Andrey Dik / Profil
- Bilgiler
11+ yıl
deneyim
|
4
ürünler
|
107
demo sürümleri
|
15
işler
|
0
sinyaller
|
0
aboneler
|
A group for communication on optimization and free product testing://t.me/+vazsAAcney4zYmZi
Attention! My Telegram doppelgangers have appeared, my real nickname is @JQS_aka_Joo
My github with optimization algorithms: https://github.com/JQSakaJoo/Population-optimization-algorithms-MQL5
All my publications: https://www.mql5.com/en/users/joo/publications
I have been developing systems based on machine learning technologies since 2007 and in the field of artificial
intelligence, optimization and forecasting.
I took an active part in the development of the MT5 platform, such as the introduction of support for universal parallel
computing on the GPU and CPU with OpenCL, testing and backtesting of distributed
computing in the LAN and cloud during optimization in MT5, my test functions are included in the standard delivery of the terminal.
⭐⭐ ⭐ ⭐ ⭐ ⭐ ⭐ ⭐ ⭐⭐ ⭐ ⭐ ⭐ ⭐ ⭐ ⭐ ⭐ ⭐ ⭐ ⭐ ⭐ ⭐ ⭐ ⭐ ⭐ ⭐ ⭐ ⭐ ⭐ ⭐ ⭐ ⭐ ⭐ ⭐ ⭐ ⭐
My Products:
https://www.mql5.com/en/users/joo/seller
Recommended Brokers:
https://rbfxdirect.com/ru/lk/?a=dnhp

Here we will consider the evolution of the ACS algorithm: three modifications aimed at improving the convergence characteristics and the algorithm efficiency. Transformation of one of the leading optimization algorithms. From matrix modifications to revolutionary approaches regarding population formation.

Artificial Cooperative Search (ACS) is an innovative method using a binary matrix and multiple dynamic populations based on mutualistic relationships and cooperation to find optimal solutions quickly and accurately. ACS unique approach to predators and prey enables it to achieve excellent results in numerical optimization problems.

In this article, we will rethink code locks, transforming them from security mechanisms into tools for solving complex optimization problems. Discover the world of code locks viewed not as simple security devices, but as inspiration for a new approach to optimization. We will create a whole population of "locks", where each lock represents a unique solution to the problem. We will then develop an algorithm that will "pick" these locks and find optimal solutions in a variety of areas, from machine learning to trading systems development.

In this article, we will look at the Comet Tail Optimization Algorithm (CTA), which draws inspiration from unique space objects - comets and their impressive tails that form when approaching the Sun. The algorithm is based on the concept of the motion of comets and their tails, and is designed to find optimal solutions in optimization problems.

This is a unique optimization algorithm inspired by the evolution of the turtle shell. The TSEA algorithm emulates the gradual formation of keratinized skin areas, which represent optimal solutions to a problem. The best solutions become "harder" and are located closer to the outer surface, while the less successful solutions remain "softer" and are located inside. The algorithm uses clustering of solutions by quality and distance, allowing to preserve less successful options and providing flexibility and adaptability.

In the second part of the article, we will move on to the practical implementation of the BSO algorithm, conduct tests on test functions and compare the efficiency of BSO with other optimization methods.

In this article, we will look at an innovative optimization method called BSO (Brain Storm Optimization) inspired by a natural phenomenon called "brainstorming". We will also discuss a new approach to solving multimodal optimization problems the BSO method applies. It allows finding multiple optimal solutions without the need to pre-determine the number of subpopulations. We will also consider the K-Means and K-Means++ clustering methods.

Мой github с алгоритмами оптимизации: https://github.com/JQSakaJoo/Population-optimization-algorithms-MQL5

The article considers Boids algorithm based on unique examples of animal flocking behavior. In turn, the Boids algorithm serves as the basis for the creation of the whole class of algorithms united under the name "Swarm Intelligence".

The article explores the bird swarm-based algorithm (BSA) inspired by the collective flocking interactions of birds in nature. The different search strategies of individuals in BSA, including switching between flight, vigilance and foraging behavior, make this algorithm multifaceted. It uses the principles of bird flocking, communication, adaptability, leading and following to efficiently find optimal solutions.

In this article, we will look at the Mersenne Twister random number generator and compare it with the standard one in MQL5. We will also find out the influence of the random number generator quality on the results of optimization algorithms.

Whale Optimization Algorithm (WOA) is a metaheuristic algorithm inspired by the behavior and hunting strategies of humpback whales. The main idea of WOA is to mimic the so-called "bubble-net" feeding method, in which whales create bubbles around prey and then attack it in a spiral motion.

