
You are missing trading opportunities:
- Free trading apps
- Over 8,000 signals for copying
- Economic news for exploring financial markets
Registration
Log in
You agree to website policy and terms of use
If you do not have an account, please register
I think that if the number of degrees of freedom is easy and the type of dependence is a priori clear - what is the question?
Z.I. I missed Desert Island. I'd like to read something that makes sense...
I don't know, but this island seems to be floating in the noosphere...
And the population is deluded in its immortality.
Imho.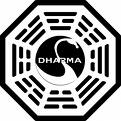
Can you be more specific?
The MOC is positioned, among other things, as a method for estimating the best parameters for a function selected a priori by the researcher.
Formulas for calculating these parameters minimizing the square of the deviation of actual data from the proxying function are derived for a variety of functions.
Where do the fat tails appear?
Please enlighten me...
Fat tails may occur if the model chosen by the researcher is not adequate to the real series. The standard check of adequacy of any regression model to the real data is the residuals distribution. Residuals are the deviation of real data from the model data. If the model is adequate, then the distribution of the residuals shall be normal. The ANC minimizes the sum of these deviations, but the distribution of the residuals will not necessarily be normal, although in some parts of the series it may be. Linear regression makes sense for SB model with drift or at some parts of the series satisfying this model. If you know how to identify such segments before they end, then linear regression makes practical sense. And ANC only picks up the parameters of the chosen model - it does not guarantee the adequacy of the model itself. That's why it's not MNC's fault - it's the selection of an adequate model and its correct parameterisation that matters. And the model may be of any kind - stationary/non-stationary or sum of different distributions, for example. imha
See https://www.mql5.com/go?link=http://emm.ostu.ru/le ct/lect6_2.html[hash]vopros11 "Checking regression model adequacy" for details.
Fat tails may occur if the model chosen by the researcher is not adequate for the real series. The standard test of the adequacy of any regression model to real data is the distribution of residuals. Residuals are the deviation of real data from the model data. If the model is adequate, then the distribution of the residuals shall be normal. The ANC minimizes the sum of these deviations, but the distribution of the residuals will not necessarily be normal, although in some parts of the series it may be. Linear regression makes sense for SB model with drift or at some parts of the series satisfying this model. If you know how to identify such segments before they end, then linear regression makes practical sense. And ANC only picks up the parameters of the chosen model - it does not guarantee the adequacy of the model itself. That's why it's not MNC's fault - it's the selection of an adequate model and its correct parameterisation that matters. And the model may be of any kind - stationary/non-stationary or sum of different distributions, for example. imha
For details https://www.mql5.com/go?link=http://emm.ostu.ru/lect/lect6_2.html[hash]vopros11 "Checking regression model adequacy".
Did you write this for me? o)
That's what I was talking about the other day...
FreeLance 19.09.2010 15:52Well, get an empirical distribution of errors when approximated by a polynomial. And compare it with the normal one. Pay particular attention to the tails, not the central part.
Are we talking about choosing the best (in the sense of MNC) polynomial parameters?
Or about choosing them - but the best ones in another sense?
Or about the correctness of the polynomial for the approximation?
I asked for an explanation of the inefficiency of MNC to calculate parameters of a pre-selected function (after all, the reason for thick-tailedness may be in an unfortunate function :).
And if there are similarly simple procedures for determining these parameters - I'm happy to get acquainted with them.
But I'm surprised by the formulation of the question: since there are tails in errors, the MNC is no good...
;)
There's a different idea being pushed around here -
Such a target function - sum of error squares - is only optimal when the error distribution itself is normal.
;)
Did you write this to me? o)
That's what I was saying the other day...
FreeLance 19.09.2010 15:52I didn't write against the quoted one, I was even for it :)
I'm not against the quoted, I'm even for it :)
Thank you for your help in the discussion.
But Alexey's question/answer remains open.
Apply ISC only if you are sure that the future error distribution is normal?
As in - know the price...
;)
Apply ISC only if you are confident that the future error distribution is normal?
;)
It seems that yes (according to google :)), MNC is optimal if the measurement error is normally distributed. For other error distributions, there's least moduli method (errors are distributed by Laplace) and maximum likelihood method (in general, if error distribution is known). MNC is not always the best :)
However, in our case the error distribution is still unknown...
FreeLance:
Apply ANC only if you are sure that the future error distribution is normal?
Yes, and I agree with Alexey that MNC in the Gaussian case is equivalent to the maximum likelihood method. For other distributions it gives worse and even much worse results. I remember, when I was a student at the institute studying matstatistics, a standard phrase of teachers, which always puzzled me, was: "Since it's easier to do calculations this way (!!!!), we'll assume that the error distribution is Gaussian". Few people think that even the founders of these methods (Euler in particular) warned researchers about the danger of sacrificing the logic of reasoning for the sake of simplicity of calculations. As a result, the mathematical apparatus of alternative methods is poorly developed, and one has to do and invent everything half-heartedly by oneself. Good thing my parents sent me to study engineering :)))
j21,
Regarding the choice of polynomial, I personally don't see the point of going beyond the third or fourth degree.
It seems that yes (according to google :)), MOC is optimal if the measurement error is normally distributed. For other error distributions, there is least moduli method (errors are distributed by Laplace) and maximum likelihood method (in general, if error distribution is known). MNC is not always the best :)
True, in our case the error distribution is still unknown...
All this means is that we should approximate the "plausible" function, not whatever...
of the fourth degree.
;)
Let me explain by giving an illustration
P.S. Just in case, let me explain: this figure clearly shows what one strong ejection can do to the MNC. The ejection is, of course, quite prominent here, apparently for the sake of clarity.