Gamuchirai Zororo Ndawana / Profile
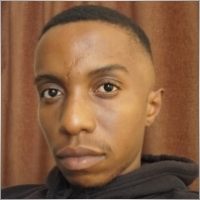
- Information
1 year
experience
|
6
products
|
15
demo versions
|
0
jobs
|
1
signals
|
0
subscribers
|
If you want to discover how you can get better results, faster, then you're in the right place.
You can get started with any of my free expert advisors, or you can read some of my publications if you thirst for knowledge.
What are you waiting for? A lifetime partnership towards your success starts here.
Consultation Calls: 1 hour call $30
Project Development: Rates Will Be Negotiated And Settled Over A Consultation Call
Algorithmic Account Management: $70 deposit + 25% commission of profits earned.
1 on 1 Tutorials: Rates Will be Negotiated And Settled Over A Consultation Call.
Email: gamuchiraindawa@gmail.com

High probability Setups are well known in our trading community, but regrettably they are not well-defined. In this article, we will aim to find an empirical and algorithmic way of defining exactly what is a high probability setup, identifying and exploiting them. By using Gradient Boosting Trees, we demonstrated how the reader can improve the performance of an arbitrary trading strategy and better communicate the exact job to be done to our computer in a more meaningful and explicit manner.

Dimension reduction techniques are widely used to improve the performance of machine learning models. Let us discuss a relatively new technique known as Uniform Manifold Approximation and Projection (UMAP). This new technique has been developed to explicitly overcome the limitations of legacy methods that create artifacts and distortions in the data. UMAP is a powerful dimension reduction technique, and it helps us group similar candle sticks in a novel and effective way that reduces our error rates on out of sample data and improves our trading performance.

This article explores optimizing RSI levels and periods for better trading signals. We introduce methods to estimate optimal RSI values and automate period selection using grid search and statistical models. Finally, we implement the solution in MQL5 while leveraging Python for analysis. Our approach aims to be pragmatic and straightforward to help you solve potentially complicated problems, with simplicity.

Traders often face drawdowns from false signals, while waiting for confirmation can lead to missed opportunities. This article introduces a triangular trading strategy using Silver’s pricing in Dollars (XAGUSD) and Euros (XAGEUR), along with the EURUSD exchange rate, to filter out noise. By leveraging cross-market relationships, traders can uncover hidden sentiment and refine their entries in real time.

Join us in our discussion today as we look for an algorithmic procedure to minimize the total number of times we get stopped out of winning trades. The problem we faced is significantly challenging, and most solutions given in community discussions lack set and fixed rules. Our algorithmic approach to solving the problem increased the profitability of our trades and reduced our average loss per trade. However, there are further advancements to be made to completely filter out all trades that will be stopped out, our solution is a good first step for anyone to try.

In this article, we take our second attempt to convert the changes in price levels on any market, into a corresponding change in angle. This time around, we selected a more mathematically sophisticated approach than we selected in our first attempt, and the results we obtained suggest that our change in approach may have been the right decision. Join us today, as we discuss how we can use Polar coordinates to calculate the angle formed by changes in price levels, in a meaningful way, regardless of which market you are analyzing.

The best practices, defining how to safely us an indicator, are not always easy to follow. Quiet market conditions may surprisingly produce readings on the indicator that do not qualify as a trading signal, leading to missed opportunities for algorithmic traders. This article will suggest a potential solution to this problem, as we discuss how to build trading applications capable of adapting their trading rules to the available market data.

Successfully employing algorithmic trading requires continuous, interdisciplinary learning. However, the infinite range of possibilities can consume years of effort without yielding tangible results. To address this, we propose a framework that gradually introduces complexity, allowing traders to refine their strategies iteratively rather than committing indefinite time to uncertain outcomes.

Financial markets are typically classified as either in a range mode or a trending mode. This static view of the market may make it easier for us to trade in the short run. However, it is disconnected from the reality of the market. In this article, we look to better understand how exactly financial markets move between these 2 possible modes and how we can use our new understanding of market behavior to gain confidence in our algorithmic trading strategies.

Moving average cross-overs are widely known by traders in our community, and yet the core of the strategy has changed very little since its inception. In this discussion, we will present you with a slight adjustment to the original strategy, that aims to minimize the lag present in the trading strategy. All fans of the original strategy, could consider revising the strategy in accordance with the insights we will discuss today. By using 2 moving averages with the same period, we reduce the lag in the trading strategy considerably, without violating the foundational principles of the strategy.
Introducing the Volatility Doctor Professional Edition Built on Trust, Refined for Excellence When we launched our original Expert Advisor in September 2023, we set out with one goal: to provide a reliable, easy-to-use trading tool that delivered real value. Since then, thanks to the feedback of hundreds of traders from all over the world, our free version has achieved over 500 downloads and earned a 4.5-star community rating. We’ve listened. We’ve refined. And now, we’re proud to introduce the

