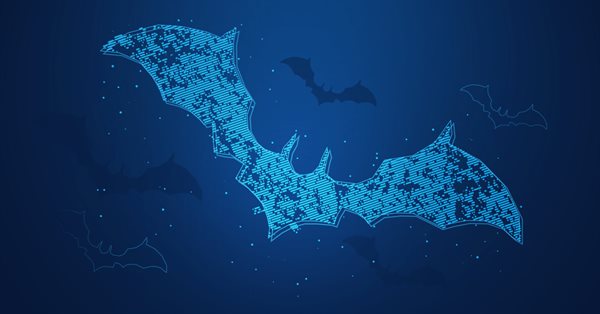
Population optimization algorithms: Bat algorithm (BA)
In this article, I will consider the Bat Algorithm (BA), which shows good convergence on smooth functions.
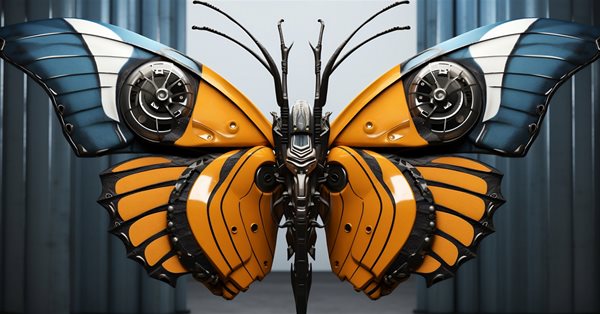
Neural networks made easy (Part 60): Online Decision Transformer (ODT)
The last two articles were devoted to the Decision Transformer method, which models action sequences in the context of an autoregressive model of desired rewards. In this article, we will look at another optimization algorithm for this method.
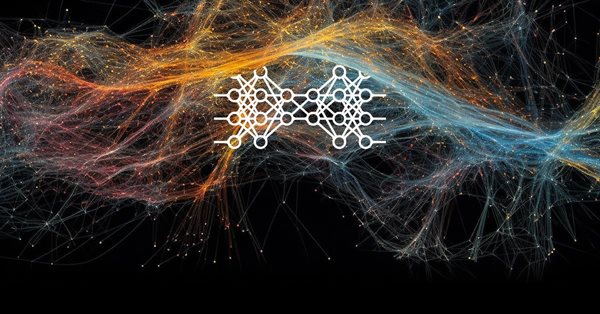
Neural networks made easy (Part 39): Go-Explore, a different approach to exploration
We continue studying the environment in reinforcement learning models. And in this article we will look at another algorithm – Go-Explore, which allows you to effectively explore the environment at the model training stage.
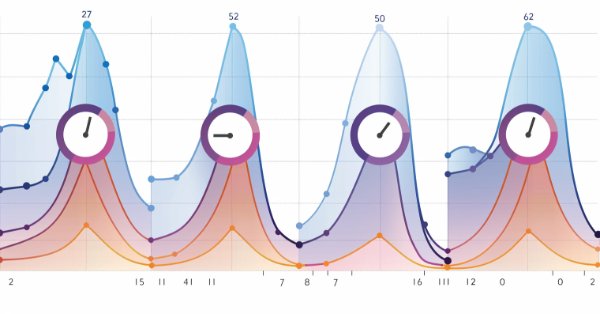
Seasonality Filtering and time period for Deep Learning ONNX models with python for EA
Can we benefit from seasonality when creating models for Deep Learning with python? Does filtering data for the ONNX models help to get better results? What time period should we use? We will cover all of this over this article.
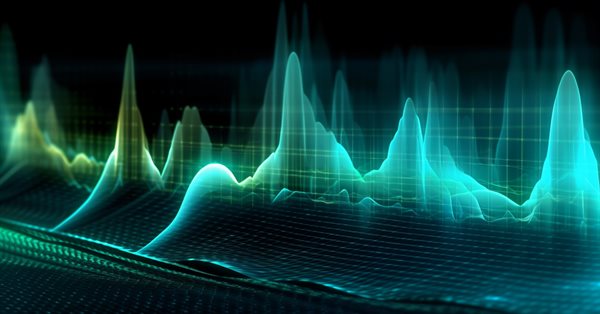
Quantization in machine learning (Part 1): Theory, sample code, analysis of implementation in CatBoost
The article considers the theoretical application of quantization in the construction of tree models and showcases the implemented quantization methods in CatBoost. No complex mathematical equations are used.
