Discussing the article: "Utilizing CatBoost Machine Learning model as a Filter for Trend-Following Strategies"
Check out the new article: Utilizing CatBoost Machine Learning model as a Filter for Trend-Following Strategies.
Author: Zhuo Kai Chen
From my own experience, I can only add that training models on data older than three months brings nothing to the equation. The model should be capable of capturing current patterns and focusing on them. You need to stay close to the market.
From my own experience, I can only add that training models on data older than three months brings nothing to the equation. The model should be capable of capturing current patterns and focusing on them. You need to stay close to the market.
Your point is absolutely valid. In my experience, I also find that using recent data makes it easier to train a model that produces better results. However, the main issue is that this approach limits the size of the training set unless you use a very short timeframe. Trend-following strategies perform better over longer timeframes compared to shorter ones according to academic papers. So you might train a model with higher predictability but fewer training samples, which is prone to overfitting, and the original strategy has less edge to begin with. There is a tradeoff between these factors, not to mention that spreads also play a role.
My solution to this is to assign greater weights to recent data in the training set while still preserving older data. In this way, we can adapt to new patterns after a regime shift while still benefiting from old patterns that have stayed consistent over the years. However, as I mentioned earlier, there are always trade-offs involved in these decisions.
Your point is absolutely valid. In my experience, I also find that using recent data makes it easier to train a model that produces better results. However, the main issue is that this approach limits the size of the training set unless you use a very short timeframe. Trend-following strategies perform better over longer timeframes compared to shorter ones according to academic papers. So you might train a model with higher predictability but fewer training samples, which is prone to overfitting, and the original strategy has less edge to begin with. There is a tradeoff between these factors, not to mention that spreads also play a role.
My solution to this is to assign greater weights to recent data in the training set while still preserving older data. In this way, we can adapt to new patterns after a regime shift while still benefiting from old patterns that have stayed consistent over the years. However, as I mentioned earlier, there are always trade-offs involved in these decisions.
Retraining the model every three months using the latest three months of data would probably do the trick. I’ll try to implement this rolling model optimization idea later. Thanks for the suggestion.
I am trying to follow your instructions and sadly due to a lack of detail am not able to continue through to the final output due to missing file references and no download links
I will take the time to teach you what you have missed because this looks like a good process.
You are missing;
1. A direct link to FileCSV.mqh which requires going through the other article to obtain it.
2. declaration of all of the features handles
3. Adequate explanation of the process for either creating or downloading the files
CatOnnx.mqh
"\\Files\\CatBoost_Momentum_test.onnx"
4. Direct links to and relevant instructions on how to install catboot using pip or similar making sure you have the dependencies installed that are required for python. (not for me but others will need to know)
5. Instruction to read the CB2.ipynb instructions and workflow.
Overall this all leads to the student getting half way through your article and being left searching for hours for the correct process to complete the tutorial and replicate your results.
Overall I give this article a 4 out of 10 for information with additional points for your Classic Trend Following Strategy which is well put together.
Please edit the article to be more instructive and step by step so we can all learn and follow.
PS
recommendations on how this could be adapted to other strategies would be great!
I am trying to follow your instructions and sadly due to a lack of detail am not able to continue through to the final output due to missing file references and no download links
I will take the time to teach you what you have missed because this looks like a good process.
You are missing;
1. A direct link to FileCSV.mqh which requires going through the other article to obtain it.
2. declaration of all of the features handles
3. Adequate explanation of the process for either creating or downloading the files
CatOnnx.mqh
"\\Files\\CatBoost_Momentum_test.onnx"
4. Direct links to and relevant instructions on how to install catboot using pip or similar making sure you have the dependencies installed that are required for python. (not for me but others will need to know)
5. Instruction to read the CB2.ipynb instructions and workflow.
Overall this all leads to the student getting half way through your article and being left searching for hours for the correct process to complete the tutorial and replicate your results.
Overall I give this article a 4 out of 10 for information with additional points for your Classic Trend Following Strategy which is well put together.
Please edit the article to be more instructive and step by step so we can all learn and follow.
PS
recommendations on how this could be adapted to other strategies would be great!
Thanks for the feedback. Unfortunately I ended up only briefly describing the relevant articles because I thought it would take up too much space, and I didn't include download links due to copyright issues. The thorough details would still be best obtained from the original source. Nevertheless, I do think I overlooked some careful instructions for my python code and direct links to python instructions although I added comments for each line. If you have specific obstacles in your own implementation process, you can discuss here or add me to chat.
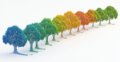
- www.mql5.com
The CatOnnx.mqh called in the ML-Momentum.mq5 file is the same as the CatBoost.mqh as I cited in this article. Sorry for causing confusions in the file names.
Thanks great to get some clarification this looks very interesting, when I go back and try again to complete the guide in this article I will keep some notes aside for later forum fertilization.
If anyone else is interested in cat farming...

- Free trading apps
- Over 8,000 signals for copying
- Economic news for exploring financial markets
You agree to website policy and terms of use
Check out the new article: Utilizing CatBoost Machine Learning model as a Filter for Trend-Following Strategies.
CatBoost is a powerful tree-based machine learning model that specializes in decision-making based on stationary features. Other tree-based models like XGBoost and Random Forest share similar traits in terms of their robustness, ability to handle complex patterns, and interpretability. These models have a wide range of uses, from feature analysis to risk management. In this article, we're going to walk through the procedure of utilizing a trained CatBoost model as a filter for a classic moving average cross trend-following strategy.
This article is meant to provide insights into the strategy development process while addressing the challenges one may face along the way. I will introduce my workflow of fetching data from MetaTrader 5, training machine learning model in Python, and integrating back to MetaTrader 5 Expert Advisors. By the end of this article, we will validate the strategy through statistical testing and discuss future aspirations extending from the current approach.
The rule of thumb in the industry for developing CTA (Commodity Trading Advisor) strategy is that it's best to have a clear, intuitive explanation behind every strategy idea. This is basically how people think of strategy ideas in the first place, not to mention it avoids overfitting as well. This suggestion is subservient even working with machine learning models. We'll try to explain the intuition behind this idea.
Why this could work:
CatBoost model creates decision trees that take in the feature inputs and output the probability of each outcome. In this case, we're only training on binary outcomes (1 is win,0 is loss). The model will alter rules in the decision trees so that it minimizes the loss function in the training data set. If the model displays a certain level of predictability on the out-of-sample testing outcome, we may consider using it to filter out trades that have little probability of winning, which could in turn boost the overall profitability.
A realistic expectation for retail traders like you and I is that the models we train will not be like oracles, but rather only slightly better than random walk. There are plenty of ways to improve the model precision, which I will discuss later, but nevertheless it's a great endeavor for slight improvement.
Author: Zhuo Kai Chen