I want to improve my consistency. I'm skipping days inbetween and I don't like that. Anyway, OOP in MQL5 is better than OOP in Java in my opinion, this chapter was fun. Not all of it made sense, I guess I haven't faced enough problems on my journey to understand why some features in MQL5 are important, but still fun. Page 202 💯
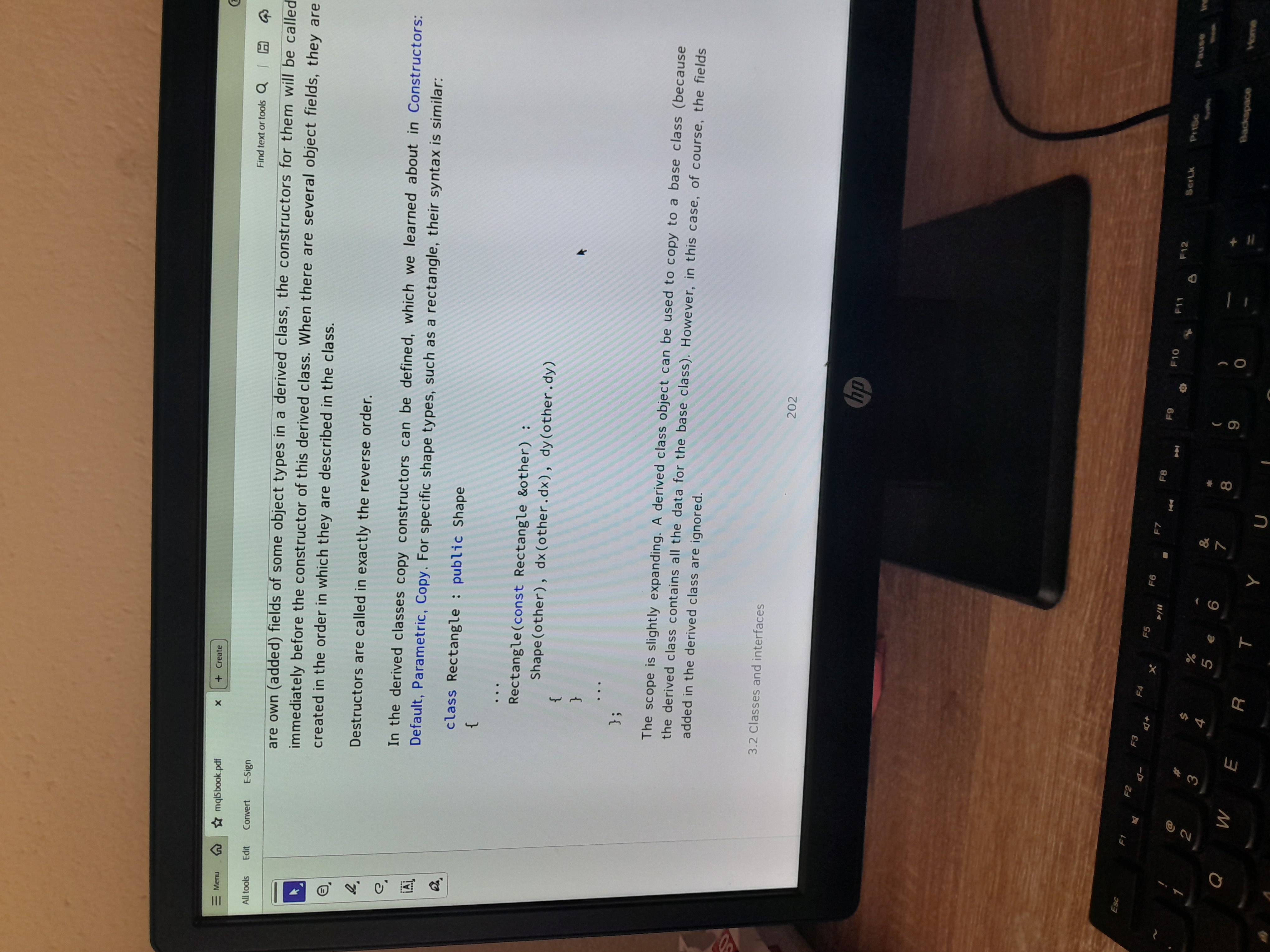

Join us today as we challenge ourselves to build a trading strategy around the USDJPY pair. We will trade candlestick patterns that are formed on the daily time frame because they potentially have more strength behind them. Our initial strategy was profitable, which encouraged us to continue refining the strategy and adding extra layers of safety, to protect the capital gained.

Join us today as we challenge ourselves to build a profitable break-out trading strategy in MQL5. We selected the EURUSD pair and attempted to trade price breakouts on the hourly timeframe. Our system had difficulty distinguishing between false breakouts and the beginning of true trends. We layered our system with filters intended to minimize our losses whilst increasing our gains. In the end, we successfully made our system profitable and less prone to false breakouts.

The moving averages and the stochastic oscillator could be used to generate trend following trading signals. However, these signals will only be observed after the price action has occurred. We can effectively overcome this inherent lag in technical indicators using AI. This article will teach you how to create a fully autonomous AI-powered Expert Advisor in a manner that can improve any of your existing trading strategies. Even the oldest trading strategy possible can be improved.

There are many posts in the MQL5 Forum asking for help calculating the slope of price changes. This article will demonstrate one possible way of calculating the angle formed by the changes in price in any market you wish to trade. Additionally, we will answer if engineering this new feature is worth the extra effort and time invested. We will explore if the slope of the price can improve any of our AI model's accuracy when forecasting the USDZAR pair on the M1.

Managing trading account risk is a challenge for all traders. How can we develop trading applications that dynamically learn high, medium, and low-risk modes for various symbols in MetaTrader 5? By using PCA, we gain better control over portfolio variance. I’ll demonstrate how to create applications that learn these three risk modes from market data fetched from MetaTrader 5.

Traditional machine learning teaches practitioners to be vigilant not to overfit their models. However, this ideology is being challenged by new insights published by diligent researches from Harvard, who have discovered that what appears to be overfitting may in some circumstances be the results of terminating your training procedures prematurely. We will demonstrate how we can use the ideas published in the research paper, to improve our use of AI in forecasting market returns.