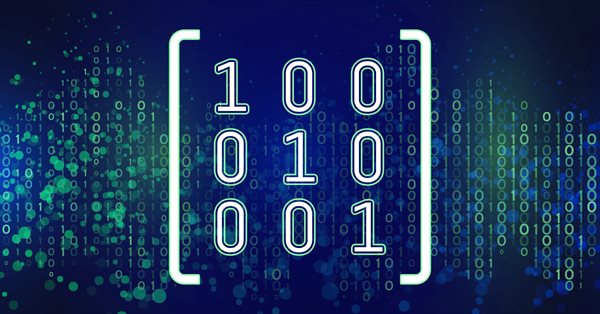
Matrix Utils, Extending the Matrices and Vector Standard Library Functionality
Matrix serves as the foundation of machine learning algorithms and computers in general because of their ability to effectively handle large mathematical operations, The Standard library has everything one needs but let's see how we can extend it by introducing several functions in the utils file, that are not yet available in the library
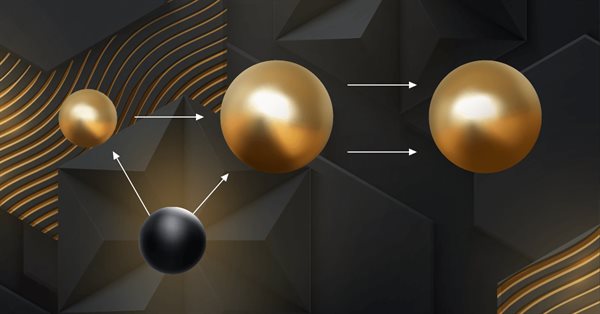
Category Theory in MQL5 (Part 5): Equalizers
Category Theory is a diverse and expanding branch of Mathematics which is only recently getting some coverage in the MQL5 community. These series of articles look to explore and examine some of its concepts & axioms with the overall goal of establishing an open library that provides insight while also hopefully furthering the use of this remarkable field in Traders' strategy development.
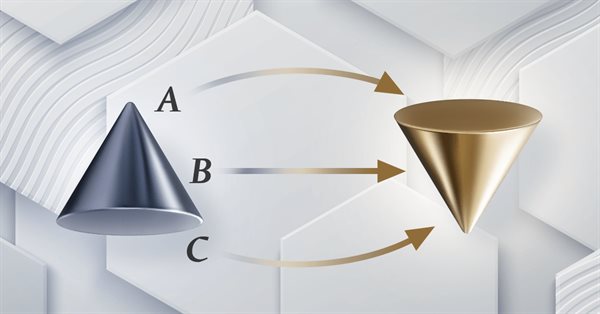
Category Theory in MQL5 (Part 22): A different look at Moving Averages
In this article we attempt to simplify our illustration of concepts covered in these series by dwelling on just one indicator, the most common and probably the easiest to understand. The moving average. In doing so we consider significance and possible applications of vertical natural transformations.
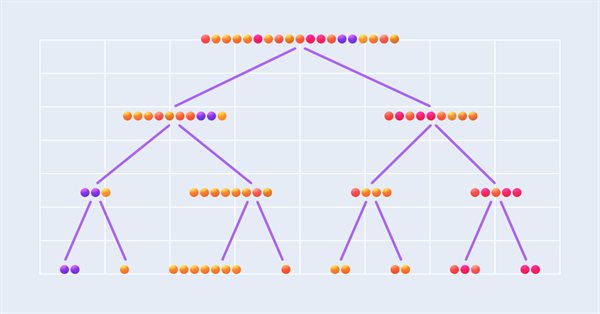
Integrating ML models with the Strategy Tester (Conclusion): Implementing a regression model for price prediction
This article describes the implementation of a regression model based on a decision tree. The model should predict prices of financial assets. We have already prepared the data, trained and evaluated the model, as well as adjusted and optimized it. However, it is important to note that this model is intended for study purposes only and should not be used in real trading.
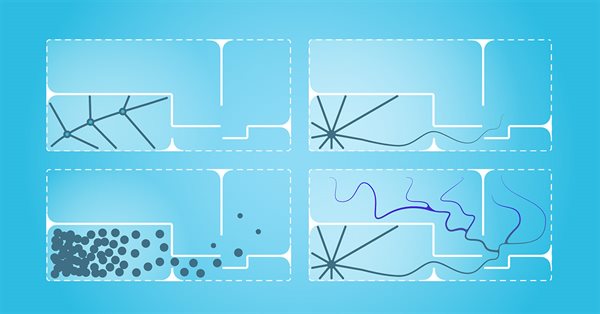
Neural networks made easy (Part 45): Training state exploration skills
Training useful skills without an explicit reward function is one of the main challenges in hierarchical reinforcement learning. Previously, we already got acquainted with two algorithms for solving this problem. But the question of the completeness of environmental research remains open. This article demonstrates a different approach to skill training, the use of which directly depends on the current state of the system.
