では、各分岐の左枝と右枝が同じ分割ルールに基づいて対称的に構築されるのが特徴です。このアプローチには以下の利点があります。
- 左右対称の分割なので、訓練するのが速い
- より簡単なツリー構造による効率的なメモリ使用
- 対称木はデータの小さな摂動に対してより頑健
CatBoostとXGBoostおよびLightGBMの詳細比較
CatBoostと他の勾配ブースト決定木との違いを理解しましょう。この3つの違いを理解することで、3つのうちのどれを使うべきで、いつ使うべきでないかを知ることができます。
側面 | CatBoost | LightGBM | XGBoost |
---|---|---|---|
カテゴリ特徴量の取り扱い | カテゴリ変数を扱うための自動エンコードと順序付きブースティングを装備 | ワンホットエンコーディング、ラベルエンコーディングなどの手動エンコーディング処理が必要 | ワンホットエンコーディング、ラベルエンコーディングなどの手動エンコーディング処理が必要 |
決定木構造 | 左右対称の決定木があり、バランスよく均等に成長。予測速度が速くなり、過剰適合のリスクも低くなる。 | 最も損失の大きい葉に焦点を当てる葉ごとの成長戦略(非対称)を持つ。その結果、精度は高くなるが、過剰適合のリスクが高くなる。 | 各ノードに最適な分割に基づいてツリーを成長させるレベル単位の成長戦略(非対称)を持つ。このため、予測は柔軟だが遅くなり、過剰適合の危険性がある。 |
モデル精度 | 順序付きブースティングにより、多くのカテゴリ特徴量を含むデータセットを扱う際に優れた精度を提供し、小規模データにおける過剰適合のリスクを低減。 | リーフワイズ成長戦略は、誤差の大きい領域での性能向上に重点を置いているため、特に大規模で高次元のデータセットにおいて優れた精度を提供。 | ほとんどのデータセットで良好な精度を提供するが、カテゴリデータセットではCatBoostに、非常に大規模なデータセットではLightGBMに劣る傾向がある。 |
訓練のスピードと正確さ | 通常、LightGBMよりも訓練に時間がかかるが、小~中程度のデータセットでは、特にカテゴリ特徴量が含まれる場合に効率的。 | 通常、最も高速であり、特に大規模なデータセットでは、高次元データでより効率的なリーフ単位のツリー成長をおこなう。 | ほんのわずかな差で最も遅いことが多い。大規模なデータセットに対して非常に効率的。 |
CatBoostモデルの展開
CatBoostのコードを書く前に、問題のシナリオを作ってみましょう。始値、高値、安値、終値と、日(現在の日付)、曜日(月曜から日曜まで)、年(1から365まで)、月(1月から12月まで)のようないくつかのカテゴリ特徴量を使って、売買シグナル(買い/売り)を予測したいとします。
OHLC(Open、High、Low、Close)値は連続的特徴であり、その他はカテゴリ特徴量です。このデータを収集するスクリプトは添付ファイルにあります。
CatBoostモデルをインストールした後、インポートすることから始めます。
インストール
コマンドライン
pip install catboost
インポートします。
Pythonコード
import numpy as np import pandas as pd import catboost from catboost import CatBoostClassifier from sklearn.pipeline import Pipeline from sklearn.model_selection import train_test_split from sklearn.metrics import classification_report import seaborn as sns import matplotlib.pyplot as plt sns.set_style("darkgrid")
データを視覚化することで、それが何なのかを確認することができます。
df = pd.read_csv("/kaggle/input/ohlc-eurusd/EURUSD.OHLC.PERIOD_D1.csv")
df.head()
結果
Open | High | Low | Close | Day | DayofWeek | DayofYear | Month | |
---|---|---|---|---|---|---|---|---|
0 | 1.09381 | 1.09548 | 1.09003 | 1.09373 | 21.0 | 3.0 | 264.0 | 9.0 |
1 | 1.09678 | 1.09810 | 1.09361 | 1.09399 | 22.0 | 4.0 | 265.0 | 9.0 |
2 | 1.09701 | 1.09973 | 1.09606 | 1.09805 | 23.0 | 5.0 | 266.0 | 9.0 |
3 | 1.09639 | 1.09869 | 1.09542 | 1.09742 | 26.0 | 1.0 | 269.0 | 9.0 |
4 | 1.10302 | 1.10396 | 1.09513 | 1.09757 | 27.0 | 2.0 | 270.0 | 9.0 |
MQL5スクリプト内でデータを収集しているときに、DayofWeek(月曜日から日曜日)とMonth(1月から12月)の値を文字列ではなく整数として取得しました。これは、これらの値を、現状ではカテゴリ変数であるにもかかわらず、文字列を追加できないマトリックスに格納していたためです。これらの値を再びカテゴリに変換し、CatBoostでどのように処理できるかを確認します。
ターゲットとなる変数を用意しましょう。
Pythonコード
