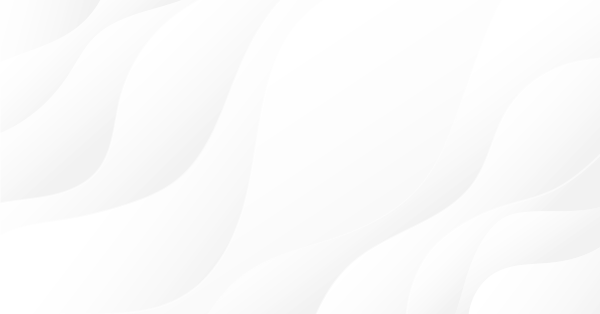
FANN2MQL Tutorial zu neuralem Netzwerk
Als erstes:
Installieren Sie bitte die fann2MQL Bibliothek. Sie ist erforderlich, um dieses Beispiel zu testen. Sie kann hier heruntergeladen werden.
Einleitung
Bisher gab es nur ein Beispiel dafür, wie man die Fann2MQL Bibliothek verwendet, wodurch Händlern ermöglicht wird, die Open-Source Bibliothek "FANN" für neurale Netzwerke in ihren MQL-Codes zu verwenden.
Aber das Beispiel, das vom Schöpfer der Fann2MQL Bibliothek geschrieben wurde, ist nicht leicht zu verstehen. Es ist nicht für Anfänger gemacht.
Daher habe ich ein weiteres Beispiel geschrieben, das in seinem Konzept einfacher und vollständig kommentiert ist.
Es hängt nicht direkt mit dem Handel zusammen und verwendet auch keine Finanzdaten. Es ist ein einfaches statisches Anwendungsbeispiel.
In diesem Beispiel möchten wir einem neuralen Netzwerk beibringen, ein einfaches Muster zu erkennen:
Das Muster, das wir lehren möchten, setzt sich aus 3 Zahlen zusammen: a, b and c.
if a < b && b < c dann ist die erwartete Ausgabe = 1
if a < b && b > c dann ist die erwartete Ausgabe = 0
if a > b && b > c dann ist die erwartete Ausgabe = 0
if a > b && b < c dann ist die erwartete Ausgabe = 1
Sie können diese Zahlen zum Beispiel als Vektorkoordinaten (Vektor geht nach oben "UP" oder unten "DOWN") oder als Marktrichtung sehen. In diesem Fall könnte das Muster folgendermaßen interpretiert werden:
UP UP = UP
UP DOWN = DOWN
DOWN DOWN = DOWN
DOWN UP = UP
Als erstes werden wir ein neurales Netzwerk erstellen.
Danach zeigen wir dem Netzwerk einige Beispiele von Mustern, so dass es die Regeln lernen und ableiten kann.
Abschließend werden wir dem Netzwerk neue Muster zeigen, die es noch nie zuvor gesehen hat und es fragen, was seine Schlussfolgerungen daraus sind. Wenn es die Regeln verstanden hat, dann wird es auch in der Lage sein, diese Muster zu erkennen.
der kommentierte Code:
// We include the Fann2MQl library #include <Fann2MQL.mqh> #property copyright "Copyright © 2009, Julien Loutre" #property link "http://www.thetradingtheory.com" #property indicator_separate_window #property indicator_buffers 0 // the total number of layers. here, there is one input layer, // 2 hidden layers, and one output layer = 4 layers. int nn_layer = 4; int nn_input = 3; // Number of input neurones. Our pattern is made of 3 numbers, // so that means 3 input neurones. int nn_hidden1 = 8; // Number of neurones on the first hidden layer int nn_hidden2 = 5; // number on the second hidden layer int nn_output = 1; // number of outputs // trainingData[][] will contain the examples // we're gonna use to teach the rules to the neurones. double trainingData[][4]; // IMPORTANT! size = nn_input + nn_output int maxTraining = 500; // maximum number of time we will train // the neurones with some examples double targetMSE = 0.002; // the Mean-Square Error of the neurones we should // get at most (you will understand this lower in the code) int ann; // This var will be the identifier of the neuronal network. // When the indicator is removed, we delete all of the neurnal networks // from the memory of the computer. int deinit() { f2M_destroy_all_anns(); return(0); } int init() { int i; double MSE; Print("=================================== START EXECUTION ================================"); IndicatorBuffers(0); IndicatorDigits(6); // We resize the trainingData array, so we can use it. // We're gonna change its size one size at a time. ArrayResize(trainingData,1); Print("##### INIT #####"); // We create a new neuronal networks ann = f2M_create_standard(nn_layer, nn_input, nn_hidden1, nn_hidden2, nn_output); // we check if it was created successfully. 0 = OK, -1 = error debug("f2M_create_standard()",ann); // We set the activation function. Don't worry about that. Just do it. f2M_set_act_function_hidden (ann, FANN_SIGMOID_SYMMETRIC_STEPWISE); f2M_set_act_function_output (ann, FANN_SIGMOID_SYMMETRIC_STEPWISE); // Some studies show that statistically, the best results are reached using this range; // but you can try to change and see is it gets better or worst f2M_randomize_weights (ann, -0.77, 0.77); // I just print to the console the number of input and output neurones. // Just to check. Just for debug purpose. debug("f2M_get_num_input(ann)",f2M_get_num_input(ann)); debug("f2M_get_num_output(ann)",f2M_get_num_output(ann)); Print("##### REGISTER DATA #####"); // Now we prepare some data examples (with expected output) // and we add them to the training set. // Once we have add all the examples we want, we're gonna send // this training data set to the neurones, so they can