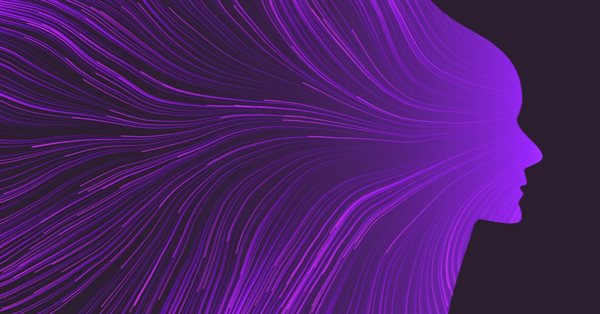
Neural networks made easy (Part 51): Behavior-Guided Actor-Critic (BAC)
The last two articles considered the Soft Actor-Critic algorithm, which incorporates entropy regularization into the reward function. This approach balances environmental exploration and model exploitation, but it is only applicable to stochastic models. The current article proposes an alternative approach that is applicable to both stochastic and deterministic models.
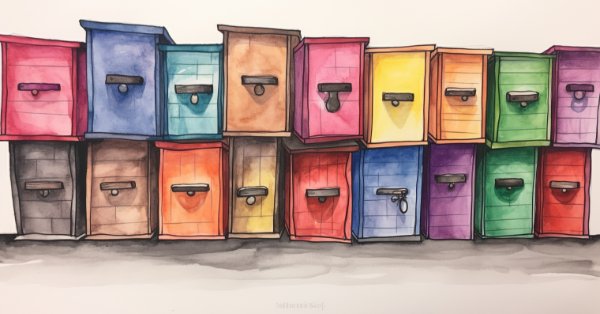
Introduction to MQL5 (Part 3): Mastering the Core Elements of MQL5
Explore the fundamentals of MQL5 programming in this beginner-friendly article, where we demystify arrays, custom functions, preprocessors, and event handling, all explained with clarity making every line of code accessible. Join us in unlocking the power of MQL5 with a unique approach that ensures understanding at every step. This article sets the foundation for mastering MQL5, emphasizing the explanation of each line of code, and providing a distinct and enriching learning experience.
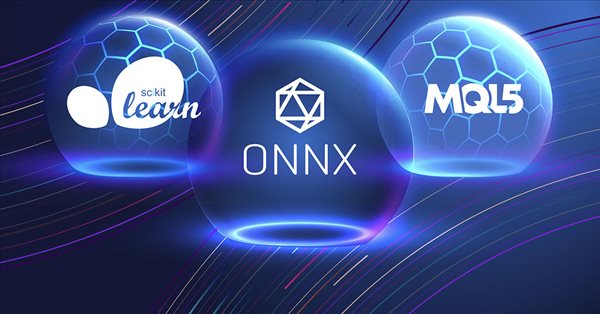
Classification models in the Scikit-Learn library and their export to ONNX
In this article, we will explore the application of all classification models available in the Scikit-Learn library to solve the classification task of Fisher's Iris dataset. We will attempt to convert these models into ONNX format and utilize the resulting models in MQL5 programs. Additionally, we will compare the accuracy of the original models with their ONNX versions on the full Iris dataset.
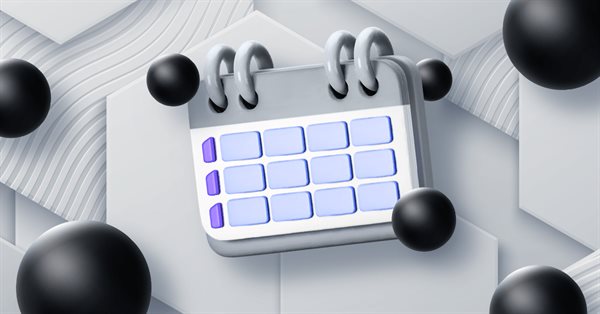
Category Theory in MQL5 (Part 13): Calendar Events with Database Schemas
This article, that follows Category Theory implementation of Orders in MQL5, considers how database schemas can be incorporated for classification in MQL5. We take an introductory look at how database schema concepts could be married with category theory when identifying trade relevant text(string) information. Calendar events are the focus.
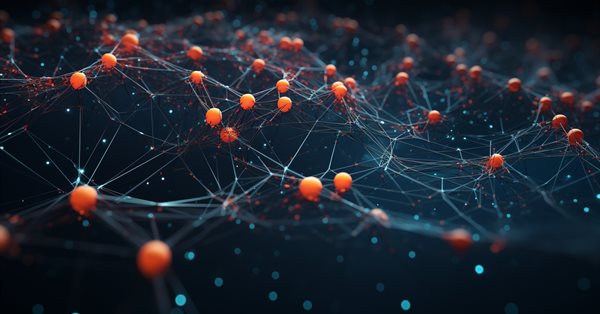
MQL5 Wizard Techniques you should know (Part 08): Perceptrons
Perceptrons, single hidden layer networks, can be a good segue for anyone familiar with basic automated trading and is looking to dip into neural networks. We take a step by step look at how this could be realized in a signal class assembly that is part of the MQL5 Wizard classes for expert advisors.