new_df = df.copy() # Create a copy of the original DataFrame # we Shift the 'Close' and 'open' columns by one row to ge the future close and open price values, then we add these new columns to the dataset new_df["target_close"] = df["Close"].shift(-1) new_df["target_open"] = df["Open"].shift(-1) new_df = new_df.dropna() # Drop the rows with NaN values resulting from the shift operation open_values = new_df["target_open"] close_values = new_df["target_close"] target = [] for i in range(len(open_values)): if close_values[i] > open_values[i]: target.append(1) # buy signal else: target.append(0) # sell signal new_df["signal"] = target # we create the signal column and add the target variable we just prepared print(new_df.shape) new_df.head()
結果
Open | High | Low | Close | Day | DayofWeek | DayofYear | Month | target_close | target_open | signal | |
---|---|---|---|---|---|---|---|---|---|---|---|
0 | 1.09381 | 1.09548 | 1.09003 | 1.09373 | 21.0 | 3.0 | 264.0 | 9.0 | 1.09399 | 1.09678 | 0 |
1 | 1.09678 | 1.09810 | 1.09361 | 1.09399 | 22.0 | 4.0 | 265.0 | 9.0 | 1.09805 | 1.09701 | 1 |
2 | 1.09701 | 1.09973 | 1.09606 | 1.09805 | 23.0 | 5.0 | 266.0 | 9.0 | 1.09742 | 1.09639 | 1 |
3 | 1.09639 | 1.09869 | 1.09542 | 1.09742 | 26.0 | 1.0 | 269.0 | 9.0 | 1.09757 | 1.10302 | 0 |
4 | 1.10302 | 1.10396 | 1.09513 | 1.09757 | 27.0 | 2.0 | 270.0 | 9.0 | 1.10297 | 1.10431 | 0 |
予測可能なシグナルが得られたので、データを訓練用サンプルとテスト用サンプルに分けましょう。
X = new_df.drop(columns = ["target_close", "target_open", "signal"]) # we drop future values y = new_df["signal"] # trading signals are the target variables we wanna predict X_train, X_test, y_train, y_test = train_test_split(X, y, train_size=0.7, random_state=42)
データセットに存在するカテゴリ特徴量のリストを定義します。
categorical_features = ["Day","DayofWeek", "DayofYear", "Month"]
カテゴリ変数は通常文字列形式なので、このリストを使ってカテゴリ特徴量を文字列形式に変換することができます。
X_train[categorical_features] = X_train[categorical_features].astype(str) X_test[categorical_features] = X_test[categorical_features].astype(str) X_train.info() # we print the data types now
結果
<class 'pandas.core.frame.DataFrame'> Index: 6999 entries, 9068 to 7270 Data columns (total 8 columns): # Column Non-Null Count Dtype --- ------ -------------- ----- 0 Open 6999 non-null float64 1 High 6999 non-null float64 2 Low 6999 non-null float64 3 Close 6999 non-null float64 4 Day 6999 non-null object 5 DayofWeek 6999 non-null object 6 DayofYear 6999 non-null object 7 Month 6999 non-null object dtypes: float64(4), object(4) memory usage: 492.1+ KB