learn. // prepareData() has a few arguments: // - Action to do (train or compute) // - the data (here, 3 data per set) // - the last argument is the expected output. // Here, this function takes the example data and the expected output, // and add them to the learning set. // Check the comment associated with this function to get more details. // // here is the pattern we're going to teach: // There is 3 numbers. Let's call them a, b and c. // You can think of those numbers as being vector coordinates // for example (vector going up or down) // if a < b && b < c then output = 1 // if a < b && b > c then output = 0 // if a > b && b > c then output = 0 // if a > b && b < c then output = 1 // UP UP = UP / if a < b && b < c then output = 1 prepareData("train",1,2,3,1); prepareData("train",8,12,20,1); prepareData("train",4,6,8,1); prepareData("train",0,5,11,1); // UP DOWN = DOWN / if a < b && b > c then output = 0 prepareData("train",1,2,1,0); prepareData("train",8,10,7,0); prepareData("train",7,10,7,0); prepareData("train",2,3,1,0); // DOWN DOWN = DOWN / if a > b && b > c then output = 0 prepareData("train",8,7,6,0); prepareData("train",20,10,1,0); prepareData("train",3,2,1,0); prepareData("train",9,4,3,0); prepareData("train",7,6,5,0); // DOWN UP = UP / if a > b && b < c then output = 1 prepareData("train",5,4,5,1); prepareData("train",2,1,6,1); prepareData("train",20,12,18,1); prepareData("train",8,2,10,1); // Now we print the full training set to the console, to check how it looks like. // this is just for debug purpose. printDataArray(); Print("##### TRAINING #####"); // We need to train the neurones many time in order // for them to be good at what we ask them to do. // Here I will train them with the same data (our examples) over and over again, // until they fully understand the rules we are trying to teach them, or until // the training has been repeated 'maxTraining' number of time // (in this case maxTraining = 500) // The better they understand the rule, the lower their mean-Square Error will be. // the teach() function returns this mean-Square Error (or MSE) // 0.1 or lower is a sufficient number for simple rules // 0.02 or lower is better for complex rules like the one // we are trying to teach them (it's a patttern recognition. not so easy.) for (i=0;i<maxTraining;i++) { MSE = teach(); // everytime the loop run, the teach() function is activated. // Check the comments associated to this function to understand more. if (MSE < targetMSE) { // if the MSE is lower than what we defined (here targetMSE = 0.02) debug("training finished. Trainings ",i+1); // then we print in the console // how many training // it took them to understand i = maxTraining; // and we go out of this loop } } // we print to the console the MSE value once the training is completed debug("MSE",f2M_get_MSE(ann)); Print("##### RUNNING #####"); // And now we can ask the neurone to analyse new data that they never saw. // Will they recognize the patterns correctly? // You can see that I used the same prepareData() function here, // with the first argument set to "compute". // The last argument which was dedicated to the expected output // when we used this function for registering examples earlier, // is now useless, so we leave it to zero. // if you prefer, you can call directly the compute() function. // In this case, the structure is compute(inputVector[]); // So instead of prepareData("compute",1,3,1,0); you would do something like: // double inputVector[]; // declare a new array // ArrayResize(inputVector,f2M_get_num_input(ann)); // resize the array to the number of neuronal input // inputVector[0] = 1; // add in the array the data // inputVector[1] = 3; // inputVector[2] = 1; // result = compute(inputVector); // call the compute() function, with the input array. // the prepareData() function call the compute() function, // which print the result to the console, // so we can check if the neurones were right or not. debug("1,3,1 = UP DOWN = DOWN. Should output 0.",""); prepareData("compute",1,3,1,0); debug("1,2,3 = UP UP = UP. Should output 1.",""); prepareData("compute",1,2,3,0); debug("3,2,1 = DOWN DOWN = DOWN. Should output 0.",""); prepareData("compute",3,2,1,0); debug("45,2,89 = DOWN UP = UP. Should output 1.",""); prepareData("compute",45,2,89,0); debug("1,3,23 = UP UP = UP. Should output 1.",""); prepareData("compute",1,3,23,0); debug("7,5,6 = DOWN UP = UP. Should output 1.",""); prepareData("compute",7,5,6,0); debug("2,8,9 = UP UP = UP. Should output 1.",""); prepareData("compute",2,8,9,0); Print("=================================== END EXECUTION ================================"); return(0); } int start() { return(0); } /************************* ** printDataArray() ** Print the datas used for training the neurones ** This is useless. Just created for debug purpose. *************************/ void printDataArray() { int i,j; int bufferSize = ArraySize(trainingData)/(f2M_get_num_input(ann)+f2M_get_num_output(ann))-1; string lineBuffer = ""; for (i=0;i<bufferSize;i++) { for (j=0;j<(f2M_get_num_input(ann)+f2M_get_num_output(ann));j++) { lineBuffer = StringConcatenate(lineBuffer, trainingData[i][j], ","); } debug("DataArray["+i+"]", lineBuffer); lineBuffer = ""; } } /************************* ** prepareData() ** Prepare the data for either training or computing. ** It takes the data, put them in an array, ** and send them to the training or running function ** Update according to the number of input/output your code needs. *************************/ void prepareData(string action, double a, double b, double c, double output) { double inputVector[]; double outputVector[]; // we resize the arrays to the right size ArrayResize(inputVector,f2M_get_num_input(ann)); ArrayResize(outputVector,f2M_get_num_output(ann)); inputVector[0] = a; inputVector[1] = b; inputVector[2] = c; outputVector[0] = output; if (action == "train") { addTrainingData(inputVector,outputVector); } if (action == "compute") { compute(inputVector); } // if you have more input than 3, just change the structure of this function. } /************************* ** addTrainingData() ** Add a single set of training data **(data example + expected output) to the global training set *************************/ void addTrainingData(double inputArray[], double outputArray[]) { int j; int bufferSize = ArraySize(trainingData)/(f2M_get_num_input(ann)+f2M_get_num_output(ann))-1; //register the input data to the main array for (j=0;j<f2M_get_num_input(ann);j++) { trainingData[bufferSize][j] = inputArray[j]; } for (j=0;j<f2M_get_num_output(ann);j++) { trainingData[bufferSize][f2M_get_num_input(ann)+j] = outputArray[j]; } ArrayResize(trainingData,bufferSize+2); } /************************* ** teach() ** Get all the trainign data and use them to train the neurones one time. ** In order to properly train the neurones, you need to run , ** this function many time until the Mean-Square Error get low enough. *************************/ double teach() { int i,j; double MSE; double inputVector[]; double outputVector[]; ArrayResize(inputVector,f2M_get_num_input(ann)); ArrayResize(outputVector,f2M_get_num_output(ann)); int call; int bufferSize = ArraySize(trainingData)/(f2M_get_num_input(ann)+f2M_get_num_output(ann))-1; for (i=0;i<bufferSize;i++) { for (j=0;j<f2M_get_num_input(ann);j++) { inputVector[j] = trainingData[i][j]; } outputVector[0] = trainingData[i][3]; //f2M_train() is showing the neurones only one example at a time. call = f2M_train(ann, inputVector, outputVector); } // Once we have show them an example, // we check if how good they are by checking their MSE. // If it's low, they learn good! MSE = f2M_get_MSE(ann); return(MSE); } /************************* ** compute() ** Compute a set of data and returns the computed result *************************/ double compute(double inputVector[]) { int j; int out; double output; ArrayResize(inputVector,f2M_get_num_input(ann)); // We sent new data to the neurones. out = f2M_run(ann, inputVector); // and check what they say about it using f2M_get_output(). output = f2M_get_output(ann, 0); debug("Computing()",MathRound(output)); return(output); } /************************* ** debug() ** Print data to the console *************************/ void debug(string a, string b) { Print(a+" ==> "+b); }
Die Ausgabe
Ausgabe des neuralen Netzwerks in der Konsole.
Fazit
Sie können auch den Artikel "Verwenden von neuralen Netzwerken in MetaTrader" lesen, der von Mariusz Woloszyn, dem Autor der Fann2MQL Bibliothek geschrieben wurde.
Ich habe 4 Tage benötigt um zu verstehen, wie man Fann in MetaTrader verwendet, indem ich die kleine Dokumentation analysiert habe, die hier und auf Google verfügbar ist.
Ich hoffe, dass dieses Beispiel nützlich für Sie sein wird und Sie daher nicht zu viel Zeit durch Experimentieren verlieren. Weitere Artikel werden in den nächsten Wochen folgen.
Sollten Sie Fragen haben, teilen Sie sie mir mit und ich werde sie beantworten.
Übersetzt aus dem Englischen von MetaQuotes Ltd.
Originalartikel: https://www.mql5.com/en/articles/1574





- Freie Handelsapplikationen
- Über 8.000 Signale zum Kopieren
- Wirtschaftsnachrichten für die Lage an den Finanzmärkte
Sie stimmen der Website-Richtlinie und den Nutzungsbedingungen zu.