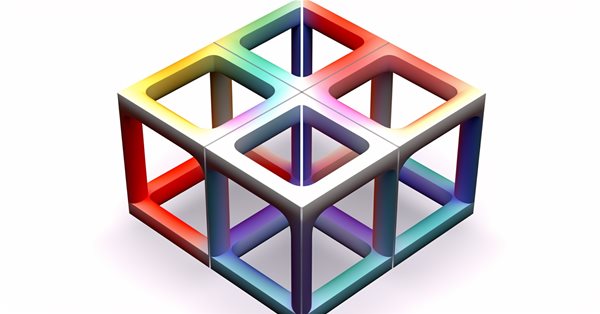
Population optimization algorithms: Nelder–Mead, or simplex search (NM) method
The article presents a complete exploration of the Nelder-Mead method, explaining how the simplex (function parameter space) is modified and rearranged at each iteration to achieve an optimal solution, and describes how the method can be improved.
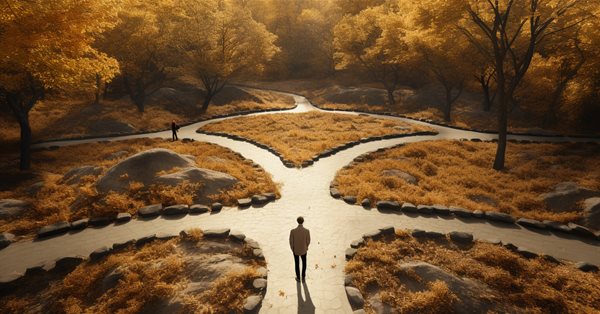
Neural networks made easy (Part 68): Offline Preference-guided Policy Optimization
Since the first articles devoted to reinforcement learning, we have in one way or another touched upon 2 problems: exploring the environment and determining the reward function. Recent articles have been devoted to the problem of exploration in offline learning. In this article, I would like to introduce you to an algorithm whose authors completely eliminated the reward function.
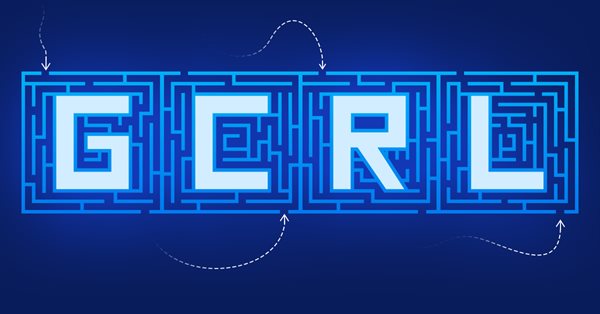
Neural networks made easy (Part 46): Goal-conditioned reinforcement learning (GCRL)
In this article, we will have a look at yet another reinforcement learning approach. It is called goal-conditioned reinforcement learning (GCRL). In this approach, an agent is trained to achieve different goals in specific scenarios.
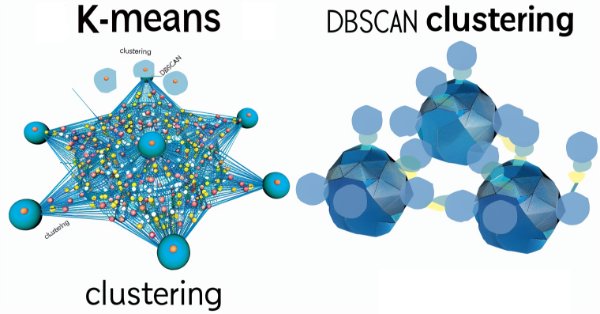
MQL5 Wizard Techniques you should know (Part 13): DBSCAN for Expert Signal Class
Density Based Spatial Clustering for Applications with Noise is an unsupervised form of grouping data that hardly requires any input parameters, save for just 2, which when compared to other approaches like k-means, is a boon. We delve into how this could be constructive for testing and eventually trading with Wizard assembled Expert Advisers
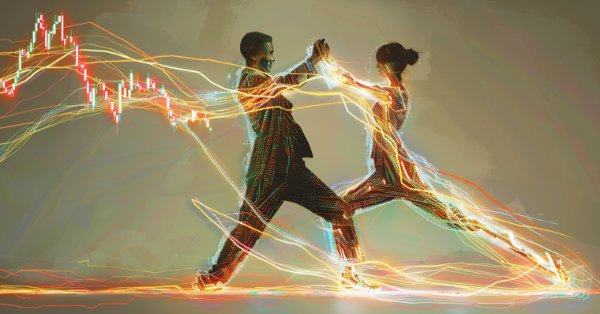
Statistical Arbitrage with predictions
We will walk around statistical arbitrage, we will search with python for correlation and cointegration symbols, we will make an indicator for Pearson's coefficient and we will make an EA for trading statistical arbitrage with predictions done with python and ONNX models.