これで、文字列データ型であるオブジェクトデータ型を持つカテゴリ変数ができました。このデータをCatBoost以外の機械学習モデルに適用しようとすると、オブジェクトデータ型は一般的な機械学習の学習データでは使用できないため、エラーが発生します。
CatBoostモデルの訓練
CatBoostモデルの訓練を担当するfitメソッドを呼び出す前に、このモデルを動かす多くのパラメータのいくつかを理解しましょう。
パラメータ | 詳細 |
---|---|
Iterations | 決定木を構築するための反復回数です。 反復回数が多いほど性能は向上しますが、過剰適合のリスクもあります。 |
learning_rate | この係数は、各木の最終予測への配分をコントロールします。 学習率を小さくすると、ツリーが収束するまでの反復回数が増えますが、より優れたモデルになることが多いです。 |
depth | 木の最大深度です。 より深い木は、データのより複雑なパターンを捉えることができますが、しばしば過剰適合につながる可能性があります。 |
cat_features | カテゴリ別インデックスのリストです。 CatBoostモデルはカテゴリ特徴量を検出することができるにもかかわらず、どの特徴量がカテゴリ特徴量であるかを明示的にモデルに指示することは良い習慣です。 カテゴリ変数を自動的に検出する方法が失敗することがあるため、これはモデルが人間の視点からカテゴリ特徴量を理解するのに役立ちます。 |
l2_leaf_reg | L2正則化係数です。 より大きな葉の重みにペナルティを課すことで、過剰適合を防ぐのに役立ちます。 |
border_count | 各カテゴリ特徴量の分割数です。この数値が高いほど性能が良くなり、計算時間が長くなります。 |
eval_metric | 訓練中に使用される評価指標です。 モデルのパフォーマンスを効果的に監視するのに役立ちます。 |
early_stopping_rounds | 検証データがモデルに提供されたとき、このラウンド数でモデルの精度の向上が見られない場合、訓練の進行は停止します。 このパラメータは過剰適合を減らし、学習時間を大幅に短縮するのに役立ちます。 |
上記のパラメータの辞書を定義することができます。
params = dict( iterations=100, learning_rate=0.01, depth=10, l2_leaf_reg=5, bagging_temperature=1, border_count=64, # Number of splits for categorical features eval_metric='Logloss', random_seed=42, # Seed for reproducibility verbose=1, # Verbosity level # early_stopping_rounds=10 # Early stopping for validation )
最後に、Sklearnパイプラインの中でCatboostモデルを定義し、このメソッドに評価データとカテゴリ特徴量のリストを与えて、訓練のためにfitメソッドを呼び出すことができます。
pipe = Pipeline([ ("catboost", CatBoostClassifier(**params)) ]) # Fit the pipeline to the training data pipe.fit(X_train, y_train, catboost__eval_set=(X_test, y_test), catboost__cat_features=categorical_features)
出力
90: learn: 0.6880592 test: 0.6936112 best: 0.6931239 (3) total: 523ms remaining: 51.7ms 91: learn: 0.6880397 test: 0.6936100 best: 0.6931239 (3) total: 529ms remaining: 46ms 92: learn: 0.6880350 test: 0.6936051 best: 0.6931239 (3) total: 532ms remaining: 40ms 93: learn: 0.6880280 test: 0.6936103 best: 0.6931239 (3) total: 535ms remaining: 34.1ms 94: learn: 0.6879448 test: 0.6936110 best: 0.6931239 (3) total: 541ms remaining: 28.5ms 95: learn: 0.6878328 test: 0.6936387 best: 0.6931239 (3) total: 547ms remaining: 22.8ms 96: learn: 0.6877888 test: 0.6936473 best: 0.6931239 (3) total: 553ms remaining: 17.1ms 97: learn: 0.6877408 test: 0.6936508 best: 0.6931239 (3) total: 559ms remaining: 11.4ms 98: learn: 0.6876611 test: 0.6936708 best: 0.6931239 (3) total: 565ms remaining: 5.71ms 99: learn: 0.6876230 test: 0.6936898 best: 0.6931239 (3) total: 571ms remaining: 0us bestTest = 0.6931239281 bestIteration = 3 Shrink model to first 4 iterations.