Here we will dive into the world of hybridization of optimization algorithms by looking at three key types: strategy mixing, sequential and parallel hybridization. We will conduct a series of experiments combining and testing relevant optimization algorithms.

We continue our experiment that aims to examine the behavior of population optimization algorithms in the context of their ability to efficiently escape local minima when population diversity is low and reach global maxima. Research results are provided.
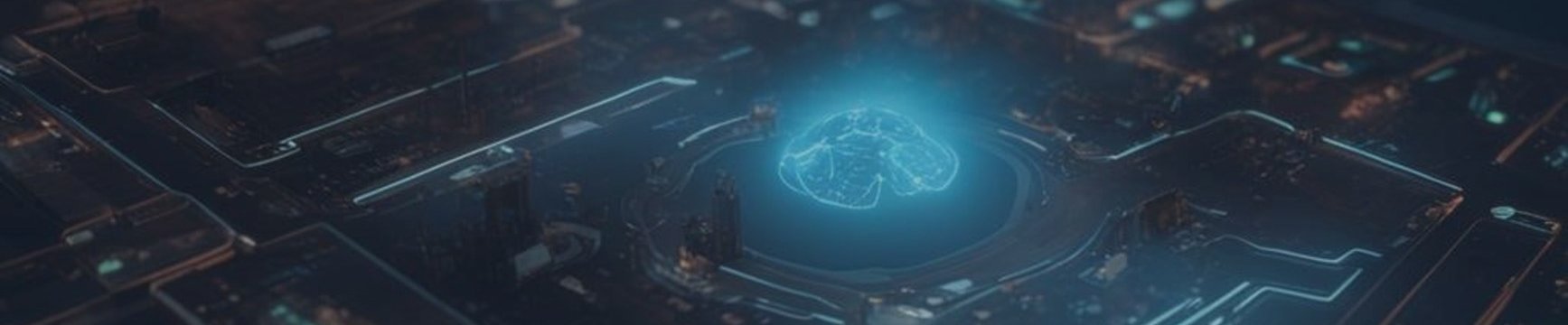
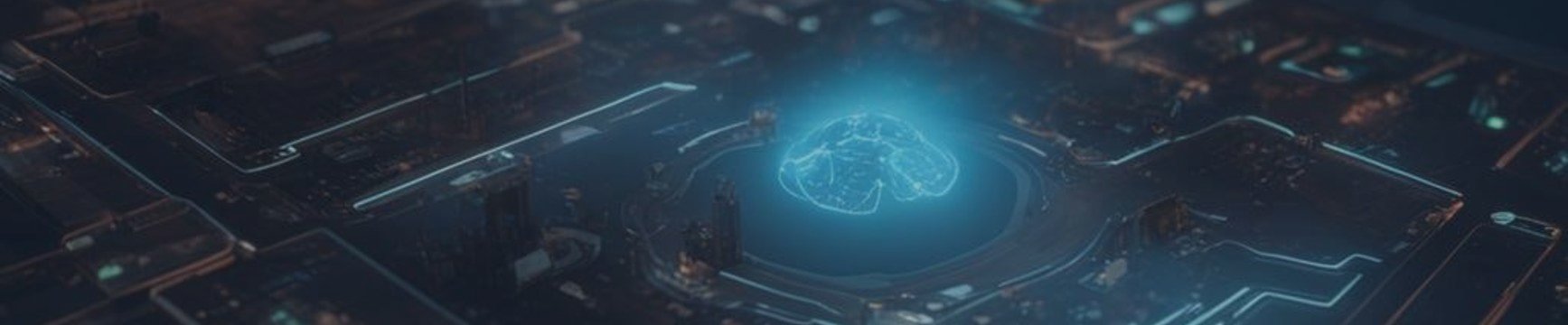

This article presents a unique experiment that aims to examine the behavior of population optimization algorithms in the context of their ability to efficiently escape local minima when population diversity is low and reach global maxima. Working in this direction will provide further insight into which specific algorithms can successfully continue their search using coordinates set by the user as a starting point, and what factors influence their success.

The article represents a unique research attempt to combine a variety of population algorithms into a single class to simplify the application of optimization methods. This approach not only opens up opportunities for the development of new algorithms, including hybrid variants, but also creates a universal basic test stand. This stand becomes a key tool for choosing the optimal algorithm depending on a specific task.

The article discusses the practical aspects of using optimization algorithms to find the best EA parameters on the fly, as well as virtualization of trading operations and EA logic. The article can be used as an instruction for implementing optimization algorithms into an EA.

This is a continuation of the previous article considering the idea of social groups. The article explores the evolution of social groups using movement and memory algorithms. The results will help to understand the evolution of social systems and apply them in optimization and search for solutions.