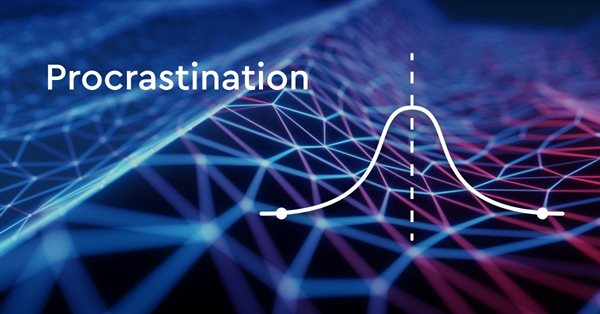
Neural networks made easy (Part 42): Model procrastination, reasons and solutions
In the context of reinforcement learning, model procrastination can be caused by several reasons. The article considers some of the possible causes of model procrastination and methods for overcoming them.
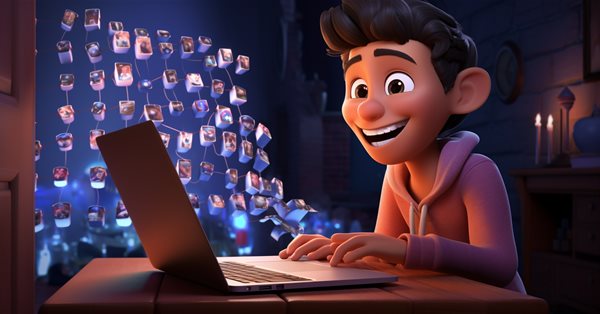
Introduction to MQL5 (Part 2): Navigating Predefined Variables, Common Functions, and Control Flow Statements
Embark on an illuminating journey with Part Two of our MQL5 series. These articles are not just tutorials, they're doorways to an enchanted realm where programming novices and wizards alike unite. What makes this journey truly magical? Part Two of our MQL5 series stands out with its refreshing simplicity, making complex concepts accessible to all. Engage with us interactively as we answer your questions, ensuring an enriching and personalized learning experience. Let's build a community where understanding MQL5 is an adventure for everyone. Welcome to the enchantment!
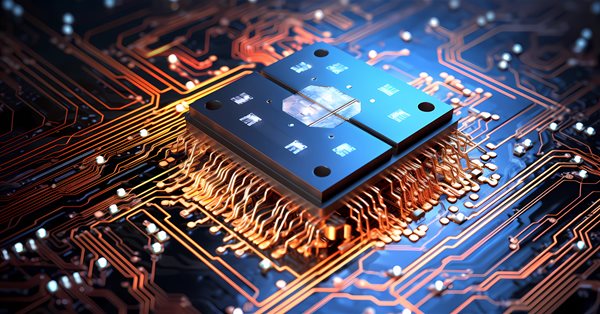
Integrate Your Own LLM into EA (Part 1): Hardware and Environment Deployment
With the rapid development of artificial intelligence today, language models (LLMs) are an important part of artificial intelligence, so we should think about how to integrate powerful LLMs into our algorithmic trading. For most people, it is difficult to fine-tune these powerful models according to their needs, deploy them locally, and then apply them to algorithmic trading. This series of articles will take a step-by-step approach to achieve this goal.
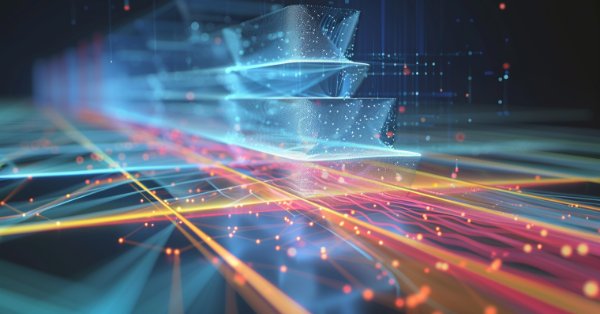
Data Science and Machine Learning (Part 20): Algorithmic Trading Insights, A Faceoff Between LDA and PCA in MQL5
Uncover the secrets behind these powerful dimensionality reduction techniques as we dissect their applications within the MQL5 trading environment. Delve into the nuances of Linear Discriminant Analysis (LDA) and Principal Component Analysis (PCA), gaining a profound understanding of their impact on strategy development and market analysis.
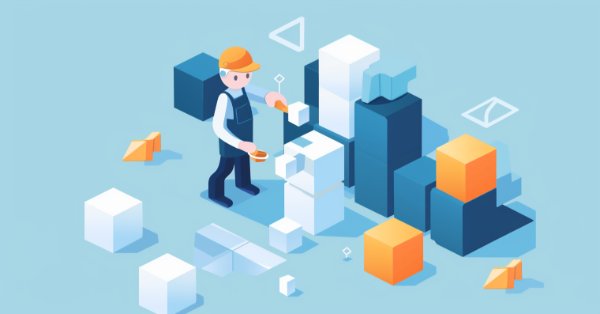
Introduction to MQL5 (Part 6): A Beginner's Guide to Array Functions in MQL5
Embark on the next phase of our MQL5 journey. In this insightful and beginner-friendly article, we'll look into the remaining array functions, demystifying complex concepts to empower you to craft efficient trading strategies. We’ll be discussing ArrayPrint, ArrayInsert, ArraySize, ArrayRange, ArrarRemove, ArraySwap, ArrayReverse, and ArraySort. Elevate your algorithmic trading expertise with these essential array functions. Join us on the path to MQL5 mastery!