モデルの評価
このモデルの性能を評価するために、Sklearnメトリクスを使うことができます。
# Make predicitons on training and testing sets y_train_pred = pipe.predict(X_train) y_test_pred = pipe.predict(X_test) # Training set evaluation print("Training Set Classification Report:") print(classification_report(y_train, y_train_pred)) # Testing set evaluation print("\nTesting Set Classification Report:") print(classification_report(y_test, y_test_pred))
出力
Training Set Classification Report: precision recall f1-score support 0 0.55 0.44 0.49 3483 1 0.54 0.64 0.58 3516 accuracy 0.54 6999 macro avg 0.54 0.54 0.54 6999 weighted avg 0.54 0.54 0.54 6999 Testing Set Classification Report: precision recall f1-score support 0 0.53 0.41 0.46 1547 1 0.49 0.61 0.54 1453 accuracy 0.51 3000 macro avg 0.51 0.51 0.50 3000 weighted avg 0.51 0.51 0.50 3000
結果は、平均的なパフォーマンスのモデルです。カテゴリ特徴量リストを削除した後、モデルの精度は訓練セットで60%に上昇したが、テストセットでは変わりませんでした。
pipe.fit(X_train, y_train, catboost__eval_set=(X_test, y_test))
結果
91: learn: 0.6844878 test: 0.6933503 best: 0.6930500 (30) total: 395ms remaining: 34.3ms 92: learn: 0.6844035 test: 0.6933539 best: 0.6930500 (30) total: 399ms remaining: 30ms 93: learn: 0.6843241 test: 0.6933791 best: 0.6930500 (30) total: 404ms remaining: 25.8ms 94: learn: 0.6842277 test: 0.6933732 best: 0.6930500 (30) total: 408ms remaining: 21.5ms 95: learn: 0.6841427 test: 0.6933758 best: 0.6930500 (30) total: 412ms remaining: 17.2ms 96: learn: 0.6840422 test: 0.6933796 best: 0.6930500 (30) total: 416ms remaining: 12.9ms 97: learn: 0.6839896 test: 0.6933825 best: 0.6930500 (30) total: 420ms remaining: 8.58ms 98: learn: 0.6839040 test: 0.6934062 best: 0.6930500 (30) total: 425ms remaining: 4.29ms 99: learn: 0.6838397 test: 0.6934259 best: 0.6930500 (30) total: 429ms remaining: 0us bestTest = 0.6930499562 bestIteration = 30 Shrink model to first 31 iterations.
Training Set Classification Report: precision recall f1-score support 0 0.61 0.53 0.57 3483 1 0.59 0.67 0.63 3516 accuracy 0.60 6999 macro avg 0.60 0.60 0.60 6999 weighted avg 0.60 0.60 0.60 6999
このモデルをさらに詳しく理解するために、特徴量の重要度プロットを作成してみましょう。
# Extract the trained CatBoostClassifier from the pipeline catboost_model = pipe.named_steps['catboost'] # Get feature importances feature_importances = catboost_model.get_feature_importance() feature_im_df = pd.DataFrame({ "feature": X.columns, "importance": feature_importances }) feature_im_df = feature_im_df.sort_values(by="importance", ascending=False) plt.figure(figsize=(10, 6)) sns.barplot(data = feature_im_df, x='importance', y='feature', palette="viridis") plt.title("CatBoost feature importance") plt.xlabel("Importance") plt.ylabel("feature") plt.show()
出力
上記の「特徴量重要度プロット」は、モデルがどのように意思決定をしていたかをすべて物語っています。CatBoostモデルは、連続変数よりもカテゴリ変数を、モデルの最終予測結果に最も影響する特徴として考慮したようです。
CatBoostモデルをONNXに保存する
このモデルをMetaTrader 5で使用するには、ONNX形式に保存する必要があります。CatBoostモデルの保存は、SklearnやKerasのモデルとは異なり、少し厄介です。
ドキュメントの指示に従った後、保存することができました。コードのほとんどは理解しようとしませんでした。
from onnx.helper import get_attribute_value import onnxruntime as rt from skl2onnx import convert_sklearn, update_registered_converter from skl2onnx.common.shape_calculator import ( calculate_linear_classifier_output_shapes, ) # noqa from skl2onnx.common.data_types import ( FloatTensorType, Int64TensorType, guess_tensor_type, ) from skl2onnx._parse import _apply_zipmap, _get_sklearn_operator_name from catboost import CatBoostClassifier from catboost.utils import convert_to_onnx_object def skl2onnx_parser_castboost_classifier(scope, model, inputs, custom_parsers=None): options = scope.get_options(model, dict(zipmap=True)) no_zipmap = isinstance(options["zipmap"], bool) and not options["zipmap"] alias = _get_sklearn_operator_name(type(model)) this_operator = scope.declare_local_operator(alias, model) this_operator.inputs = inputs label_variable = scope.declare_local_variable("label", Int64TensorType()) prob_dtype = guess_tensor_type(inputs[0].type) probability_tensor_variable = scope.declare_local_variable( "probabilities", prob_dtype ) this_operator.outputs.append(label_variable) this_operator.outputs.append(probability_tensor_variable) probability_tensor = this_operator.outputs if no_zipmap: return probability_tensor return _apply_zipmap( options["zipmap"], scope, model, inputs[0].type, probability_tensor ) def skl2onnx_convert_catboost(scope, operator, container): """ CatBoost returns an ONNX graph with a single node. This function adds it to the main graph. """ onx = convert_to_onnx_object(operator.raw_operator) opsets = {d.domain: d.version for d in onx.opset_import} if "" in opsets and opsets[""] >= container.target_opset: raise RuntimeError("CatBoost uses an opset more recent than the target one.") if len(onx.graph.initializer) > 0 or len(onx.graph.sparse_initializer) > 0: raise NotImplementedError( "CatBoost returns a model initializers. This option is not implemented yet." ) if ( len(onx.graph.node) not in (1, 2) or not onx.graph.node[0].op_type.startswith("TreeEnsemble") or (len(onx.graph.node) == 2 and onx.graph.node[1].op_type != "ZipMap") ): types = ", ".join(map(lambda n: n.op_type, onx.graph.node)) raise NotImplementedError( f"CatBoost returns {len(onx.graph.node)} != 1 (types={types}). " f"This option is not implemented yet." ) node = onx.graph.node[0] atts = {} for att in node.attribute: atts[att.name] = get_attribute_value(att) container.add_node( node.op_type, [operator.inputs[0].full_name], [operator.outputs[0].full_name, operator.outputs[1].full_name], op_domain=node.domain, op_version=opsets.get(node.domain, None), **atts, ) update_registered_converter( CatBoostClassifier, "CatBoostCatBoostClassifier", calculate_linear_classifier_output_shapes, skl2onnx_convert_catboost, parser=skl2onnx_parser_castboost_classifier, options={"nocl": [True, False], "zipmap": [True, False, "columns"]}, )
最終的にモデルを変換し拡張子「.onnx」を持つファイルに保存します。
model_onnx = convert_sklearn( pipe, "pipeline_catboost", [("input", FloatTensorType([None, X_train.shape[1]]))], target_opset={"": 12, "ai.onnx.ml": 2}, ) # And save. with open("CatBoost.EURUSD.OHLC.D1.onnx", "wb") as f: f.write(model_onnx.SerializeToString())
Netronで可視化すると、モデル構造はXGBoostやLightGBMと同じに見えます。
これは単なる勾配ブースティング決定木なので、理にかなっています。両者の核心は似たような構造を持っています。
パイプラインのCatBoostモデルをカテゴリ変数付きのONNXに変換しようとすると、エラーが出て処理に失敗しました。
CatBoostError: catboost/libs/model/model_export/model_exporter.cpp:96: ONNX-ML format export does yet not support categorical features
カテゴリ変数が、MetaTrader 5で最初に収集したときと同じようにfloat64 (double)型であることを確認する必要がありました。これによりエラーが修正され、MQL5でこのモデルを操作するときに、double値またはfloat値を整数と混在させることを心配する必要がなくなります。
categorical_features = ["Day","DayofWeek", "DayofYear", "Month"] # Remove these two lines of code operations # X_train[categorical_features] = X_train[categorical_features].astype(str) # X_test[categorical_features] = X_test[categorical_features].astype(str) X_train.info() # we print the data types now
CatBoostモデルは多様な性質のデータセットで動作できるため、この変更にもかかわらず、CatBoostモデルは影響を受けませんでした(同じ精度値を提供した)。
CatBoostエキスパートアドバイザー(取引ロボット)の作成
まず、ONNXモデルをリソースとしてメインのエキスパートアドバイザー(EA)に組み込みます。
MQL5コード
#resource "\\Files\\CatBoost.EURUSD.OHLC.D1.onnx" as uchar catboost_onnx[]
CatBoostモデルをロードするためのライブラリをインポートします。
#include <MALE5\Gradient Boosted Decision Trees(GBDTs)\CatBoost\CatBoost.mqh>
CCatBoost cat_boost;
OnTick関数内で訓練データを収集したのと同じ方法でデータを収集しなければなりません。