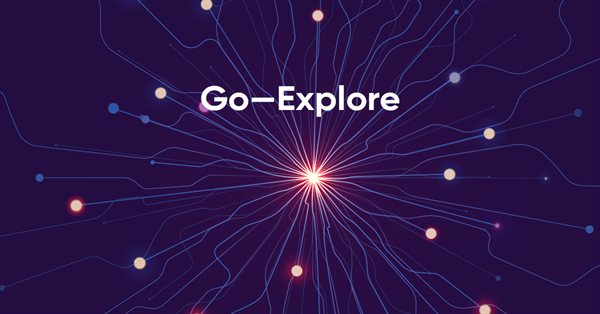
Neural networks made easy (Part 40): Using Go-Explore on large amounts of data
This article discusses the use of the Go-Explore algorithm over a long training period, since the random action selection strategy may not lead to a profitable pass as training time increases.
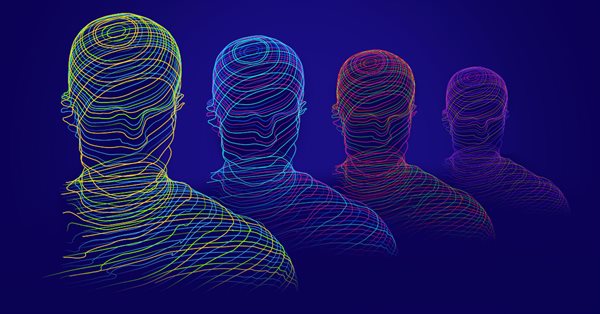
Population optimization algorithms: Mind Evolutionary Computation (MEC) algorithm
The article considers the algorithm of the MEC family called the simple mind evolutionary computation algorithm (Simple MEC, SMEC). The algorithm is distinguished by the beauty of its idea and ease of implementation.
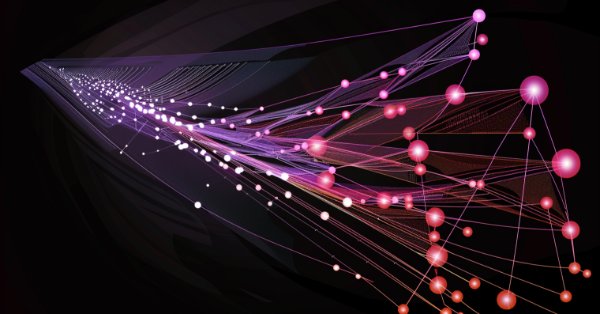
MQL5 Wizard Techniques you should know (Part 16): Principal Component Analysis with Eigen Vectors
Principal Component Analysis, a dimensionality reducing technique in data analysis, is looked at in this article, with how it could be implemented with Eigen values and vectors. As always, we aim to develop a prototype expert-signal-class usable in the MQL5 wizard.
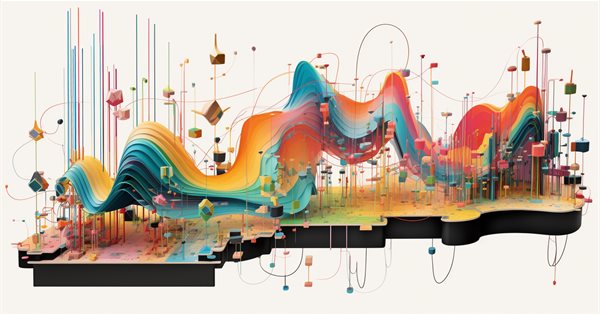
Data label for time series mining (Part 4):Interpretability Decomposition Using Label Data
This series of articles introduces several time series labeling methods, which can create data that meets most artificial intelligence models, and targeted data labeling according to needs can make the trained artificial intelligence model more in line with the expected design, improve the accuracy of our model, and even help the model make a qualitative leap!
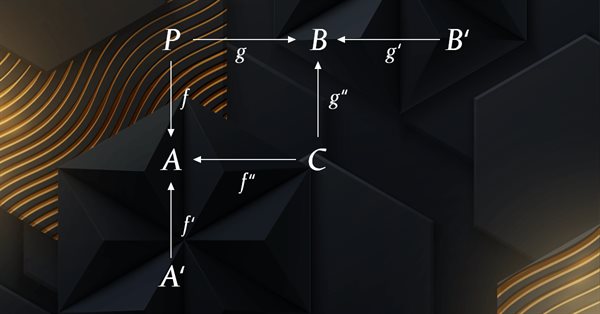
Category Theory in MQL5 (Part 4): Spans, Experiments, and Compositions
Category Theory is a diverse and expanding branch of Mathematics which as of yet is relatively uncovered in the MQL5 community. These series of articles look to introduce and examine some of its concepts with the overall goal of establishing an open library that provides insight while hopefully furthering the use of this remarkable field in Traders' strategy development.
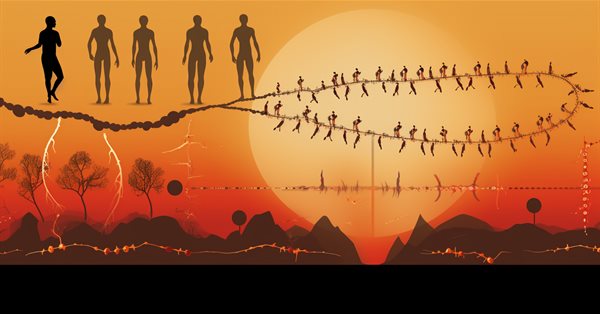
Population optimization algorithms: Differential Evolution (DE)
In this article, we will consider the algorithm that demonstrates the most controversial results of all those discussed previously - the differential evolution (DE) algorithm.
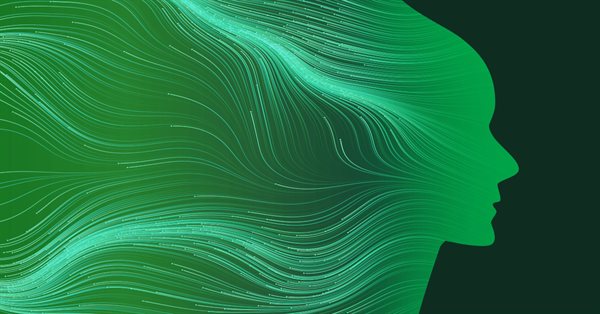
Neural networks made easy (Part 52): Research with optimism and distribution correction
As the model is trained based on the experience reproduction buffer, the current Actor policy moves further and further away from the stored examples, which reduces the efficiency of training the model as a whole. In this article, we will look at the algorithm of improving the efficiency of using samples in reinforcement learning algorithms.
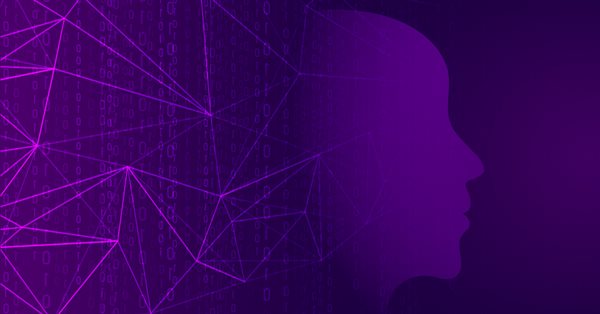
Neural networks made easy (Part 57): Stochastic Marginal Actor-Critic (SMAC)
Here I will consider the fairly new Stochastic Marginal Actor-Critic (SMAC) algorithm, which allows building latent variable policies within the framework of entropy maximization.
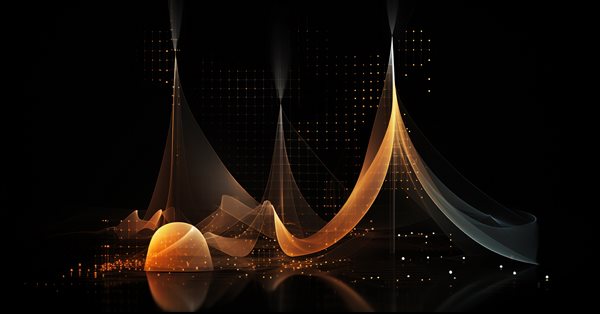
Category Theory in MQL5 (Part 23): A different look at the Double Exponential Moving Average
In this article we continue with our theme in the last of tackling everyday trading indicators viewed in a ‘new’ light. We are handling horizontal composition of natural transformations for this piece and the best indicator for this, that expands on what we just covered, is the double exponential moving average (DEMA).
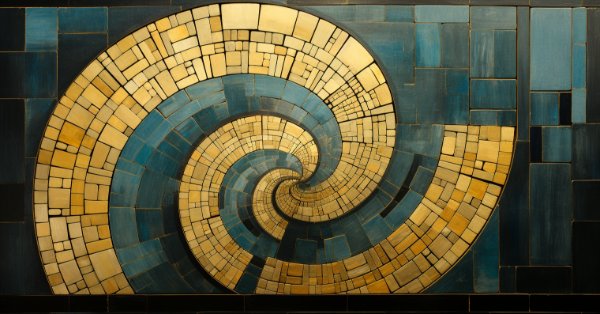
MQL5 Wizard Techniques you should know (Part 11): Number Walls
Number Walls are a variant of Linear Shift Back Registers that prescreen sequences for predictability by checking for convergence. We look at how these ideas could be of use in MQL5.