void OnTick() { ... ... ... if (CopyRates(Symbol(), timeframe, 1, 1, rates) < 0) //Copy information from the previous bar { printf("Failed to obtain OHLC price values error = ",GetLastError()); return; } MqlDateTime time_struct; string time = (string)datetime(rates[0].time); //converting the date from seconds to datetime then to string TimeToStruct((datetime)StringToTime(time), time_struct); //converting the time in string format to date then assigning it to a structure vector x = {rates[0].open, rates[0].high, rates[0].low, rates[0].close, time_struct.day, time_struct.day_of_week, time_struct.day_of_year, time_struct.mon}; //input features from the previously closed bar ... ... ... }
そして最終的に、予測シグナルと、弱気シグナルクラス0と強気シグナルクラス1の間の確率ベクトルを得ることができます。
vector proba = cat_boost.predict_proba(x); //predict the probability between the classes long signal = cat_boost.predict_bin(x); //predict the trading signal class Comment("Predicted Probability = ", proba,"\nSignal = ",signal);
モデルから得られた予測に基づいて取引戦略をコーディングすることで、このEAをまとめることができます。
戦略は単純で、モデルが強気シグナルを予測したら買い、売りシグナルがあったら決済します。モデルが弱気シグナルを予測した場合は、その逆をおこないます。
void OnTick() { //--- if (!NewBar()) return; //--- Trade at the opening of each bar if (CopyRates(Symbol(), timeframe, 1, 1, rates) < 0) //Copy information from the previous bar { printf("Failed to obtain OHLC price values error = ",GetLastError()); return; } MqlDateTime time_struct; string time = (string)datetime(rates[0].time); //converting the date from seconds to datetime then to string TimeToStruct((datetime)StringToTime(time), time_struct); //converting the time in string format to date then assigning it to a structure vector x = {rates[0].open, rates[0].high, rates[0].low, rates[0].close, time_struct.day, time_struct.day_of_week, time_struct.day_of_year, time_struct.mon}; //input features from the previously closed bar vector proba = cat_boost.predict_proba(x); //predict the probability between the classes long signal = cat_boost.predict_bin(x); //predict the trading signal class Comment("Predicted Probability = ", proba,"\nSignal = ",signal); //--- MqlTick ticks; SymbolInfoTick(Symbol(), ticks); if (signal==1) //if the signal is bullish { if (!PosExists(POSITION_TYPE_BUY)) //There are no buy positions m_trade.Buy(min_lot, Symbol(), ticks.ask, 0, 0); //Open a buy trade ClosePosition(POSITION_TYPE_SELL); //close the opposite trade } else //Bearish signal { if (!PosExists(POSITION_TYPE_SELL)) //There are no Sell positions m_trade.Sell(min_lot, Symbol(), ticks.bid, 0, 0); //open a sell trade ClosePosition(POSITION_TYPE_BUY); //close the opposite trade } }
ストラテジーテスターで2021.01.01から2024.10.8まで4時間足でテストしてみました。モデルは「始値のみ」に設定されました。以下はその結果です。
'
EAは控えめに言ってもうまくいき、55%の利益を上げ、合計96米ドルの純利益をもたらしました。シンプルなデータセット、シンプルなモデル、最小限の取引量の設定にしては悪くありません。
最後に
CatBoostや他の勾配ブースティング決定木アルゴリズムは、限られたリソース環境で作業する際や、多数の機械学習モデルを扱う場面で、複雑な特徴エンジニアリングやモデル構成に時間を費やすことなく「そのまま機能する」モデルを求める場合に最適なソリューションです。
そのシンプルさと低い参入障壁にもかかわらず、CatBoostは多くの実世界のアプリケーションで採用されており、最も優れた、そして効果的なAIモデルの1つとして広く評価されています。
ご精読ありがとうございました。
機械学習モデルの開発を追跡し、本連載で説明されている多くのことは、このGitHubレポに掲載されています。
添付ファイルの表
ファイル名 | ファイルタイプ | 説明と使用法 |
---|---|---|
Experts\CatBoost EA.mq5 | エキスパートアドバイザー(EA) | CatboostモデルをONNX形式で読み込み、MetaTrader 5で取引戦略をテストするための自動売買ロボット |
Include\CatBoost.mqh | インクルードファイル |
|
Files\ CatBoost.EURUSD.OHLC.D1.onnx | ONNXモデル | ONNX形式で保存された学習済みCatBoostモデル |
Scripts\CollectData.mq5 | MQL5スクリプト | 訓練データを収集するスクリプト |
Jupyter Notebook\CatBoost-4-trading.ipynb | Python/Jupyterノートブック | この記事で説明したすべてのPythonコードを含むノートブック |
情報源と参考文献
MetaQuotes Ltdにより英語から翻訳されました。
元の記事: https://www.mql5.com/en/articles/16017





- 無料取引アプリ
- 8千を超えるシグナルをコピー
- 金融ニュースで金融マーケットを探索