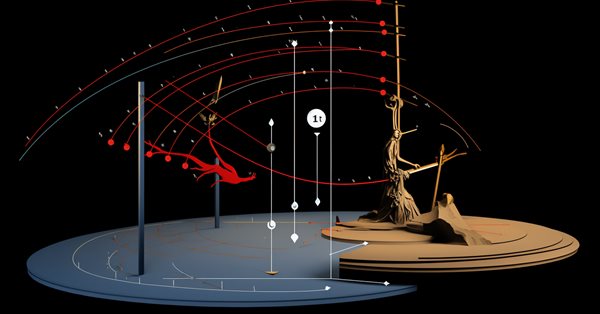
Neural networks made easy (Part 64): ConserWeightive Behavioral Cloning (CWBC) method
As a result of tests performed in previous articles, we came to the conclusion that the optimality of the trained strategy largely depends on the training set used. In this article, we will get acquainted with a fairly simple yet effective method for selecting trajectories to train models.
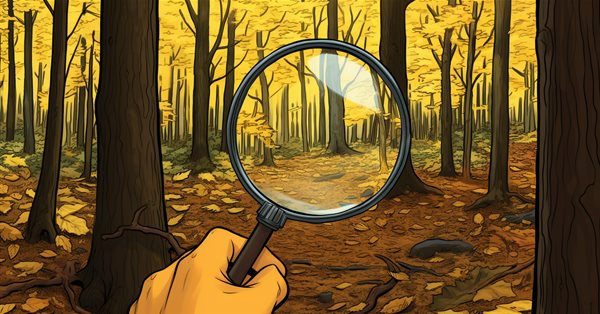
Data Science and Machine Learning (Part 17): Money in the Trees? The Art and Science of Random Forests in Forex Trading
Discover the secrets of algorithmic alchemy as we guide you through the blend of artistry and precision in decoding financial landscapes. Unearth how Random Forests transform data into predictive prowess, offering a unique perspective on navigating the complex terrain of stock markets. Join us on this journey into the heart of financial wizardry, where we demystify the role of Random Forests in shaping market destiny and unlocking the doors to lucrative opportunities
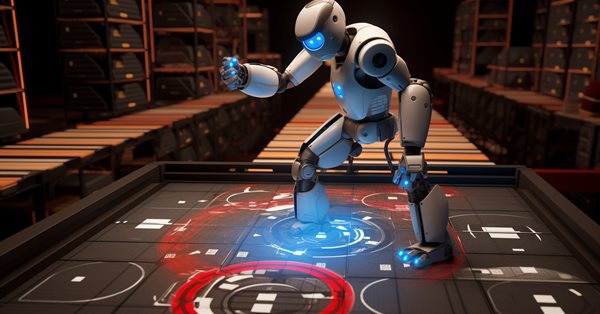
Neural networks made easy (Part 62): Using Decision Transformer in hierarchical models
In recent articles, we have seen several options for using the Decision Transformer method. The method allows analyzing not only the current state, but also the trajectory of previous states and actions performed in them. In this article, we will focus on using this method in hierarchical models.
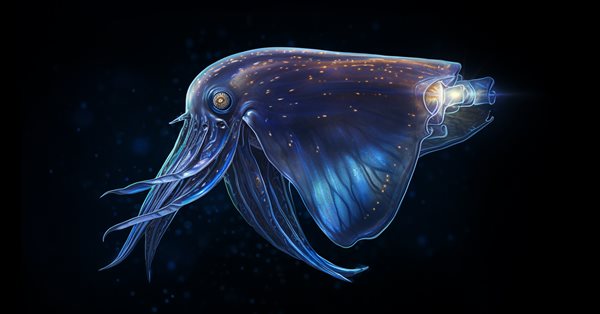
Population optimization algorithms: Changing shape, shifting probability distributions and testing on Smart Cephalopod (SC)
The article examines the impact of changing the shape of probability distributions on the performance of optimization algorithms. We will conduct experiments using the Smart Cephalopod (SC) test algorithm to evaluate the efficiency of various probability distributions in the context of optimization problems.
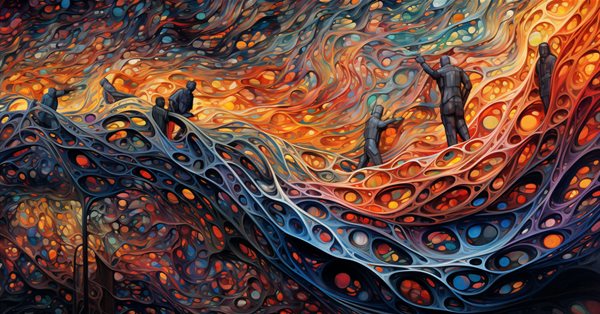
Neural networks made easy (Part 65): Distance Weighted Supervised Learning (DWSL)
In this article, we will get acquainted with an interesting algorithm that is built at the intersection of supervised and reinforcement learning methods.